From 7fe88613fa15d230d59482889c440c7befa17c25 Mon Sep 17 00:00:00 2001
From: Steven Liu <59462357+stevhliu@users.noreply.github.com>
Date: Tue, 21 Mar 2023 17:35:21 -0700
Subject: [PATCH] [docs] Clarify purpose of reproducibility docs (#2756)
* clarify purpose of repro docs
* apply feedback
---
docs/source/en/_toctree.yml | 4 +-
.../en/using-diffusers/reproducibility.mdx | 90 +++++++++----------
.../en/using-diffusers/reusing_seeds.mdx | 26 ++----
3 files changed, 51 insertions(+), 69 deletions(-)
diff --git a/docs/source/en/_toctree.yml b/docs/source/en/_toctree.yml
index f7bce58d..241377fe 100644
--- a/docs/source/en/_toctree.yml
+++ b/docs/source/en/_toctree.yml
@@ -43,9 +43,9 @@
- local: using-diffusers/depth2img
title: Text-Guided Depth-to-Image
- local: using-diffusers/reusing_seeds
- title: Reusing seeds for deterministic generation
+ title: Improve image quality with deterministic generation
- local: using-diffusers/reproducibility
- title: Reproducibility
+ title: Create reproducible pipelines
- local: using-diffusers/custom_pipeline_examples
title: Community Pipelines
- local: using-diffusers/contribute_pipeline
diff --git a/docs/source/en/using-diffusers/reproducibility.mdx b/docs/source/en/using-diffusers/reproducibility.mdx
index 0dd68b7c..35191c13 100644
--- a/docs/source/en/using-diffusers/reproducibility.mdx
+++ b/docs/source/en/using-diffusers/reproducibility.mdx
@@ -10,26 +10,26 @@ an "AS IS" BASIS, WITHOUT WARRANTIES OR CONDITIONS OF ANY KIND, either express o
specific language governing permissions and limitations under the License.
-->
-# Reproducibility
+# Create reproducible pipelines
-Before reading about reproducibility for Diffusers, it is strongly recommended to take a look at
-[PyTorch's statement about reproducibility](https://pytorch.org/docs/stable/notes/randomness.html).
+Reproducibility is important for testing, replicating results, and can even be used to [improve image quality](reusing_seeds). However, the randomness in diffusion models is a desired property because it allows the pipeline to generate different images every time it is run. While you can't expect to get the exact same results across platforms, you can expect results to be reproducible across releases and platforms within a certain tolerance range. Even then, tolerance varies depending on the diffusion pipeline and checkpoint.
-PyTorch states that
-> *completely reproducible results are not guaranteed across PyTorch releases, individual commits, or different platforms.*
-While one can never expect the same results across platforms, one can expect results to be reproducible
-across releases, platforms, etc... within a certain tolerance. However, this tolerance strongly varies
-depending on the diffusion pipeline and checkpoint.
+This is why it's important to understand how to control sources of randomness in diffusion models.
-In the following, we show how to best control sources of randomness for diffusion models.
+
+
+๐ก We strongly recommend reading PyTorch's [statement about reproducibility](https://pytorch.org/docs/stable/notes/randomness.html):
+
+> Completely reproducible results are not guaranteed across PyTorch releases, individual commits, or different platforms. Furthermore, results may not be reproducible between CPU and GPU executions, even when using identical seeds.
+
+
## Inference
-During inference, diffusion pipelines heavily rely on random sampling operations, such as the creating the
-gaussian noise tensors to be denoised and adding noise to the scheduling step.
+During inference, pipelines rely heavily on random sampling operations which include creating the
+Gaussian noise tensors to denoise and adding noise to the scheduling step.
-Let's have a look at an example. We run the [DDIM pipeline](./api/pipelines/ddim.mdx)
-for just two inference steps and return a numpy tensor to look into the numerical values of the output.
+Take a look at the tensor values in the [`DDIMPipeline`] after two inference steps:
```python
from diffusers import DDIMPipeline
@@ -45,11 +45,15 @@ image = ddim(num_inference_steps=2, output_type="np").images
print(np.abs(image).sum())
```
-Running the above prints a value of 1464.2076, but running it again prints a different
-value of 1495.1768. What is going on here? Every time the pipeline is run, gaussian noise
-is created and step-wise denoised. To create the gaussian noise with [`torch.randn`](https://pytorch.org/docs/stable/generated/torch.randn.html), a different random seed is taken every time, thus leading to a different result.
-This is a desired property of diffusion pipelines, as it means that the pipeline can create a different random image every time it is run. In many cases, one would like to generate the exact same image of a certain
-run, for which case an instance of a [PyTorch generator](https://pytorch.org/docs/stable/generated/torch.randn.html) has to be passed:
+Running the code above prints one value, but if you run it again you get a different value. What is going on here?
+
+Every time the pipeline is run, [`torch.randn`](https://pytorch.org/docs/stable/generated/torch.randn.html) uses a different random seed to create Gaussian noise which is denoised stepwise. This leads to a different result each time it is run, which is great for diffusion pipelines since it generates a different random image each time.
+
+But if you need to reliably generate the same image, that'll depend on whether you're running the pipeline on a CPU or GPU.
+
+### CPU
+
+To generate reproducible results on a CPU, you'll need to use a PyTorch [`Generator`](https://pytorch.org/docs/stable/generated/torch.randn.html) and set a seed:
```python
import torch
@@ -69,28 +73,22 @@ image = ddim(num_inference_steps=2, output_type="np", generator=generator).image
print(np.abs(image).sum())
```
-Running the above always prints a value of 1491.1711 - also upon running it again because we
-define the generator object to be passed to all random functions of the pipeline.
+Now when you run the code above, it always prints a value of `1491.1711` no matter what because the `Generator` object with the seed is passed to all the random functions of the pipeline.
-If you run this code snippet on your specific hardware and version, you should get a similar, if not the same, result.
+If you run this code example on your specific hardware and PyTorch version, you should get a similar, if not the same, result.
-It might be a bit unintuitive at first to pass `generator` objects to the pipelines instead of
+๐ก It might be a bit unintuitive at first to pass `Generator` objects to the pipeline instead of
just integer values representing the seed, but this is the recommended design when dealing with
-probabilistic models in PyTorch as generators are *random states* that are advanced and can thus be
+probabilistic models in PyTorch as `Generator`'s are *random states* that can be
passed to multiple pipelines in a sequence.
-Great! Now, we know how to write reproducible pipelines, but it gets a bit trickier since the above example only runs on the CPU. How do we also achieve reproducibility on GPU?
-In short, one should not expect full reproducibility across different hardware when running pipelines on GPU
-as matrix multiplications are less deterministic on GPU than on CPU and diffusion pipelines tend to require
-a lot of matrix multiplications. Let's see what we can do to keep the randomness within limits across
-different GPU hardware.
+### GPU
-To achieve maximum speed performance, it is recommended to create the generator directly on GPU when running
-the pipeline on GPU:
+Writing a reproducible pipeline on a GPU is a bit trickier, and full reproducibility across different hardware is not guaranteed because matrix multiplication - which diffusion pipelines require a lot of - is less deterministic on a GPU than a CPU. For example, if you run the same code example above on a GPU:
```python
import torch
@@ -111,12 +109,11 @@ image = ddim(num_inference_steps=2, output_type="np", generator=generator).image
print(np.abs(image).sum())
```
-Running the above now prints a value of 1389.8634 - even though we're using the exact same seed!
-This is unfortunate as it means we cannot reproduce the results we achieved on GPU, also on CPU.
-Nevertheless, it should be expected since the GPU uses a different random number generator than the CPU.
+The result is not the same even though you're using an identical seed because the GPU uses a different random number generator than the CPU.
-To circumvent this problem, we created a [`randn_tensor`](#diffusers.utils.randn_tensor) function, which can create random noise
-on the CPU and then move the tensor to GPU if necessary. The function is used everywhere inside the pipelines allowing the user to **always** pass a CPU generator even if the pipeline is run on GPU:
+To circumvent this problem, ๐งจ Diffusers has a [`randn_tensor`](#diffusers.utils.randn_tensor) function for creating random noise on the CPU, and then moving the tensor to a GPU if necessary. The `randn_tensor` function is used everywhere inside the pipeline, allowing the user to **always** pass a CPU `Generator` even if the pipeline is run on a GPU.
+
+You'll see the results are much closer now!
```python
import torch
@@ -129,7 +126,7 @@ model_id = "google/ddpm-cifar10-32"
ddim = DDIMPipeline.from_pretrained(model_id)
ddim.to("cuda")
-# create a generator for reproducibility
+# create a generator for reproducibility; notice you don't place it on the GPU!
generator = torch.manual_seed(0)
# run pipeline for just two steps and return numpy tensor
@@ -137,23 +134,18 @@ image = ddim(num_inference_steps=2, output_type="np", generator=generator).image
print(np.abs(image).sum())
```
-Running the above now prints a value of 1491.1713, much closer to the value of 1491.1711 when
-the pipeline is fully run on the CPU.
-
-As a consequence, we recommend always passing a CPU generator if Reproducibility is important.
-The loss of performance is often neglectable, but one can be sure to generate much more similar
-values than if the pipeline would have been run on CPU.
+๐ก If reproducibility is important, we recommend always passing a CPU generator.
+The performance loss is often neglectable, and you'll generate much more similar
+values than if the pipeline had been run on a GPU.
-Finally, we noticed that more complex pipelines, such as [`UnCLIPPipeline`] are often extremely
-susceptible to precision error propagation and thus one cannot expect even similar results across
-different GPU hardware or PyTorch versions. In such cases, one has to make sure to run
-exactly the same hardware and PyTorch version for full Reproducibility.
+Finally, for more complex pipelines such as [`UnCLIPPipeline`], these are often extremely
+susceptible to precision error propagation. Don't expect similar results across
+different GPU hardware or PyTorch versions. In this case, you'll need to run
+exactly the same hardware and PyTorch version for full reproducibility.
-## Randomness utilities
-
-### randn_tensor
+## randn_tensor
[[autodoc]] diffusers.utils.randn_tensor
diff --git a/docs/source/en/using-diffusers/reusing_seeds.mdx b/docs/source/en/using-diffusers/reusing_seeds.mdx
index d2b6b468..eea0fd7e 100644
--- a/docs/source/en/using-diffusers/reusing_seeds.mdx
+++ b/docs/source/en/using-diffusers/reusing_seeds.mdx
@@ -10,23 +10,17 @@ an "AS IS" BASIS, WITHOUT WARRANTIES OR CONDITIONS OF ANY KIND, either express o
specific language governing permissions and limitations under the License.
-->
-# Re-using seeds for fast prompt engineering
+# Improve image quality with deterministic generation
-A common use case when generating images is to generate a batch of images, select one image and improve it with a better, more detailed prompt in a second run.
-To do this, one needs to make each generated image of the batch deterministic.
-Images are generated by denoising gaussian random noise which can be instantiated by passing a [torch generator](https://pytorch.org/docs/stable/generated/torch.Generator.html#generator).
+A common way to improve the quality of generated images is with *deterministic batch generation*, generate a batch of images and select one image to improve with a more detailed prompt in a second round of inference. The key is to pass a list of [`torch.Generator`](https://pytorch.org/docs/stable/generated/torch.Generator.html#generator)'s to the pipeline for batched image generation, and tie each `Generator` to a seed so you can reuse it for an image.
-Now, for batched generation, we need to make sure that every single generated image in the batch is tied exactly to one seed. In ๐งจ Diffusers, this can be achieved by not passing one `generator`, but a list
-of `generators` to the pipeline.
-
-Let's go through an example using [`runwayml/stable-diffusion-v1-5`](runwayml/stable-diffusion-v1-5).
-We want to generate several versions of the prompt:
+Let's use [`runwayml/stable-diffusion-v1-5`](runwayml/stable-diffusion-v1-5) for example, and generate several versions of the following prompt:
```py
prompt = "Labrador in the style of Vermeer"
```
-Let's load the pipeline
+Instantiate a pipeline with [`DiffusionPipeline.from_pretrained`] and place it on a GPU (if available):
```python
>>> from diffusers import DiffusionPipeline
@@ -35,7 +29,7 @@ Let's load the pipeline
>>> pipe = pipe.to("cuda")
```
-Now, let's define 4 different generators, since we would like to reproduce a certain image. We'll use seeds `0` to `3` to create our generators.
+Now, define four different `Generator`'s and assign each `Generator` a seed (`0` to `3`) so you can reuse a `Generator` later for a specific image:
```python
>>> import torch
@@ -43,7 +37,7 @@ Now, let's define 4 different generators, since we would like to reproduce a cer
>>> generator = [torch.Generator(device="cuda").manual_seed(i) for i in range(4)]
```
-Let's generate 4 images:
+Generate the images and have a look:
```python
>>> images = pipe(prompt, generator=generator, num_images_per_prompt=4).images
@@ -52,18 +46,14 @@ Let's generate 4 images:
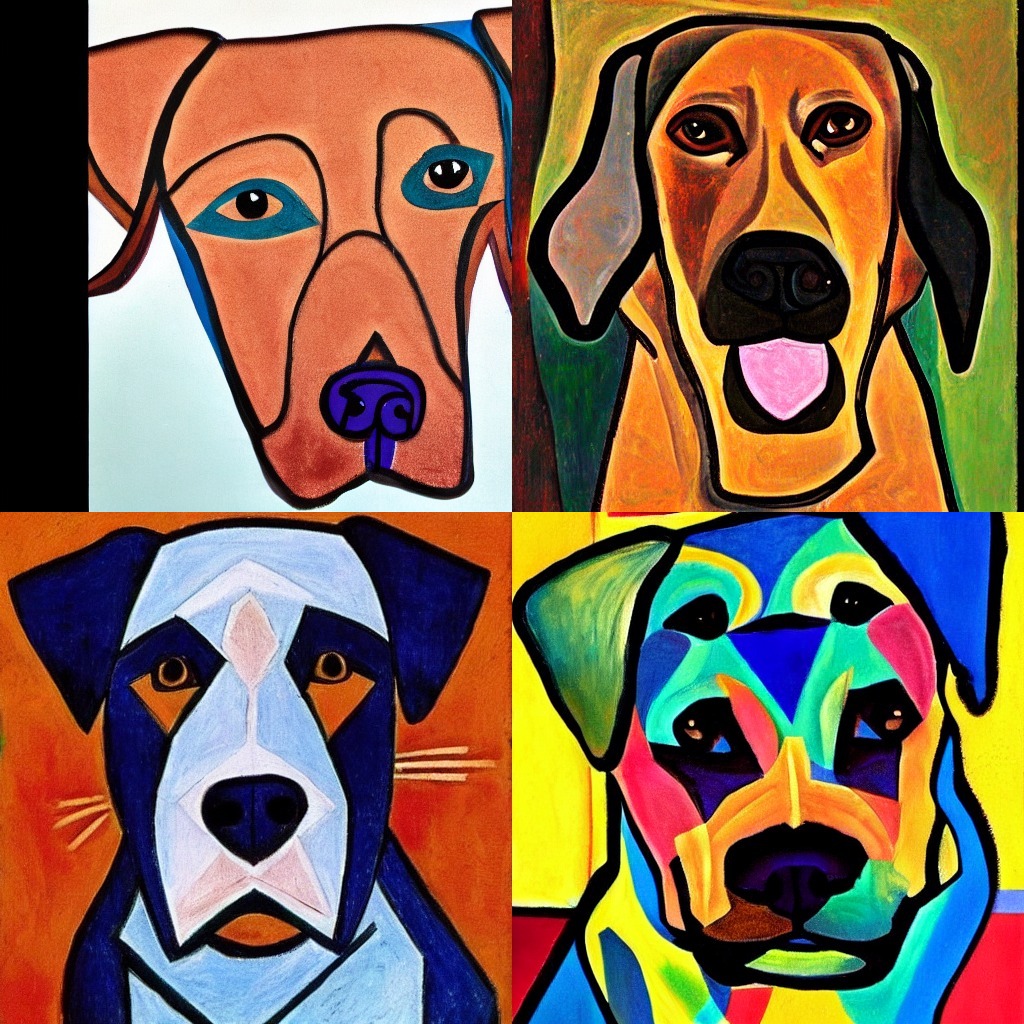
-Ok, the last images has some double eyes, but the first image looks good!
-Let's try to make the prompt a bit better **while keeping the first seed**
-so that the images are similar to the first image.
+In this example, you'll improve upon the first image - but in reality, you can use any image you want (even the image with double sets of eyes!). The first image used the `Generator` with seed `0`, so you'll reuse that `Generator` for the second round of inference. To improve the quality of the image, add some additional text to the prompt:
```python
prompt = [prompt + t for t in [", highly realistic", ", artsy", ", trending", ", colorful"]]
generator = [torch.Generator(device="cuda").manual_seed(0) for i in range(4)]
```
-We create 4 generators with seed `0`, which is the first seed we used before.
-
-Let's run the pipeline again.
+Create four generators with seed `0`, and generate another batch of images, all of which should look like the first image from the previous round!
```python
>>> images = pipe(prompt, generator=generator).images