Merge branch 'main' into feat/page_re_alloc
This commit is contained in:
commit
fe6a2756f1
|
@ -51,16 +51,19 @@ jobs:
|
|||
steps:
|
||||
- name: Checkout repository
|
||||
uses: actions/checkout@v3
|
||||
- name: Initialize Docker Buildx
|
||||
uses: docker/setup-buildx-action@v2.0.0
|
||||
with:
|
||||
install: true
|
||||
|
||||
- name: Inject slug/short variables
|
||||
uses: rlespinasse/github-slug-action@v4.4.1
|
||||
- name: Tailscale
|
||||
uses: huggingface/tailscale-action@main
|
||||
with:
|
||||
authkey: ${{ secrets.TAILSCALE_AUTHKEY }}
|
||||
slackChannel: ${{ secrets.SLACK_CIFEEDBACK_CHANNEL }}
|
||||
slackToken: ${{ secrets.SLACK_CIFEEDBACK_BOT_TOKEN }}
|
||||
- name: Initialize Docker Buildx
|
||||
uses: docker/setup-buildx-action@v2.0.0
|
||||
with:
|
||||
install: true
|
||||
- name: Login to GitHub Container Registry
|
||||
if: github.event_name != 'pull_request'
|
||||
uses: docker/login-action@v2
|
||||
|
@ -121,6 +124,7 @@ jobs:
|
|||
DOCKER_LABEL=sha-${{ env.GITHUB_SHA_SHORT }}${{ matrix.label }}
|
||||
tags: ${{ steps.meta.outputs.tags || steps.meta-pr.outputs.tags }}
|
||||
labels: ${{ steps.meta.outputs.labels || steps.meta-pr.outputs.labels }}
|
||||
network: host
|
||||
cache-from: type=registry,ref=registry.internal.huggingface.tech/api-inference/community/text-generation-inference:cache${{ matrix.label }},mode=min
|
||||
cache-to: type=registry,ref=registry.internal.huggingface.tech/api-inference/community/text-generation-inference:cache${{ matrix.label }},mode=min
|
||||
- name: Set up Python
|
||||
|
@ -139,3 +143,8 @@ jobs:
|
|||
export DOCKER_IMAGE=registry.internal.huggingface.tech/api-inference/community/text-generation-inference:sha-${{ env.GITHUB_SHA_SHORT }}
|
||||
export HUGGING_FACE_HUB_TOKEN=${{ secrets.HUGGING_FACE_HUB_TOKEN }}
|
||||
pytest -s -vv integration-tests
|
||||
- name: Tailscale Wait
|
||||
if: ${{ failure() || runner.debug == '1' }}
|
||||
uses: huggingface/tailscale-action@main
|
||||
with:
|
||||
waitForSSH: true
|
||||
|
|
|
@ -33,9 +33,9 @@ jobs:
|
|||
- name: Install Rust
|
||||
uses: actions-rs/toolchain@v1
|
||||
with:
|
||||
# Released on: 02 May, 2024
|
||||
# https://releases.rs/docs/1.78.0/
|
||||
toolchain: 1.78.0
|
||||
# Released on: June 13, 2024
|
||||
# https://releases.rs/docs/1.79.0/
|
||||
toolchain: 1.79.0
|
||||
override: true
|
||||
components: rustfmt, clippy
|
||||
- name: Install Protoc
|
||||
|
|
|
@ -0,0 +1,133 @@
|
|||
|
||||
# Contributor Covenant Code of Conduct
|
||||
|
||||
## Our Pledge
|
||||
|
||||
We as members, contributors, and leaders pledge to make participation in our
|
||||
community a harassment-free experience for everyone, regardless of age, body
|
||||
size, visible or invisible disability, ethnicity, sex characteristics, gender
|
||||
identity and expression, level of experience, education, socio-economic status,
|
||||
nationality, personal appearance, race, caste, color, religion, or sexual
|
||||
identity and orientation.
|
||||
|
||||
We pledge to act and interact in ways that contribute to an open, welcoming,
|
||||
diverse, inclusive, and healthy community.
|
||||
|
||||
## Our Standards
|
||||
|
||||
Examples of behavior that contributes to a positive environment for our
|
||||
community include:
|
||||
|
||||
* Demonstrating empathy and kindness toward other people
|
||||
* Being respectful of differing opinions, viewpoints, and experiences
|
||||
* Giving and gracefully accepting constructive feedback
|
||||
* Accepting responsibility and apologizing to those affected by our mistakes,
|
||||
and learning from the experience
|
||||
* Focusing on what is best not just for us as individuals, but for the overall
|
||||
community
|
||||
|
||||
Examples of unacceptable behavior include:
|
||||
|
||||
* The use of sexualized language or imagery, and sexual attention or advances of
|
||||
any kind
|
||||
* Trolling, insulting or derogatory comments, and personal or political attacks
|
||||
* Public or private harassment
|
||||
* Publishing others' private information, such as a physical or email address,
|
||||
without their explicit permission
|
||||
* Other conduct which could reasonably be considered inappropriate in a
|
||||
professional setting
|
||||
|
||||
## Enforcement Responsibilities
|
||||
|
||||
Community leaders are responsible for clarifying and enforcing our standards of
|
||||
acceptable behavior and will take appropriate and fair corrective action in
|
||||
response to any behavior that they deem inappropriate, threatening, offensive,
|
||||
or harmful.
|
||||
|
||||
Community leaders have the right and responsibility to remove, edit, or reject
|
||||
comments, commits, code, wiki edits, issues, and other contributions that are
|
||||
not aligned to this Code of Conduct, and will communicate reasons for moderation
|
||||
decisions when appropriate.
|
||||
|
||||
## Scope
|
||||
|
||||
This Code of Conduct applies within all community spaces, and also applies when
|
||||
an individual is officially representing the community in public spaces.
|
||||
Examples of representing our community include using an official e-mail address,
|
||||
posting via an official social media account, or acting as an appointed
|
||||
representative at an online or offline event.
|
||||
|
||||
## Enforcement
|
||||
|
||||
Instances of abusive, harassing, or otherwise unacceptable behavior may be
|
||||
reported to the community leaders responsible for enforcement at
|
||||
feedback@huggingface.co.
|
||||
All complaints will be reviewed and investigated promptly and fairly.
|
||||
|
||||
All community leaders are obligated to respect the privacy and security of the
|
||||
reporter of any incident.
|
||||
|
||||
## Enforcement Guidelines
|
||||
|
||||
Community leaders will follow these Community Impact Guidelines in determining
|
||||
the consequences for any action they deem in violation of this Code of Conduct:
|
||||
|
||||
### 1. Correction
|
||||
|
||||
**Community Impact**: Use of inappropriate language or other behavior deemed
|
||||
unprofessional or unwelcome in the community.
|
||||
|
||||
**Consequence**: A private, written warning from community leaders, providing
|
||||
clarity around the nature of the violation and an explanation of why the
|
||||
behavior was inappropriate. A public apology may be requested.
|
||||
|
||||
### 2. Warning
|
||||
|
||||
**Community Impact**: A violation through a single incident or series of
|
||||
actions.
|
||||
|
||||
**Consequence**: A warning with consequences for continued behavior. No
|
||||
interaction with the people involved, including unsolicited interaction with
|
||||
those enforcing the Code of Conduct, for a specified period of time. This
|
||||
includes avoiding interactions in community spaces as well as external channels
|
||||
like social media. Violating these terms may lead to a temporary or permanent
|
||||
ban.
|
||||
|
||||
### 3. Temporary Ban
|
||||
|
||||
**Community Impact**: A serious violation of community standards, including
|
||||
sustained inappropriate behavior.
|
||||
|
||||
**Consequence**: A temporary ban from any sort of interaction or public
|
||||
communication with the community for a specified period of time. No public or
|
||||
private interaction with the people involved, including unsolicited interaction
|
||||
with those enforcing the Code of Conduct, is allowed during this period.
|
||||
Violating these terms may lead to a permanent ban.
|
||||
|
||||
### 4. Permanent Ban
|
||||
|
||||
**Community Impact**: Demonstrating a pattern of violation of community
|
||||
standards, including sustained inappropriate behavior, harassment of an
|
||||
individual, or aggression toward or disparagement of classes of individuals.
|
||||
|
||||
**Consequence**: A permanent ban from any sort of public interaction within the
|
||||
community.
|
||||
|
||||
## Attribution
|
||||
|
||||
This Code of Conduct is adapted from the [Contributor Covenant][homepage],
|
||||
version 2.1, available at
|
||||
[https://www.contributor-covenant.org/version/2/1/code_of_conduct.html][v2.1].
|
||||
|
||||
Community Impact Guidelines were inspired by
|
||||
[Mozilla's code of conduct enforcement ladder][Mozilla CoC].
|
||||
|
||||
For answers to common questions about this code of conduct, see the FAQ at
|
||||
[https://www.contributor-covenant.org/faq][FAQ]. Translations are available at
|
||||
[https://www.contributor-covenant.org/translations][translations].
|
||||
|
||||
[homepage]: https://www.contributor-covenant.org
|
||||
[v2.1]: https://www.contributor-covenant.org/version/2/1/code_of_conduct.html
|
||||
[Mozilla CoC]: https://github.com/mozilla/diversity
|
||||
[FAQ]: https://www.contributor-covenant.org/faq
|
||||
[translations]: https://www.contributor-covenant.org/translations
|
|
@ -0,0 +1,120 @@
|
|||
<!---
|
||||
Copyright 2024 The HuggingFace Team. All rights reserved.
|
||||
|
||||
Licensed under the Apache License, Version 2.0 (the "License");
|
||||
you may not use this file except in compliance with the License.
|
||||
You may obtain a copy of the License at
|
||||
|
||||
http://www.apache.org/licenses/LICENSE-2.0
|
||||
|
||||
Unless required by applicable law or agreed to in writing, software
|
||||
distributed under the License is distributed on an "AS IS" BASIS,
|
||||
WITHOUT WARRANTIES OR CONDITIONS OF ANY KIND, either express or implied.
|
||||
See the License for the specific language governing permissions and
|
||||
limitations under the License.
|
||||
-->
|
||||
|
||||
# Contribute to text-generation-inference
|
||||
|
||||
Everyone is welcome to contribute, and we value everybody's contribution. Code
|
||||
contributions are not the only way to help the community. Answering questions, helping
|
||||
others, and improving the documentation are also immensely valuable.
|
||||
|
||||
It also helps us if you spread the word! Reference the library in blog posts
|
||||
about the awesome projects it made possible, shout out on Twitter every time it has
|
||||
helped you, or simply ⭐️ the repository to say thank you.
|
||||
|
||||
However you choose to contribute, please be mindful and respect our
|
||||
[code of conduct](https://github.com/huggingface/text-generation-inference/blob/main/CODE_OF_CONDUCT.md).
|
||||
|
||||
**This guide was heavily inspired by the awesome [scikit-learn guide to contributing](https://github.com/scikit-learn/scikit-learn/blob/main/CONTRIBUTING.md).**
|
||||
|
||||
## Ways to contribute
|
||||
|
||||
There are several ways you can contribute to text-generation-inference.
|
||||
|
||||
* Fix outstanding issues with the existing code.
|
||||
* Submit issues related to bugs or desired new features.
|
||||
* Contribute to the examples or to the documentation.
|
||||
|
||||
> All contributions are equally valuable to the community. 🥰
|
||||
|
||||
## Fixing outstanding issues
|
||||
|
||||
If you notice an issue with the existing code and have a fix in mind, feel free to [start contributing](https://docs.github.com/en/pull-requests/collaborating-with-pull-requests/proposing-changes-to-your-work-with-pull-requests/creating-a-pull-request) and open
|
||||
a Pull Request!
|
||||
|
||||
## Submitting a bug-related issue or feature request
|
||||
|
||||
Do your best to follow these guidelines when submitting a bug-related issue or a feature
|
||||
request. It will make it easier for us to come back to you quickly and with good
|
||||
feedback.
|
||||
|
||||
### Did you find a bug?
|
||||
|
||||
The text-generation-inference library is robust and reliable thanks to users who report the problems they encounter.
|
||||
|
||||
Before you report an issue, we would really appreciate it if you could **make sure the bug was not
|
||||
already reported** (use the search bar on GitHub under Issues). Your issue should also be related to bugs in the
|
||||
library itself, and not your code.
|
||||
|
||||
Once you've confirmed the bug hasn't already been reported, please include the following information in your issue so
|
||||
we can quickly resolve it:
|
||||
|
||||
* Your **OS type and version**, as well as your environment versions (versions of rust, python, and dependencies).
|
||||
* A short, self-contained, code snippet that allows us to reproduce the bug.
|
||||
* The *full* traceback if an exception is raised.
|
||||
* Attach any other additional information, like screenshots, you think may help.
|
||||
|
||||
To get the OS and software versions automatically, you can re-run the launcher with the `--env` flag:
|
||||
|
||||
```bash
|
||||
text-generation-launcher --env
|
||||
```
|
||||
|
||||
This will precede the launch of the model with the information relative to your environment. We recommend pasting
|
||||
that in your issue report.
|
||||
|
||||
### Do you want a new feature?
|
||||
|
||||
If there is a new feature you'd like to see in text-generation-inference, please open an issue and describe:
|
||||
|
||||
1. What is the *motivation* behind this feature? Is it related to a problem or frustration with the library? Is it
|
||||
a feature related to something you need for a project? Is it something you worked on and think it could benefit
|
||||
the community?
|
||||
|
||||
Whatever it is, we'd love to hear about it!
|
||||
|
||||
2. Describe your requested feature in as much detail as possible. The more you can tell us about it, the better
|
||||
we'll be able to help you.
|
||||
3. Provide a *code snippet* that demonstrates the feature's usage.
|
||||
4. If the feature is related to a paper, please include a link.
|
||||
|
||||
If your issue is well written we're already 80% of the way there by the time you create it.
|
||||
|
||||
We have added [templates](https://github.com/huggingface/text-generation-inference/tree/main/.github/ISSUE_TEMPLATE)
|
||||
to help you get started with your issue.
|
||||
|
||||
## Do you want to implement a new model?
|
||||
|
||||
New models are constantly released and if you want to implement a new model, please provide the following information:
|
||||
|
||||
* A short description of the model and a link to the paper.
|
||||
* Link to the implementation if it is open-sourced.
|
||||
* Link to the model weights if they are available.
|
||||
|
||||
If you are willing to contribute the model yourself, let us know so we can help you add it to text-generation-inference!
|
||||
|
||||
## Do you want to add documentation?
|
||||
|
||||
We're always looking for improvements to the documentation that make it more clear and accurate. Please let us know
|
||||
how the documentation can be improved such as typos and any content that is missing, unclear or inaccurate. We'll be
|
||||
happy to make the changes or help you make a contribution if you're interested!
|
||||
|
||||
## I want to become a maintainer of the project. How do I get there?
|
||||
|
||||
TGI is a project led and managed by Hugging Face as it powers our internal services. However, we are happy to have
|
||||
motivated individuals from other organizations join us as maintainers with the goal of making TGI the best inference
|
||||
service.
|
||||
|
||||
If you are such an individual (or organization), please reach out to us and let's collaborate.
|
|
@ -1856,12 +1856,23 @@ dependencies = [
|
|||
|
||||
[[package]]
|
||||
name = "minijinja"
|
||||
version = "1.0.12"
|
||||
source = "git+https://github.com/mitsuhiko/minijinja.git?rev=5cd4efb#5cd4efb9e2639247df275fe6e22a5dbe0ce71b28"
|
||||
version = "2.0.2"
|
||||
source = "registry+https://github.com/rust-lang/crates.io-index"
|
||||
checksum = "e136ef580d7955019ab0a407b68d77c292a9976907e217900f3f76bc8f6dc1a4"
|
||||
dependencies = [
|
||||
"serde",
|
||||
]
|
||||
|
||||
[[package]]
|
||||
name = "minijinja-contrib"
|
||||
version = "2.0.2"
|
||||
source = "registry+https://github.com/rust-lang/crates.io-index"
|
||||
checksum = "15ee37078c98d31e510d6a7af488031a2c3ccacdb76c5c4fc98ddfe6d0e9da07"
|
||||
dependencies = [
|
||||
"minijinja",
|
||||
"serde",
|
||||
]
|
||||
|
||||
[[package]]
|
||||
name = "minimal-lexical"
|
||||
version = "0.2.1"
|
||||
|
@ -3604,6 +3615,7 @@ dependencies = [
|
|||
"metrics",
|
||||
"metrics-exporter-prometheus",
|
||||
"minijinja",
|
||||
"minijinja-contrib",
|
||||
"ngrok",
|
||||
"nohash-hasher",
|
||||
"once_cell",
|
||||
|
|
|
@ -1,5 +1,5 @@
|
|||
# Rust builder
|
||||
FROM lukemathwalker/cargo-chef:latest-rust-1.78 AS chef
|
||||
FROM lukemathwalker/cargo-chef:latest-rust-1.79 AS chef
|
||||
WORKDIR /usr/src
|
||||
|
||||
ARG CARGO_REGISTRIES_CRATES_IO_PROTOCOL=sparse
|
||||
|
@ -140,9 +140,9 @@ RUN TORCH_CUDA_ARCH_LIST="8.0;8.6+PTX" make build-eetq
|
|||
# Build marlin kernels
|
||||
FROM kernel-builder as marlin-kernels-builder
|
||||
WORKDIR /usr/src
|
||||
COPY server/Makefile-marlin Makefile
|
||||
COPY server/marlin/ .
|
||||
# Build specific version of transformers
|
||||
RUN TORCH_CUDA_ARCH_LIST="8.0;8.6+PTX" make build-marlin
|
||||
RUN TORCH_CUDA_ARCH_LIST="8.0;8.6+PTX" python setup.py build
|
||||
|
||||
# Build Transformers CUDA kernels
|
||||
FROM kernel-builder as custom-kernels-builder
|
||||
|
@ -213,7 +213,7 @@ COPY --from=awq-kernels-builder /usr/src/llm-awq/awq/kernels/build/lib.linux-x86
|
|||
# Copy build artifacts from eetq kernels builder
|
||||
COPY --from=eetq-kernels-builder /usr/src/eetq/build/lib.linux-x86_64-cpython-310 /opt/conda/lib/python3.10/site-packages
|
||||
# Copy build artifacts from marlin kernels builder
|
||||
COPY --from=marlin-kernels-builder /usr/src/marlin/build/lib.linux-x86_64-cpython-310 /opt/conda/lib/python3.10/site-packages
|
||||
COPY --from=marlin-kernels-builder /usr/src/build/lib.linux-x86_64-cpython-310 /opt/conda/lib/python3.10/site-packages
|
||||
|
||||
# Copy builds artifacts from vllm builder
|
||||
COPY --from=vllm-builder /usr/src/vllm/build/lib.linux-x86_64-cpython-310 /opt/conda/lib/python3.10/site-packages
|
||||
|
|
|
@ -1,5 +1,5 @@
|
|||
# Rust builder
|
||||
FROM lukemathwalker/cargo-chef:latest-rust-1.78 AS chef
|
||||
FROM lukemathwalker/cargo-chef:latest-rust-1.79 AS chef
|
||||
WORKDIR /usr/src
|
||||
|
||||
ARG CARGO_REGISTRIES_CRATES_IO_PROTOCOL=sparse
|
||||
|
|
|
@ -1,4 +1,4 @@
|
|||
FROM lukemathwalker/cargo-chef:latest-rust-1.78 AS chef
|
||||
FROM lukemathwalker/cargo-chef:latest-rust-1.79 AS chef
|
||||
WORKDIR /usr/src
|
||||
|
||||
ARG CARGO_REGISTRIES_CRATES_IO_PROTOCOL=sparse
|
||||
|
|
|
@ -17,6 +17,8 @@
|
|||
title: Supported Models and Hardware
|
||||
- local: messages_api
|
||||
title: Messages API
|
||||
- local: architecture
|
||||
title: Internal Architecture
|
||||
title: Getting started
|
||||
- sections:
|
||||
- local: basic_tutorials/consuming_tgi
|
||||
|
|
|
@ -0,0 +1,227 @@
|
|||
# Text Generation Inference Architecture
|
||||
|
||||
This document aims at describing the architecture of Text Generation Inference (TGI), by describing the call flow between the separate components.
|
||||
|
||||
A high-level architecture diagram can be seen here:
|
||||
|
||||
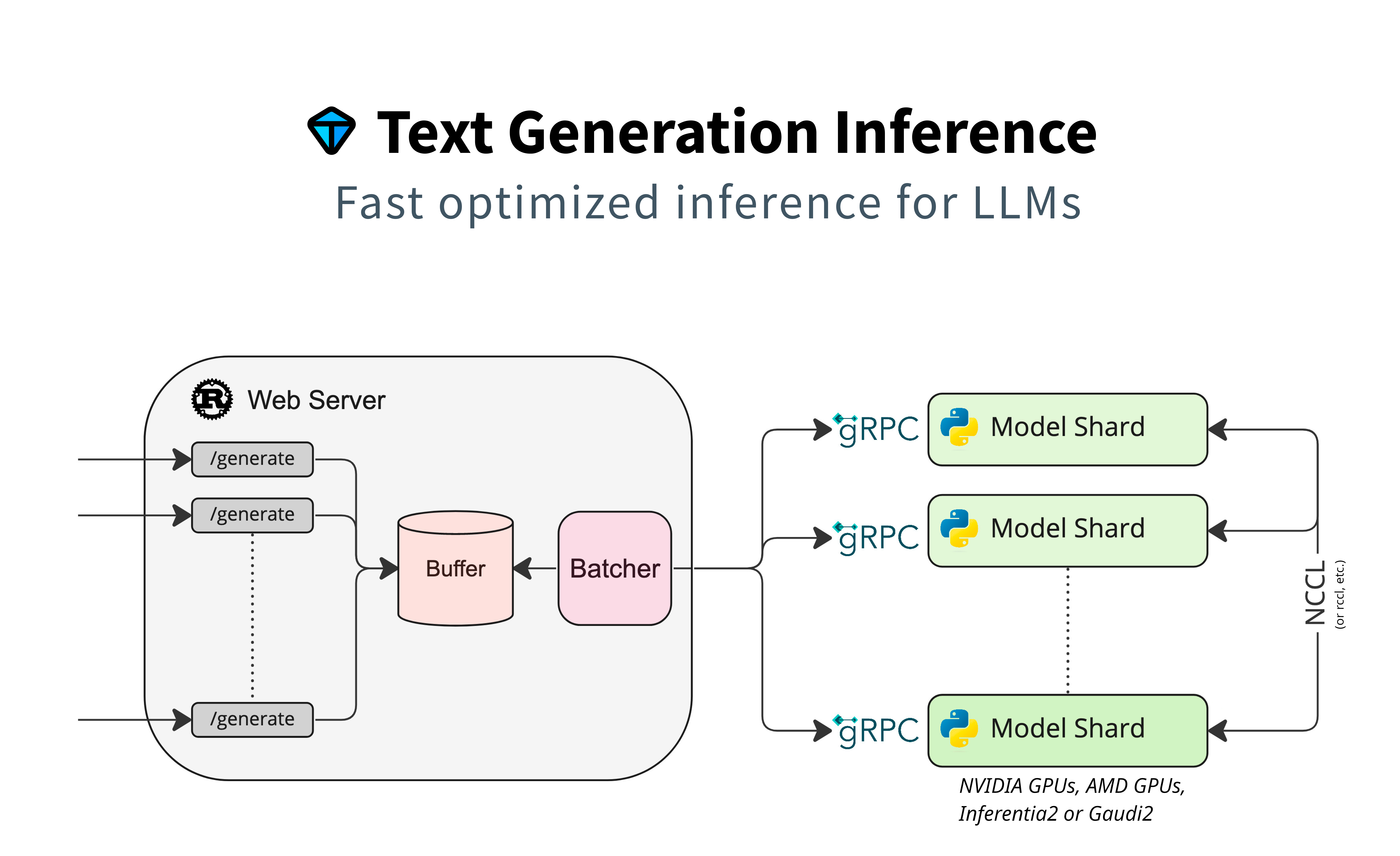
|
||||
|
||||
This diagram shows well there are these separate components:
|
||||
|
||||
- **The router**, also named `webserver`, that receives the client requests, buffers them, creates some batches, and prepares gRPC calls to a model server.
|
||||
- **The model server**, responsible of receiving the gRPC requests and to process the inference on the model. If the model is sharded across multiple accelerators (e.g.: multiple GPUs), the model server shards might be synchronized via NCCL or equivalent.
|
||||
- **The launcher** is a helper thar will be able to launch one or several model servers (if model is sharded), and it launches the router with the compatible arguments.
|
||||
|
||||
The router and the model server can be two different machines, they do not need to be deployed together.
|
||||
|
||||
## The Router
|
||||
|
||||
This component is a rust web server binary that accepts HTTP requests using the custom [HTTP API](https://huggingface.github.io/text-generation-inference/), as well as OpenAI's [Messages API](https://huggingface.co/docs/text-generation-inference/messages_api).
|
||||
The router receives the API calls and handles the "baches" logic (and introduction to batching can be found [here](https://github.com/huggingface/text-generation-inference/blob/main/router/README.md)).
|
||||
It uses different strategies to reduce latency between requests and responses, especially oriented to decoding latency. It will use queues, schedulers, and block allocators to achieve that and produce batched requests that it will then be sent to the model server.
|
||||
|
||||
### Router's command line
|
||||
|
||||
The router command line will be the way to pass parameters to it (it does not rely on configuration file):
|
||||
|
||||
```
|
||||
Text Generation Webserver
|
||||
|
||||
Usage: text-generation-router [OPTIONS]
|
||||
|
||||
Options:
|
||||
--max-concurrent-requests <MAX_CONCURRENT_REQUESTS>
|
||||
[env: MAX_CONCURRENT_REQUESTS=] [default: 128]
|
||||
--max-best-of <MAX_BEST_OF>
|
||||
[env: MAX_BEST_OF=] [default: 2]
|
||||
--max-stop-sequences <MAX_STOP_SEQUENCES>
|
||||
[env: MAX_STOP_SEQUENCES=] [default: 4]
|
||||
--max-top-n-tokens <MAX_TOP_N_TOKENS>
|
||||
[env: MAX_TOP_N_TOKENS=] [default: 5]
|
||||
--max-input-tokens <MAX_INPUT_TOKENS>
|
||||
[env: MAX_INPUT_TOKENS=] [default: 1024]
|
||||
--max-total-tokens <MAX_TOTAL_TOKENS>
|
||||
[env: MAX_TOTAL_TOKENS=] [default: 2048]
|
||||
--waiting-served-ratio <WAITING_SERVED_RATIO>
|
||||
[env: WAITING_SERVED_RATIO=] [default: 1.2]
|
||||
--max-batch-prefill-tokens <MAX_BATCH_PREFILL_TOKENS>
|
||||
[env: MAX_BATCH_PREFILL_TOKENS=] [default: 4096]
|
||||
--max-batch-total-tokens <MAX_BATCH_TOTAL_TOKENS>
|
||||
[env: MAX_BATCH_TOTAL_TOKENS=]
|
||||
--max-waiting-tokens <MAX_WAITING_TOKENS>
|
||||
[env: MAX_WAITING_TOKENS=] [default: 20]
|
||||
--max-batch-size <MAX_BATCH_SIZE>
|
||||
[env: MAX_BATCH_SIZE=]
|
||||
--hostname <HOSTNAME>
|
||||
[env: HOSTNAME=] [default: 0.0.0.0]
|
||||
-p, --port <PORT>
|
||||
[env: PORT=] [default: 3000]
|
||||
--master-shard-uds-path <MASTER_SHARD_UDS_PATH>
|
||||
[env: MASTER_SHARD_UDS_PATH=] [default: /tmp/text-generation-server-0]
|
||||
--tokenizer-name <TOKENIZER_NAME>
|
||||
[env: TOKENIZER_NAME=] [default: bigscience/bloom]
|
||||
--tokenizer-config-path <TOKENIZER_CONFIG_PATH>
|
||||
[env: TOKENIZER_CONFIG_PATH=]
|
||||
--revision <REVISION>
|
||||
[env: REVISION=]
|
||||
--validation-workers <VALIDATION_WORKERS>
|
||||
[env: VALIDATION_WORKERS=] [default: 2]
|
||||
--json-output
|
||||
[env: JSON_OUTPUT=]
|
||||
--otlp-endpoint <OTLP_ENDPOINT>
|
||||
[env: OTLP_ENDPOINT=]
|
||||
--cors-allow-origin <CORS_ALLOW_ORIGIN>
|
||||
[env: CORS_ALLOW_ORIGIN=]
|
||||
--ngrok
|
||||
[env: NGROK=]
|
||||
--ngrok-authtoken <NGROK_AUTHTOKEN>
|
||||
[env: NGROK_AUTHTOKEN=]
|
||||
--ngrok-edge <NGROK_EDGE>
|
||||
[env: NGROK_EDGE=]
|
||||
--messages-api-enabled
|
||||
[env: MESSAGES_API_ENABLED=]
|
||||
--disable-grammar-support
|
||||
[env: DISABLE_GRAMMAR_SUPPORT=]
|
||||
--max-client-batch-size <MAX_CLIENT_BATCH_SIZE>
|
||||
[env: MAX_CLIENT_BATCH_SIZE=] [default: 4]
|
||||
-h, --help
|
||||
Print help
|
||||
-V, --version
|
||||
Print version
|
||||
```
|
||||
|
||||
## The Model Server
|
||||
|
||||
The model server is a python server, capable of starting a server waiting for gRPC requests, loads a given model, perform sharding to provide [tensor parallelism](https://huggingface.co/docs/text-generation-inference/conceptual/tensor_parallelism), and stays alive while waiting for new requests.
|
||||
The model server supports models instantiated using Pytorch and optimized for inference mainly on CUDA/ROCM.
|
||||
|
||||
### Model Server Variants
|
||||
|
||||
Several variants of the model server exist that are actively supported by Hugging Face:
|
||||
|
||||
- By default, the model server will attempt building [a server optimized for Nvidia GPUs with CUDA](https://huggingface.co/docs/text-generation-inference/installation_nvidia). The code for this version is hosted in the [main TGI repository](https://github.com/huggingface/text-generation-inference).
|
||||
- A [version optimized for AMD with ROCm](https://huggingface.co/docs/text-generation-inference/installation_amd) is hosted in the main TGI repository. Some model features differ.
|
||||
- The [version for Intel Gaudi](https://huggingface.co/docs/text-generation-inference/installation_gaudi) is maintained on a forked repository, often resynchronized with the main [TGI repository](https://github.com/huggingface/tgi-gaudi).
|
||||
- A [version for Neuron (AWS Inferentia2)](https://huggingface.co/docs/text-generation-inference/installation_inferentia) is maintained as part of [Optimum Neuron](https://github.com/huggingface/optimum-neuron/tree/main/text-generation-inference).
|
||||
- A version for Google TPUs is maintained as part of [Optimum TPU](https://github.com/huggingface/optimum-tpu/tree/main/text-generation-inference).
|
||||
|
||||
Not all variants provide the same features, as hardware and middleware capabilities do not provide the same optimizations.
|
||||
|
||||
### Command Line Interface
|
||||
|
||||
The official command line interface (CLI) for the server supports three subcommands, `download-weights`, `quantize` and `serve`:
|
||||
|
||||
- `download-weights` will download weights from the hub and, in some variants it will convert weights to a format that is adapted to the given implementation;
|
||||
- `quantize` will allow to quantize a model using the `qptq` package. This feature is not available nor supported on all variants;
|
||||
- `serve` will start the server that load a model (or a model shard), receives gRPC calls from the router, performs an inference and provides a formatted response to the given request.
|
||||
|
||||
Serve's command line parameters on the TGI repository are these:
|
||||
|
||||
```
|
||||
Usage: cli.py serve [OPTIONS] MODEL_ID
|
||||
|
||||
╭─ Arguments ──────────────────────────────────────────────────────────────────────────────────────────────╮
|
||||
│ * model_id TEXT [default: None] [required] │
|
||||
╰──────────────────────────────────────────────────────────────────────────────────────────────────────────╯
|
||||
╭─ Options ────────────────────────────────────────────────────────────────────────────────────────────────╮
|
||||
│ --revision TEXT [default: None] │
|
||||
│ --sharded --no-sharded [default: no-sharded] │
|
||||
│ --quantize [bitsandbytes|bitsandbytes [default: None] │
|
||||
│ -nf4|bitsandbytes-fp4|gptq │
|
||||
│ |awq|eetq|exl2|fp8] │
|
||||
│ --speculate INTEGER [default: None] │
|
||||
│ --dtype [float16|bfloat16] [default: None] │
|
||||
│ --trust-remote-code --no-trust-remote-code [default: │
|
||||
│ no-trust-remote-code] │
|
||||
│ --uds-path PATH [default: │
|
||||
│ /tmp/text-generation-serve… │
|
||||
│ --logger-level TEXT [default: INFO] │
|
||||
│ --json-output --no-json-output [default: no-json-output] │
|
||||
│ --otlp-endpoint TEXT [default: None] │
|
||||
│ --help Show this message and exit. │
|
||||
╰──────────────────────────────────────────────────────────────────────────────────────────────────────────╯
|
||||
```
|
||||
|
||||
Note that some variants might support different parameters, and they could possibly accept more options that can be passed on using environment variables.
|
||||
|
||||
## Call Flow
|
||||
|
||||
Once both components are initialized, weights downloaded and model server is up and running, router and model server exchange data and info through the gRPC call. There are currently two supported schemas, [v2](https://github.com/huggingface/text-generation-inference/blob/main/proto/generate.proto) and [v3](https://github.com/huggingface/text-generation-inference/blob/main/proto/v3/generate.proto). These two versions are almost identical, except for:
|
||||
|
||||
- input chunks support, for text and image data,
|
||||
- paged attention support
|
||||
|
||||
Here's a diagram that displays the exchanges that follow the router and model server startup.
|
||||
|
||||
```mermaid
|
||||
sequenceDiagram
|
||||
|
||||
Router->>Model Server: service discovery
|
||||
Model Server-->>Router: urls for other shards
|
||||
|
||||
Router->>Model Server: get model info
|
||||
Model Server-->>Router: shard info
|
||||
|
||||
Router->>Model Server: health check
|
||||
Model Server-->>Router: health OK
|
||||
|
||||
Router->>Model Server: warmup(max_input_tokens, max_batch_prefill_tokens, max_total_tokens, max_batch_size)
|
||||
Model Server-->>Router: warmup result
|
||||
```
|
||||
|
||||
After these are done, the router is ready to receive generate calls from multiple clients. Here's an example.
|
||||
|
||||
```mermaid
|
||||
sequenceDiagram
|
||||
participant Client 1
|
||||
participant Client 2
|
||||
participant Client 3
|
||||
participant Router
|
||||
participant Model Server
|
||||
|
||||
Client 1->>Router: generate_stream
|
||||
Router->>Model Server: prefill(batch1)
|
||||
Model Server-->>Router: generations, cached_batch1, timings
|
||||
Router-->>Client 1: token 1
|
||||
|
||||
Router->>Model Server: decode(cached_batch1)
|
||||
Model Server-->>Router: generations, cached_batch1, timings
|
||||
Router-->>Client 1: token 2
|
||||
|
||||
Router->>Model Server: decode(cached_batch1)
|
||||
Model Server-->>Router: generations, cached_batch1, timings
|
||||
Router-->>Client 1: token 3
|
||||
|
||||
Client 2->>Router: generate_stream
|
||||
Router->>Model Server: prefill(batch2)
|
||||
Note right of Model Server: This stops previous batch, that is restarted
|
||||
Model Server-->>Router: generations, cached_batch2, timings
|
||||
Router-->>Client 2: token 1'
|
||||
|
||||
Router->>Model Server: decode(cached_batch1, cached_batch2)
|
||||
Model Server-->>Router: generations, cached_batch1, timings
|
||||
Router-->>Client 1: token 4
|
||||
Router-->>Client 2: token 2'
|
||||
|
||||
Note left of Client 1: Client 1 leaves
|
||||
Router->>Model Server: filter_batch(cached_batch1, request_ids_to_keep=batch2)
|
||||
Model Server-->>Router: filtered batch
|
||||
|
||||
Router->>Model Server: decode(cached_batch2)
|
||||
Model Server-->>Router: generations, cached_batch2, timings
|
||||
Router-->>Client 2: token 3'
|
||||
|
||||
Client 3->>Router: generate_stream
|
||||
Note right of Model Server: This stops previous batch, that is restarted
|
||||
Router->>Model Server: prefill(batch3)
|
||||
Note left of Client 1: Client 3 leaves without receiving any batch
|
||||
Router->>Model Server: clear_cache(batch3)
|
||||
Note right of Model Server: This stops previous batch, that is restarted
|
||||
|
||||
Router->>Model Server: decode(cached_batch3)
|
||||
Note right of Model Server: Last token (stopping criteria)
|
||||
Model Server-->>Router: generations, cached_batch3, timings
|
||||
Router-->>Client 2: token 4'
|
||||
|
||||
|
||||
```
|
|
@ -20,7 +20,7 @@ Text Generation Inference enables serving optimized models on specific hardware
|
|||
- [Baichuan](https://huggingface.co/baichuan-inc/Baichuan2-7B-Chat)
|
||||
- [Falcon](https://huggingface.co/tiiuae/falcon-7b-instruct)
|
||||
- [StarCoder 2](https://huggingface.co/bigcode/starcoder2-15b-instruct-v0.1)
|
||||
- [Qwen 2](https://huggingface.co/bigcode/starcoder2-15b-instruct-v0.1)
|
||||
- [Qwen 2](https://huggingface.co/collections/Qwen/qwen2-6659360b33528ced941e557f)
|
||||
- [Opt](https://huggingface.co/facebook/opt-6.7b)
|
||||
- [T5](https://huggingface.co/google/flan-t5-xxl)
|
||||
- [Galactica](https://huggingface.co/facebook/galactica-120b)
|
||||
|
|
|
@ -0,0 +1,84 @@
|
|||
{
|
||||
"details": {
|
||||
"best_of_sequences": null,
|
||||
"finish_reason": "length",
|
||||
"generated_tokens": 10,
|
||||
"prefill": [
|
||||
{
|
||||
"id": 2323,
|
||||
"logprob": null,
|
||||
"text": "Test"
|
||||
},
|
||||
{
|
||||
"id": 1715,
|
||||
"logprob": -11.34375,
|
||||
"text": " request"
|
||||
}
|
||||
],
|
||||
"seed": null,
|
||||
"tokens": [
|
||||
{
|
||||
"id": 198,
|
||||
"logprob": -2.5742188,
|
||||
"special": false,
|
||||
"text": "\n"
|
||||
},
|
||||
{
|
||||
"id": 262,
|
||||
"logprob": -1.6230469,
|
||||
"special": false,
|
||||
"text": " "
|
||||
},
|
||||
{
|
||||
"id": 3270,
|
||||
"logprob": -2.046875,
|
||||
"special": false,
|
||||
"text": " \"\"\"\n"
|
||||
},
|
||||
{
|
||||
"id": 262,
|
||||
"logprob": -0.015281677,
|
||||
"special": false,
|
||||
"text": " "
|
||||
},
|
||||
{
|
||||
"id": 422,
|
||||
"logprob": -2.1425781,
|
||||
"special": false,
|
||||
"text": " if"
|
||||
},
|
||||
{
|
||||
"id": 1715,
|
||||
"logprob": -0.9238281,
|
||||
"special": false,
|
||||
"text": " request"
|
||||
},
|
||||
{
|
||||
"id": 13204,
|
||||
"logprob": -0.076660156,
|
||||
"special": false,
|
||||
"text": ".method"
|
||||
},
|
||||
{
|
||||
"id": 624,
|
||||
"logprob": -0.021987915,
|
||||
"special": false,
|
||||
"text": " =="
|
||||
},
|
||||
{
|
||||
"id": 364,
|
||||
"logprob": -0.39208984,
|
||||
"special": false,
|
||||
"text": " '"
|
||||
},
|
||||
{
|
||||
"id": 3019,
|
||||
"logprob": -0.10821533,
|
||||
"special": false,
|
||||
"text": "POST"
|
||||
}
|
||||
],
|
||||
"top_tokens": null
|
||||
},
|
||||
"generated_text": "\n \"\"\"\n if request.method == 'POST"
|
||||
}
|
|
@ -0,0 +1,84 @@
|
|||
{
|
||||
"details": {
|
||||
"best_of_sequences": null,
|
||||
"finish_reason": "length",
|
||||
"generated_tokens": 10,
|
||||
"prefill": [
|
||||
{
|
||||
"id": 2323,
|
||||
"logprob": null,
|
||||
"text": "Test"
|
||||
},
|
||||
{
|
||||
"id": 1715,
|
||||
"logprob": -11.34375,
|
||||
"text": " request"
|
||||
}
|
||||
],
|
||||
"seed": 0,
|
||||
"tokens": [
|
||||
{
|
||||
"id": 13,
|
||||
"logprob": -2.2539062,
|
||||
"special": false,
|
||||
"text": "."
|
||||
},
|
||||
{
|
||||
"id": 578,
|
||||
"logprob": -0.15563965,
|
||||
"special": false,
|
||||
"text": " The"
|
||||
},
|
||||
{
|
||||
"id": 3622,
|
||||
"logprob": -0.8203125,
|
||||
"special": false,
|
||||
"text": " server"
|
||||
},
|
||||
{
|
||||
"id": 706,
|
||||
"logprob": 0.0,
|
||||
"special": false,
|
||||
"text": " has"
|
||||
},
|
||||
{
|
||||
"id": 539,
|
||||
"logprob": 0.0,
|
||||
"special": false,
|
||||
"text": " not"
|
||||
},
|
||||
{
|
||||
"id": 3686,
|
||||
"logprob": 0.0,
|
||||
"special": false,
|
||||
"text": " yet"
|
||||
},
|
||||
{
|
||||
"id": 3288,
|
||||
"logprob": 0.0,
|
||||
"special": false,
|
||||
"text": " sent"
|
||||
},
|
||||
{
|
||||
"id": 904,
|
||||
"logprob": 0.0,
|
||||
"special": false,
|
||||
"text": " any"
|
||||
},
|
||||
{
|
||||
"id": 828,
|
||||
"logprob": 0.0,
|
||||
"special": false,
|
||||
"text": " data"
|
||||
},
|
||||
{
|
||||
"id": 382,
|
||||
"logprob": -1.5517578,
|
||||
"special": false,
|
||||
"text": ".\n\n"
|
||||
}
|
||||
],
|
||||
"top_tokens": null
|
||||
},
|
||||
"generated_text": "Test request. The server has not yet sent any data.\n\n"
|
||||
}
|
|
@ -0,0 +1,338 @@
|
|||
[
|
||||
{
|
||||
"details": {
|
||||
"best_of_sequences": null,
|
||||
"finish_reason": "length",
|
||||
"generated_tokens": 10,
|
||||
"prefill": [
|
||||
{
|
||||
"id": 2323,
|
||||
"logprob": null,
|
||||
"text": "Test"
|
||||
},
|
||||
{
|
||||
"id": 1715,
|
||||
"logprob": -11.34375,
|
||||
"text": " request"
|
||||
}
|
||||
],
|
||||
"seed": null,
|
||||
"tokens": [
|
||||
{
|
||||
"id": 198,
|
||||
"logprob": -2.5742188,
|
||||
"special": false,
|
||||
"text": "\n"
|
||||
},
|
||||
{
|
||||
"id": 262,
|
||||
"logprob": -1.6220703,
|
||||
"special": false,
|
||||
"text": " "
|
||||
},
|
||||
{
|
||||
"id": 3270,
|
||||
"logprob": -2.0410156,
|
||||
"special": false,
|
||||
"text": " \"\"\"\n"
|
||||
},
|
||||
{
|
||||
"id": 262,
|
||||
"logprob": -0.015281677,
|
||||
"special": false,
|
||||
"text": " "
|
||||
},
|
||||
{
|
||||
"id": 422,
|
||||
"logprob": -2.1445312,
|
||||
"special": false,
|
||||
"text": " if"
|
||||
},
|
||||
{
|
||||
"id": 1715,
|
||||
"logprob": -0.92333984,
|
||||
"special": false,
|
||||
"text": " request"
|
||||
},
|
||||
{
|
||||
"id": 13204,
|
||||
"logprob": -0.07672119,
|
||||
"special": false,
|
||||
"text": ".method"
|
||||
},
|
||||
{
|
||||
"id": 624,
|
||||
"logprob": -0.021987915,
|
||||
"special": false,
|
||||
"text": " =="
|
||||
},
|
||||
{
|
||||
"id": 364,
|
||||
"logprob": -0.39208984,
|
||||
"special": false,
|
||||
"text": " '"
|
||||
},
|
||||
{
|
||||
"id": 3019,
|
||||
"logprob": -0.10638428,
|
||||
"special": false,
|
||||
"text": "POST"
|
||||
}
|
||||
],
|
||||
"top_tokens": null
|
||||
},
|
||||
"generated_text": "\n \"\"\"\n if request.method == 'POST"
|
||||
},
|
||||
{
|
||||
"details": {
|
||||
"best_of_sequences": null,
|
||||
"finish_reason": "length",
|
||||
"generated_tokens": 10,
|
||||
"prefill": [
|
||||
{
|
||||
"id": 2323,
|
||||
"logprob": null,
|
||||
"text": "Test"
|
||||
},
|
||||
{
|
||||
"id": 1715,
|
||||
"logprob": -11.34375,
|
||||
"text": " request"
|
||||
}
|
||||
],
|
||||
"seed": null,
|
||||
"tokens": [
|
||||
{
|
||||
"id": 198,
|
||||
"logprob": -2.5742188,
|
||||
"special": false,
|
||||
"text": "\n"
|
||||
},
|
||||
{
|
||||
"id": 262,
|
||||
"logprob": -1.6220703,
|
||||
"special": false,
|
||||
"text": " "
|
||||
},
|
||||
{
|
||||
"id": 3270,
|
||||
"logprob": -2.0410156,
|
||||
"special": false,
|
||||
"text": " \"\"\"\n"
|
||||
},
|
||||
{
|
||||
"id": 262,
|
||||
"logprob": -0.015281677,
|
||||
"special": false,
|
||||
"text": " "
|
||||
},
|
||||
{
|
||||
"id": 422,
|
||||
"logprob": -2.1445312,
|
||||
"special": false,
|
||||
"text": " if"
|
||||
},
|
||||
{
|
||||
"id": 1715,
|
||||
"logprob": -0.92333984,
|
||||
"special": false,
|
||||
"text": " request"
|
||||
},
|
||||
{
|
||||
"id": 13204,
|
||||
"logprob": -0.07672119,
|
||||
"special": false,
|
||||
"text": ".method"
|
||||
},
|
||||
{
|
||||
"id": 624,
|
||||
"logprob": -0.021987915,
|
||||
"special": false,
|
||||
"text": " =="
|
||||
},
|
||||
{
|
||||
"id": 364,
|
||||
"logprob": -0.39208984,
|
||||
"special": false,
|
||||
"text": " '"
|
||||
},
|
||||
{
|
||||
"id": 3019,
|
||||
"logprob": -0.10638428,
|
||||
"special": false,
|
||||
"text": "POST"
|
||||
}
|
||||
],
|
||||
"top_tokens": null
|
||||
},
|
||||
"generated_text": "\n \"\"\"\n if request.method == 'POST"
|
||||
},
|
||||
{
|
||||
"details": {
|
||||
"best_of_sequences": null,
|
||||
"finish_reason": "length",
|
||||
"generated_tokens": 10,
|
||||
"prefill": [
|
||||
{
|
||||
"id": 2323,
|
||||
"logprob": null,
|
||||
"text": "Test"
|
||||
},
|
||||
{
|
||||
"id": 1715,
|
||||
"logprob": -11.34375,
|
||||
"text": " request"
|
||||
}
|
||||
],
|
||||
"seed": null,
|
||||
"tokens": [
|
||||
{
|
||||
"id": 198,
|
||||
"logprob": -2.5742188,
|
||||
"special": false,
|
||||
"text": "\n"
|
||||
},
|
||||
{
|
||||
"id": 262,
|
||||
"logprob": -1.6220703,
|
||||
"special": false,
|
||||
"text": " "
|
||||
},
|
||||
{
|
||||
"id": 3270,
|
||||
"logprob": -2.0410156,
|
||||
"special": false,
|
||||
"text": " \"\"\"\n"
|
||||
},
|
||||
{
|
||||
"id": 262,
|
||||
"logprob": -0.015281677,
|
||||
"special": false,
|
||||
"text": " "
|
||||
},
|
||||
{
|
||||
"id": 422,
|
||||
"logprob": -2.1445312,
|
||||
"special": false,
|
||||
"text": " if"
|
||||
},
|
||||
{
|
||||
"id": 1715,
|
||||
"logprob": -0.92333984,
|
||||
"special": false,
|
||||
"text": " request"
|
||||
},
|
||||
{
|
||||
"id": 13204,
|
||||
"logprob": -0.07672119,
|
||||
"special": false,
|
||||
"text": ".method"
|
||||
},
|
||||
{
|
||||
"id": 624,
|
||||
"logprob": -0.021987915,
|
||||
"special": false,
|
||||
"text": " =="
|
||||
},
|
||||
{
|
||||
"id": 364,
|
||||
"logprob": -0.39208984,
|
||||
"special": false,
|
||||
"text": " '"
|
||||
},
|
||||
{
|
||||
"id": 3019,
|
||||
"logprob": -0.10638428,
|
||||
"special": false,
|
||||
"text": "POST"
|
||||
}
|
||||
],
|
||||
"top_tokens": null
|
||||
},
|
||||
"generated_text": "\n \"\"\"\n if request.method == 'POST"
|
||||
},
|
||||
{
|
||||
"details": {
|
||||
"best_of_sequences": null,
|
||||
"finish_reason": "length",
|
||||
"generated_tokens": 10,
|
||||
"prefill": [
|
||||
{
|
||||
"id": 2323,
|
||||
"logprob": null,
|
||||
"text": "Test"
|
||||
},
|
||||
{
|
||||
"id": 1715,
|
||||
"logprob": -11.34375,
|
||||
"text": " request"
|
||||
}
|
||||
],
|
||||
"seed": null,
|
||||
"tokens": [
|
||||
{
|
||||
"id": 198,
|
||||
"logprob": -2.5742188,
|
||||
"special": false,
|
||||
"text": "\n"
|
||||
},
|
||||
{
|
||||
"id": 262,
|
||||
"logprob": -1.6220703,
|
||||
"special": false,
|
||||
"text": " "
|
||||
},
|
||||
{
|
||||
"id": 3270,
|
||||
"logprob": -2.0410156,
|
||||
"special": false,
|
||||
"text": " \"\"\"\n"
|
||||
},
|
||||
{
|
||||
"id": 262,
|
||||
"logprob": -0.015281677,
|
||||
"special": false,
|
||||
"text": " "
|
||||
},
|
||||
{
|
||||
"id": 422,
|
||||
"logprob": -2.1445312,
|
||||
"special": false,
|
||||
"text": " if"
|
||||
},
|
||||
{
|
||||
"id": 1715,
|
||||
"logprob": -0.92333984,
|
||||
"special": false,
|
||||
"text": " request"
|
||||
},
|
||||
{
|
||||
"id": 13204,
|
||||
"logprob": -0.07672119,
|
||||
"special": false,
|
||||
"text": ".method"
|
||||
},
|
||||
{
|
||||
"id": 624,
|
||||
"logprob": -0.021987915,
|
||||
"special": false,
|
||||
"text": " =="
|
||||
},
|
||||
{
|
||||
"id": 364,
|
||||
"logprob": -0.39208984,
|
||||
"special": false,
|
||||
"text": " '"
|
||||
},
|
||||
{
|
||||
"id": 3019,
|
||||
"logprob": -0.10638428,
|
||||
"special": false,
|
||||
"text": "POST"
|
||||
}
|
||||
],
|
||||
"top_tokens": null
|
||||
},
|
||||
"generated_text": "\n \"\"\"\n if request.method == 'POST"
|
||||
}
|
||||
]
|
|
@ -0,0 +1,61 @@
|
|||
{
|
||||
"details": {
|
||||
"best_of_sequences": null,
|
||||
"finish_reason": "eos_token",
|
||||
"generated_tokens": 8,
|
||||
"prefill": [],
|
||||
"seed": null,
|
||||
"tokens": [
|
||||
{
|
||||
"id": 2502,
|
||||
"logprob": -1.734375,
|
||||
"special": false,
|
||||
"text": "image"
|
||||
},
|
||||
{
|
||||
"id": 2196,
|
||||
"logprob": -0.5756836,
|
||||
"special": false,
|
||||
"text": " result"
|
||||
},
|
||||
{
|
||||
"id": 604,
|
||||
"logprob": -0.007843018,
|
||||
"special": false,
|
||||
"text": " for"
|
||||
},
|
||||
{
|
||||
"id": 12254,
|
||||
"logprob": -1.7167969,
|
||||
"special": false,
|
||||
"text": " chicken"
|
||||
},
|
||||
{
|
||||
"id": 611,
|
||||
"logprob": -0.17053223,
|
||||
"special": false,
|
||||
"text": " on"
|
||||
},
|
||||
{
|
||||
"id": 573,
|
||||
"logprob": -0.7626953,
|
||||
"special": false,
|
||||
"text": " the"
|
||||
},
|
||||
{
|
||||
"id": 8318,
|
||||
"logprob": -0.02709961,
|
||||
"special": false,
|
||||
"text": " beach"
|
||||
},
|
||||
{
|
||||
"id": 1,
|
||||
"logprob": -0.20739746,
|
||||
"special": true,
|
||||
"text": "<eos>"
|
||||
}
|
||||
],
|
||||
"top_tokens": null
|
||||
},
|
||||
"generated_text": "image result for chicken on the beach"
|
||||
}
|
|
@ -0,0 +1,23 @@
|
|||
{
|
||||
"choices": [
|
||||
{
|
||||
"finish_reason": "eos_token",
|
||||
"index": 0,
|
||||
"logprobs": null,
|
||||
"message": {
|
||||
"content": "{\n \"temperature\": [\n 35,\n 34,\n 36\n ],\n \"unit\": \"°c\"\n}",
|
||||
"role": "assistant"
|
||||
}
|
||||
}
|
||||
],
|
||||
"created": 1718044128,
|
||||
"id": "",
|
||||
"model": "TinyLlama/TinyLlama-1.1B-Chat-v1.0",
|
||||
"object": "text_completion",
|
||||
"system_fingerprint": "2.0.5-dev0-native",
|
||||
"usage": {
|
||||
"completion_tokens": 39,
|
||||
"prompt_tokens": 136,
|
||||
"total_tokens": 175
|
||||
}
|
||||
}
|
|
@ -0,0 +1,85 @@
|
|||
{
|
||||
"details": {
|
||||
"best_of_sequences": null,
|
||||
"finish_reason": "eos_token",
|
||||
"generated_tokens": 12,
|
||||
"prefill": [],
|
||||
"seed": null,
|
||||
"tokens": [
|
||||
{
|
||||
"id": 450,
|
||||
"logprob": -0.26342773,
|
||||
"special": false,
|
||||
"text": " The"
|
||||
},
|
||||
{
|
||||
"id": 21282,
|
||||
"logprob": -0.01838684,
|
||||
"special": false,
|
||||
"text": " cow"
|
||||
},
|
||||
{
|
||||
"id": 322,
|
||||
"logprob": -0.18041992,
|
||||
"special": false,
|
||||
"text": " and"
|
||||
},
|
||||
{
|
||||
"id": 521,
|
||||
"logprob": -0.62841797,
|
||||
"special": false,
|
||||
"text": " ch"
|
||||
},
|
||||
{
|
||||
"id": 21475,
|
||||
"logprob": -0.0037956238,
|
||||
"special": false,
|
||||
"text": "icken"
|
||||
},
|
||||
{
|
||||
"id": 526,
|
||||
"logprob": -0.018737793,
|
||||
"special": false,
|
||||
"text": " are"
|
||||
},
|
||||
{
|
||||
"id": 373,
|
||||
"logprob": -1.0820312,
|
||||
"special": false,
|
||||
"text": " on"
|
||||
},
|
||||
{
|
||||
"id": 263,
|
||||
"logprob": -0.5083008,
|
||||
"special": false,
|
||||
"text": " a"
|
||||
},
|
||||
{
|
||||
"id": 25695,
|
||||
"logprob": -0.07128906,
|
||||
"special": false,
|
||||
"text": " beach"
|
||||
},
|
||||
{
|
||||
"id": 29889,
|
||||
"logprob": -0.12573242,
|
||||
"special": false,
|
||||
"text": "."
|
||||
},
|
||||
{
|
||||
"id": 32002,
|
||||
"logprob": -0.0029792786,
|
||||
"special": true,
|
||||
"text": "<end_of_utterance>"
|
||||
},
|
||||
{
|
||||
"id": 2,
|
||||
"logprob": -0.00024962425,
|
||||
"special": true,
|
||||
"text": "</s>"
|
||||
}
|
||||
],
|
||||
"top_tokens": null
|
||||
},
|
||||
"generated_text": " The cow and chicken are on a beach."
|
||||
}
|
|
@ -0,0 +1,133 @@
|
|||
{
|
||||
"details": {
|
||||
"best_of_sequences": null,
|
||||
"finish_reason": "length",
|
||||
"generated_tokens": 20,
|
||||
"prefill": [],
|
||||
"seed": null,
|
||||
"tokens": [
|
||||
{
|
||||
"id": 415,
|
||||
"logprob": -0.04421997,
|
||||
"special": false,
|
||||
"text": " The"
|
||||
},
|
||||
{
|
||||
"id": 12072,
|
||||
"logprob": -0.13500977,
|
||||
"special": false,
|
||||
"text": " cow"
|
||||
},
|
||||
{
|
||||
"id": 349,
|
||||
"logprob": -0.06750488,
|
||||
"special": false,
|
||||
"text": " is"
|
||||
},
|
||||
{
|
||||
"id": 6328,
|
||||
"logprob": -0.6352539,
|
||||
"special": false,
|
||||
"text": " standing"
|
||||
},
|
||||
{
|
||||
"id": 356,
|
||||
"logprob": -0.16186523,
|
||||
"special": false,
|
||||
"text": " on"
|
||||
},
|
||||
{
|
||||
"id": 272,
|
||||
"logprob": -0.5078125,
|
||||
"special": false,
|
||||
"text": " the"
|
||||
},
|
||||
{
|
||||
"id": 10305,
|
||||
"logprob": -0.017913818,
|
||||
"special": false,
|
||||
"text": " beach"
|
||||
},
|
||||
{
|
||||
"id": 304,
|
||||
"logprob": -1.5205078,
|
||||
"special": false,
|
||||
"text": " and"
|
||||
},
|
||||
{
|
||||
"id": 272,
|
||||
"logprob": -0.029174805,
|
||||
"special": false,
|
||||
"text": " the"
|
||||
},
|
||||
{
|
||||
"id": 13088,
|
||||
"logprob": -0.003479004,
|
||||
"special": false,
|
||||
"text": " chicken"
|
||||
},
|
||||
{
|
||||
"id": 349,
|
||||
"logprob": -0.0035095215,
|
||||
"special": false,
|
||||
"text": " is"
|
||||
},
|
||||
{
|
||||
"id": 6398,
|
||||
"logprob": -0.3088379,
|
||||
"special": false,
|
||||
"text": " sitting"
|
||||
},
|
||||
{
|
||||
"id": 356,
|
||||
"logprob": -0.027755737,
|
||||
"special": false,
|
||||
"text": " on"
|
||||
},
|
||||
{
|
||||
"id": 264,
|
||||
"logprob": -0.31884766,
|
||||
"special": false,
|
||||
"text": " a"
|
||||
},
|
||||
{
|
||||
"id": 17972,
|
||||
"logprob": -0.047943115,
|
||||
"special": false,
|
||||
"text": " pile"
|
||||
},
|
||||
{
|
||||
"id": 302,
|
||||
"logprob": -0.0002925396,
|
||||
"special": false,
|
||||
"text": " of"
|
||||
},
|
||||
{
|
||||
"id": 2445,
|
||||
"logprob": -0.02935791,
|
||||
"special": false,
|
||||
"text": " money"
|
||||
},
|
||||
{
|
||||
"id": 28723,
|
||||
"logprob": -0.031219482,
|
||||
"special": false,
|
||||
"text": "."
|
||||
},
|
||||
{
|
||||
"id": 32002,
|
||||
"logprob": -0.00034475327,
|
||||
"special": true,
|
||||
"text": "<end_of_utterance>"
|
||||
},
|
||||
{
|
||||
"id": 2,
|
||||
"logprob": -1.1920929e-07,
|
||||
"special": true,
|
||||
"text": "</s>"
|
||||
}
|
||||
],
|
||||
"top_tokens": null
|
||||
},
|
||||
"generated_text": " The cow is standing on the beach and the chicken is sitting on a pile of money."
|
||||
}
|
|
@ -0,0 +1,65 @@
|
|||
import pytest
|
||||
|
||||
|
||||
@pytest.fixture(scope="module")
|
||||
def flash_llama_gptq_marlin_handle(launcher):
|
||||
with launcher(
|
||||
"astronomer/Llama-3-8B-Instruct-GPTQ-4-Bit", num_shard=2, quantize="marlin"
|
||||
) as handle:
|
||||
yield handle
|
||||
|
||||
|
||||
@pytest.fixture(scope="module")
|
||||
async def flash_llama_gptq_marlin(flash_llama_gptq_marlin_handle):
|
||||
await flash_llama_gptq_marlin_handle.health(300)
|
||||
return flash_llama_gptq_marlin_handle.client
|
||||
|
||||
|
||||
@pytest.mark.asyncio
|
||||
@pytest.mark.private
|
||||
async def test_flash_llama_gptq_marlin(flash_llama_gptq_marlin, response_snapshot):
|
||||
response = await flash_llama_gptq_marlin.generate(
|
||||
"Test request", max_new_tokens=10, decoder_input_details=True
|
||||
)
|
||||
|
||||
assert response.details.generated_tokens == 10
|
||||
assert response == response_snapshot
|
||||
|
||||
|
||||
@pytest.mark.asyncio
|
||||
@pytest.mark.private
|
||||
async def test_flash_llama_gptq_marlin_all_params(
|
||||
flash_llama_gptq_marlin, response_snapshot
|
||||
):
|
||||
response = await flash_llama_gptq_marlin.generate(
|
||||
"Test request",
|
||||
max_new_tokens=10,
|
||||
repetition_penalty=1.2,
|
||||
return_full_text=True,
|
||||
temperature=0.5,
|
||||
top_p=0.9,
|
||||
top_k=10,
|
||||
truncate=5,
|
||||
typical_p=0.9,
|
||||
watermark=True,
|
||||
decoder_input_details=True,
|
||||
seed=0,
|
||||
)
|
||||
|
||||
assert response.details.generated_tokens == 10
|
||||
assert response == response_snapshot
|
||||
|
||||
|
||||
@pytest.mark.asyncio
|
||||
@pytest.mark.private
|
||||
async def test_flash_llama_gptq_marlin_load(
|
||||
flash_llama_gptq_marlin, generate_load, response_snapshot
|
||||
):
|
||||
responses = await generate_load(
|
||||
flash_llama_gptq_marlin, "Test request", max_new_tokens=10, n=4
|
||||
)
|
||||
|
||||
assert len(responses) == 4
|
||||
assert all([r.generated_text == responses[0].generated_text for r in responses])
|
||||
|
||||
assert responses == response_snapshot
|
|
@ -22,6 +22,12 @@ async def flash_pali_gemma(flash_pali_gemma_handle):
|
|||
return flash_pali_gemma_handle.client
|
||||
|
||||
|
||||
def get_chicken():
|
||||
with open("integration-tests/images/chicken_on_money.png", "rb") as image_file:
|
||||
encoded_string = base64.b64encode(image_file.read())
|
||||
return f"data:image/png;base64,{encoded_string.decode('utf-8')}"
|
||||
|
||||
|
||||
def get_cow_beach():
|
||||
with open("integration-tests/images/cow_beach.png", "rb") as image_file:
|
||||
encoded_string = base64.b64encode(image_file.read())
|
||||
|
@ -37,3 +43,20 @@ async def test_flash_pali_gemma(flash_pali_gemma, response_snapshot):
|
|||
|
||||
assert response.generated_text == "beach"
|
||||
assert response == response_snapshot
|
||||
|
||||
|
||||
@pytest.mark.asyncio
|
||||
@pytest.mark.private
|
||||
async def test_flash_pali_gemma_two_images(flash_pali_gemma, response_snapshot):
|
||||
chicken = get_chicken()
|
||||
cow_beach = get_cow_beach()
|
||||
response = await flash_pali_gemma.generate(
|
||||
f"caption\n",
|
||||
max_new_tokens=20,
|
||||
)
|
||||
# Is PaliGemma not able to handle two separate images? At least we
|
||||
# get output showing that both images are used.
|
||||
assert (
|
||||
response.generated_text == "image result for chicken on the beach"
|
||||
), f"{repr(response.generated_text)}"
|
||||
assert response == response_snapshot
|
||||
|
|
|
@ -0,0 +1,101 @@
|
|||
import pytest
|
||||
import requests
|
||||
from pydantic import BaseModel
|
||||
from typing import List
|
||||
|
||||
|
||||
@pytest.fixture(scope="module")
|
||||
def llama_grammar_handle(launcher):
|
||||
with launcher(
|
||||
"TinyLlama/TinyLlama-1.1B-Chat-v1.0",
|
||||
num_shard=1,
|
||||
disable_grammar_support=False,
|
||||
use_flash_attention=False,
|
||||
max_batch_prefill_tokens=3000,
|
||||
) as handle:
|
||||
yield handle
|
||||
|
||||
|
||||
@pytest.fixture(scope="module")
|
||||
async def llama_grammar(llama_grammar_handle):
|
||||
await llama_grammar_handle.health(300)
|
||||
return llama_grammar_handle.client
|
||||
|
||||
|
||||
@pytest.mark.asyncio
|
||||
async def test_grammar_response_format_llama_json(llama_grammar, response_snapshot):
|
||||
|
||||
class Weather(BaseModel):
|
||||
unit: str
|
||||
temperature: List[int]
|
||||
|
||||
# send the request
|
||||
response = requests.post(
|
||||
f"{llama_grammar.base_url}/v1/chat/completions",
|
||||
headers=llama_grammar.headers,
|
||||
json={
|
||||
"model": "tgi",
|
||||
"messages": [
|
||||
{
|
||||
"role": "system",
|
||||
"content": f"Respond to the users questions and answer them in the following format: {Weather.schema()}",
|
||||
},
|
||||
{
|
||||
"role": "user",
|
||||
"content": "What's the weather like the next 3 days in San Francisco, CA?",
|
||||
},
|
||||
],
|
||||
"seed": 42,
|
||||
"max_tokens": 500,
|
||||
"response_format": {"type": "json_object", "value": Weather.schema()},
|
||||
},
|
||||
)
|
||||
|
||||
chat_completion = response.json()
|
||||
called = chat_completion["choices"][0]["message"]["content"]
|
||||
|
||||
assert response.status_code == 200
|
||||
assert (
|
||||
called
|
||||
== '{\n "temperature": [\n 35,\n 34,\n 36\n ],\n "unit": "°c"\n}'
|
||||
)
|
||||
assert chat_completion == response_snapshot
|
||||
|
||||
|
||||
@pytest.mark.asyncio
|
||||
async def test_grammar_response_format_llama_error_if_tools_not_installed(
|
||||
llama_grammar,
|
||||
):
|
||||
class Weather(BaseModel):
|
||||
unit: str
|
||||
temperature: List[int]
|
||||
|
||||
# send the request
|
||||
response = requests.post(
|
||||
f"{llama_grammar.base_url}/v1/chat/completions",
|
||||
headers=llama_grammar.headers,
|
||||
json={
|
||||
"model": "tgi",
|
||||
"messages": [
|
||||
{
|
||||
"role": "system",
|
||||
"content": f"Respond to the users questions and answer them in the following format: {Weather.schema()}",
|
||||
},
|
||||
{
|
||||
"role": "user",
|
||||
"content": "What's the weather like the next 3 days in San Francisco, CA?",
|
||||
},
|
||||
],
|
||||
"seed": 42,
|
||||
"max_tokens": 500,
|
||||
"tools": [],
|
||||
"response_format": {"type": "json_object", "value": Weather.schema()},
|
||||
},
|
||||
)
|
||||
|
||||
# 422 means the server was unable to process the request because it contains invalid data.
|
||||
assert response.status_code == 422
|
||||
assert response.json() == {
|
||||
"error": "Grammar and tools are mutually exclusive",
|
||||
"error_type": "grammar and tools",
|
||||
}
|
|
@ -23,6 +23,12 @@ def get_chicken():
|
|||
return f"data:image/png;base64,{encoded_string.decode('utf-8')}"
|
||||
|
||||
|
||||
def get_cow_beach():
|
||||
with open("integration-tests/images/cow_beach.png", "rb") as image_file:
|
||||
encoded_string = base64.b64encode(image_file.read())
|
||||
return f"data:image/png;base64,{encoded_string.decode('utf-8')}"
|
||||
|
||||
|
||||
@pytest.mark.asyncio
|
||||
async def test_idefics(idefics, response_snapshot):
|
||||
chicken = get_chicken()
|
||||
|
@ -39,6 +45,21 @@ async def test_idefics(idefics, response_snapshot):
|
|||
assert response == response_snapshot
|
||||
|
||||
|
||||
@pytest.mark.asyncio
|
||||
@pytest.mark.private
|
||||
async def test_idefics_two_images(idefics, response_snapshot):
|
||||
chicken = get_chicken()
|
||||
cow_beach = get_cow_beach()
|
||||
response = await idefics.generate(
|
||||
f"User:Where are the cow and chicken?<end_of_utterance> \nAssistant:",
|
||||
max_new_tokens=20,
|
||||
)
|
||||
assert (
|
||||
response.generated_text == " The cow and chicken are on a beach."
|
||||
), f"{repr(response.generated_text)}"
|
||||
assert response == response_snapshot
|
||||
|
||||
|
||||
@pytest.mark.asyncio
|
||||
async def test_idefics_load(idefics, generate_load, response_snapshot):
|
||||
chicken = get_chicken()
|
||||
|
|
|
@ -9,6 +9,12 @@ def get_chicken():
|
|||
return f"data:image/png;base64,{encoded_string.decode('utf-8')}"
|
||||
|
||||
|
||||
def get_cow_beach():
|
||||
with open("integration-tests/images/cow_beach.png", "rb") as image_file:
|
||||
encoded_string = base64.b64encode(image_file.read())
|
||||
return f"data:image/png;base64,{encoded_string.decode('utf-8')}"
|
||||
|
||||
|
||||
@pytest.fixture(scope="module")
|
||||
def flash_idefics2_next_handle(launcher):
|
||||
with launcher(
|
||||
|
@ -38,6 +44,23 @@ async def test_flash_idefics2_next_simple(flash_idefics2_next, response_snapshot
|
|||
assert response == response_snapshot
|
||||
|
||||
|
||||
@pytest.mark.asyncio
|
||||
@pytest.mark.private
|
||||
async def test_flash_idefics2_two_images(flash_idefics2_next, response_snapshot):
|
||||
chicken = get_chicken()
|
||||
cow_beach = get_cow_beach()
|
||||
response = await flash_idefics2_next.generate(
|
||||
f"User:Where are the cow and chicken?<end_of_utterance> \nAssistant:",
|
||||
max_new_tokens=20,
|
||||
)
|
||||
assert (
|
||||
response.generated_text
|
||||
== " The cow is standing on the beach and the chicken is sitting on a pile of money."
|
||||
), f"{repr(response.generated_text)}"
|
||||
assert response.details.generated_tokens == 20
|
||||
assert response == response_snapshot
|
||||
|
||||
|
||||
@pytest.mark.asyncio
|
||||
@pytest.mark.private
|
||||
async def test_flash_idefics2_next_all_params(flash_idefics2_next, response_snapshot):
|
||||
|
|
|
@ -44,7 +44,8 @@ utoipa = { version = "4.2.0", features = ["axum_extras"] }
|
|||
utoipa-swagger-ui = { version = "6.0.0", features = ["axum"] }
|
||||
ngrok = { version = "0.13.1", features = ["axum"], optional = true }
|
||||
init-tracing-opentelemetry = { version = "0.14.1", features = ["opentelemetry-otlp"] }
|
||||
minijinja = { git = "https://github.com/mitsuhiko/minijinja.git", rev = "5cd4efb" }
|
||||
minijinja = { version = "2.0.2" }
|
||||
minijinja-contrib = { version = "2.0.2", features = ["pycompat"] }
|
||||
futures-util = "0.3.30"
|
||||
regex = "1.10.3"
|
||||
once_cell = "1.19.0"
|
||||
|
@ -58,3 +59,4 @@ vergen = { version = "8.2.5", features = ["build", "git", "gitcl"] }
|
|||
default = ["ngrok"]
|
||||
ngrok = ["dep:ngrok"]
|
||||
google = []
|
||||
kserve = []
|
||||
|
|
|
@ -1,7 +1,7 @@
|
|||
use std::fs;
|
||||
|
||||
fn main() -> Result<(), Box<dyn std::error::Error>> {
|
||||
println!("cargo:rerun-if-changed=../../proto/**");
|
||||
println!("cargo:rerun-if-changed=../../proto/");
|
||||
|
||||
fs::create_dir_all("src/v2/pb").unwrap_or(());
|
||||
let mut config = prost_build::Config::new();
|
||||
|
|
|
@ -12,6 +12,8 @@ use crate::{
|
|||
use crate::{FunctionRef, FunctionsMap, GrammarType, Properties, Tool, ToolType, Tools};
|
||||
use futures::future::try_join_all;
|
||||
use minijinja::{Environment, ErrorKind, Template};
|
||||
use minijinja_contrib::pycompat;
|
||||
|
||||
use serde_json::{json, Map, Value};
|
||||
use std::collections::HashMap;
|
||||
use std::sync::Arc;
|
||||
|
@ -62,14 +64,7 @@ impl Infer {
|
|||
.find(|t| t.name == "default")
|
||||
.map(|t| t.template),
|
||||
})
|
||||
.map(|t| {
|
||||
// .strip() is not supported in minijinja
|
||||
// .capitalize() is not supported in minijinja but we can use | capitalize
|
||||
let t = t
|
||||
.replace(".strip()", " | trim")
|
||||
.replace(".capitalize()", " | capitalize");
|
||||
ChatTemplate::new(t, tokenizer_config.bos_token, tokenizer_config.eos_token)
|
||||
});
|
||||
.map(|t| ChatTemplate::new(t, tokenizer_config.bos_token, tokenizer_config.eos_token));
|
||||
|
||||
// Inference limit with a semaphore
|
||||
let semaphore = Arc::new(Semaphore::new(max_concurrent_requests));
|
||||
|
@ -277,6 +272,8 @@ struct ChatTemplate {
|
|||
impl ChatTemplate {
|
||||
fn new(template: String, bos_token: Option<String>, eos_token: Option<String>) -> Self {
|
||||
let mut env = Box::new(Environment::new());
|
||||
// enable things like .strip() or .capitalize()
|
||||
env.set_unknown_method_callback(pycompat::unknown_method_callback);
|
||||
let template_str = template.into_boxed_str();
|
||||
env.add_function("raise_exception", raise_exception);
|
||||
|
||||
|
|
|
@ -0,0 +1,247 @@
|
|||
use crate::{
|
||||
default_parameters,
|
||||
server::{generate_internal, ComputeType},
|
||||
Deserialize, ErrorResponse, GenerateParameters, GenerateRequest, Infer, Serialize, ToSchema,
|
||||
};
|
||||
use axum::extract::{Extension, Path};
|
||||
use axum::response::{IntoResponse, Response};
|
||||
use axum::Json;
|
||||
use futures::stream::FuturesUnordered;
|
||||
use futures::TryStreamExt;
|
||||
use reqwest::header::HeaderMap;
|
||||
use reqwest::StatusCode;
|
||||
|
||||
#[derive(Debug, Serialize, Deserialize, ToSchema)]
|
||||
pub struct OutputChunk {
|
||||
pub name: String,
|
||||
pub shape: Vec<usize>,
|
||||
pub datatype: String,
|
||||
pub data: Vec<u8>,
|
||||
}
|
||||
|
||||
#[derive(Debug, Serialize, Deserialize, ToSchema)]
|
||||
pub struct InferenceOutput {
|
||||
pub id: String,
|
||||
pub outputs: Vec<OutputChunk>,
|
||||
}
|
||||
|
||||
#[derive(Debug, Deserialize, ToSchema)]
|
||||
pub(crate) struct InferenceRequest {
|
||||
pub id: String,
|
||||
#[serde(default = "default_parameters")]
|
||||
pub parameters: GenerateParameters,
|
||||
pub inputs: Vec<Input>,
|
||||
pub outputs: Vec<Output>,
|
||||
}
|
||||
|
||||
#[derive(Debug, Serialize, Deserialize, ToSchema)]
|
||||
pub(crate) struct Input {
|
||||
pub name: String,
|
||||
pub shape: Vec<usize>,
|
||||
pub datatype: String,
|
||||
pub data: Vec<u8>,
|
||||
}
|
||||
|
||||
#[derive(Debug, Serialize, Deserialize, ToSchema)]
|
||||
pub(crate) struct Output {
|
||||
pub name: String,
|
||||
}
|
||||
|
||||
#[derive(Debug, Serialize, Deserialize, ToSchema)]
|
||||
pub struct LiveResponse {
|
||||
pub live: bool,
|
||||
}
|
||||
|
||||
#[derive(Debug, Serialize, Deserialize, ToSchema)]
|
||||
pub struct ReadyResponse {
|
||||
pub live: bool,
|
||||
}
|
||||
|
||||
#[derive(Debug, Serialize, Deserialize, ToSchema)]
|
||||
pub struct MetadataServerResponse {
|
||||
pub name: String,
|
||||
pub version: String,
|
||||
pub extensions: Vec<String>,
|
||||
}
|
||||
|
||||
// Routes
|
||||
|
||||
#[utoipa::path(
|
||||
post,
|
||||
tag = "Text Generation Inference",
|
||||
path = "/v2/health/live",
|
||||
responses(
|
||||
(status = 200, description = "Service is live", body = LiveReponse),
|
||||
(status = 404, description = "Service not found", body = ErrorResponse,
|
||||
example = json!({"error": "No response"}))
|
||||
)
|
||||
)]
|
||||
pub async fn kserve_health_live() -> Result<Response, (StatusCode, Json<ErrorResponse>)> {
|
||||
let data = LiveResponse { live: true };
|
||||
Ok((HeaderMap::new(), Json(data)).into_response())
|
||||
}
|
||||
|
||||
#[utoipa::path(
|
||||
post,
|
||||
tag = "Text Generation Inference",
|
||||
path = "/v2/health/ready",
|
||||
responses(
|
||||
(status = 200, description = "Service is ready", body = ReadyResponse),
|
||||
(status = 404, description = "Service not found", body = ErrorResponse,
|
||||
example = json!({"error": "No response"}))
|
||||
)
|
||||
)]
|
||||
pub async fn kserve_health_ready() -> Result<Response, (StatusCode, Json<ErrorResponse>)> {
|
||||
let data = ReadyResponse { live: true };
|
||||
Ok((HeaderMap::new(), Json(data)).into_response())
|
||||
}
|
||||
|
||||
#[utoipa::path(
|
||||
get,
|
||||
tag = "Text Generation Inference",
|
||||
path = "/v2",
|
||||
responses(
|
||||
(status = 200, description = "Metadata retrieved", body = MetadataServerResponse),
|
||||
(status = 404, description = "Service not found", body = ErrorResponse,
|
||||
example = json!({"error": "No response"}))
|
||||
)
|
||||
)]
|
||||
pub async fn kerve_server_metadata() -> Result<Response, (StatusCode, Json<ErrorResponse>)> {
|
||||
let data = MetadataServerResponse {
|
||||
name: "text-generation-inference".to_string(),
|
||||
version: env!("CARGO_PKG_VERSION").to_string(),
|
||||
extensions: vec![
|
||||
"health".to_string(),
|
||||
"models".to_string(),
|
||||
"metrics".to_string(),
|
||||
],
|
||||
};
|
||||
Ok((HeaderMap::new(), Json(data)).into_response())
|
||||
}
|
||||
|
||||
#[utoipa::path(
|
||||
get,
|
||||
tag = "Text Generation Inference",
|
||||
path = "/v2/models/{model_name}/versions/{model_version}",
|
||||
responses(
|
||||
(status = 200, description = "Model version metadata retrieved", body = MetadataServerResponse),
|
||||
(status = 404, description = "Model or version not found", body = ErrorResponse,
|
||||
example = json!({"error": "No response"}))
|
||||
)
|
||||
)]
|
||||
pub async fn kserve_model_metadata(
|
||||
Path((model_name, model_version)): Path<(String, String)>,
|
||||
) -> Result<Response, (StatusCode, Json<ErrorResponse>)> {
|
||||
let data = MetadataServerResponse {
|
||||
name: model_name,
|
||||
version: model_version,
|
||||
extensions: vec!["infer".to_string(), "ready".to_string()],
|
||||
};
|
||||
Ok((HeaderMap::new(), Json(data)).into_response())
|
||||
}
|
||||
|
||||
#[utoipa::path(
|
||||
post,
|
||||
tag = "Text Generation Inference",
|
||||
path = "/v2/models/{model_name}/versions/{model_version}/infer",
|
||||
request_body = Json<InferenceRequest>,
|
||||
responses(
|
||||
(status = 200, description = "Inference executed successfully", body = InferenceOutput),
|
||||
(status = 404, description = "Model or version not found", body = ErrorResponse,
|
||||
example = json!({"error": "No response"}))
|
||||
)
|
||||
)]
|
||||
pub async fn kserve_model_infer(
|
||||
infer: Extension<Infer>,
|
||||
Extension(compute_type): Extension<ComputeType>,
|
||||
Json(payload): Json<InferenceRequest>,
|
||||
) -> Result<Response, (StatusCode, Json<ErrorResponse>)> {
|
||||
let id = payload.id.clone();
|
||||
let str_inputs = payload
|
||||
.inputs
|
||||
.iter()
|
||||
.map(|input| {
|
||||
std::str::from_utf8(&input.data).map_err(|e| {
|
||||
(
|
||||
StatusCode::UNPROCESSABLE_ENTITY,
|
||||
Json(ErrorResponse {
|
||||
error: e.to_string(),
|
||||
error_type: "utf8".to_string(),
|
||||
}),
|
||||
)
|
||||
})
|
||||
})
|
||||
.collect::<Result<Vec<_>, _>>()?;
|
||||
|
||||
if str_inputs.len() != payload.outputs.len() {
|
||||
return Err((
|
||||
StatusCode::UNPROCESSABLE_ENTITY,
|
||||
Json(ErrorResponse {
|
||||
error: "Inputs and outputs length mismatch".to_string(),
|
||||
error_type: "length mismatch".to_string(),
|
||||
}),
|
||||
));
|
||||
}
|
||||
|
||||
let output_chunks = str_inputs
|
||||
.iter()
|
||||
.zip(&payload.outputs)
|
||||
.map(|(str_input, output)| {
|
||||
let generate_request = GenerateRequest {
|
||||
inputs: str_input.to_string(),
|
||||
parameters: payload.parameters.clone(),
|
||||
};
|
||||
let infer = infer.clone();
|
||||
let compute_type = compute_type.clone();
|
||||
let span = tracing::Span::current();
|
||||
async move {
|
||||
generate_internal(infer, compute_type, Json(generate_request), span)
|
||||
.await
|
||||
.map(|(_, Json(generation))| {
|
||||
let generation_as_bytes = generation.generated_text.as_bytes().to_vec();
|
||||
OutputChunk {
|
||||
name: output.name.clone(),
|
||||
shape: vec![1, generation_as_bytes.len()],
|
||||
datatype: "BYTES".to_string(),
|
||||
data: generation_as_bytes,
|
||||
}
|
||||
})
|
||||
.map_err(|_| {
|
||||
(
|
||||
StatusCode::INTERNAL_SERVER_ERROR,
|
||||
Json(ErrorResponse {
|
||||
error: "Incomplete generation".into(),
|
||||
error_type: "Incomplete generation".into(),
|
||||
}),
|
||||
)
|
||||
})
|
||||
}
|
||||
})
|
||||
.collect::<FuturesUnordered<_>>()
|
||||
.try_collect::<Vec<_>>()
|
||||
.await?;
|
||||
|
||||
let inference_output = InferenceOutput {
|
||||
id: id.clone(),
|
||||
outputs: output_chunks,
|
||||
};
|
||||
|
||||
Ok((HeaderMap::new(), Json(inference_output)).into_response())
|
||||
}
|
||||
|
||||
#[utoipa::path(
|
||||
get,
|
||||
tag = "Text Generation Inference",
|
||||
path = "/v2/models/{model_name}/versions/{model_version}/ready",
|
||||
responses(
|
||||
(status = 200, description = "Model version is ready", body = ReadyResponse),
|
||||
(status = 404, description = "Model or version not found", body = ErrorResponse,
|
||||
example = json!({"error": "No response"}))
|
||||
)
|
||||
)]
|
||||
pub async fn kserve_model_metadata_ready(
|
||||
Path((_model_name, _model_version)): Path<(String, String)>,
|
||||
) -> Result<Response, (StatusCode, Json<ErrorResponse>)> {
|
||||
let data = ReadyResponse { live: true };
|
||||
Ok((HeaderMap::new(), Json(data)).into_response())
|
||||
}
|
|
@ -4,6 +4,9 @@ mod infer;
|
|||
pub mod server;
|
||||
mod validation;
|
||||
|
||||
#[cfg(feature = "kserve")]
|
||||
mod kserve;
|
||||
|
||||
use serde::{Deserialize, Serialize};
|
||||
use tracing::warn;
|
||||
use utoipa::ToSchema;
|
||||
|
@ -89,6 +92,7 @@ pub(crate) enum GrammarType {
|
|||
/// JSON Schema is a declarative language that allows to annotate JSON documents
|
||||
/// with types and descriptions.
|
||||
#[serde(rename = "json")]
|
||||
#[serde(alias = "json_object")]
|
||||
#[schema(example = json ! ({"properties": {"location":{"type": "string"}}}))]
|
||||
Json(serde_json::Value),
|
||||
#[serde(rename = "regex")]
|
||||
|
@ -791,6 +795,13 @@ pub(crate) struct ChatRequest {
|
|||
#[schema(nullable = true, example = "null")]
|
||||
#[serde(deserialize_with = "deserialize_tool_choice::deserialize")]
|
||||
pub tool_choice: Option<ToolType>,
|
||||
|
||||
/// Response format constraints for the generation.
|
||||
///
|
||||
/// NOTE: A request can use `response_format` OR `tools` but not both.
|
||||
#[serde(default)]
|
||||
#[schema(nullable = true, default = "null", example = "null")]
|
||||
pub response_format: Option<GrammarType>,
|
||||
}
|
||||
|
||||
fn default_tool_prompt() -> Option<String> {
|
||||
|
|
|
@ -4,6 +4,11 @@ use crate::infer::v2::SchedulerV2;
|
|||
use crate::infer::v3::SchedulerV3;
|
||||
use crate::infer::{HealthCheck, Scheduler};
|
||||
use crate::infer::{Infer, InferError, InferResponse, InferStreamResponse, ToolGrammar};
|
||||
#[cfg(feature = "kserve")]
|
||||
use crate::kserve::{
|
||||
kerve_server_metadata, kserve_health_live, kserve_health_ready, kserve_model_infer,
|
||||
kserve_model_metadata, kserve_model_metadata_ready,
|
||||
};
|
||||
use crate::validation::ValidationError;
|
||||
use crate::{
|
||||
BestOfSequence, Details, ErrorResponse, FinishReason, GenerateParameters, GenerateRequest,
|
||||
|
@ -172,7 +177,7 @@ async fn generate(
|
|||
generate_internal(infer, ComputeType(compute_type), Json(req), span).await
|
||||
}
|
||||
|
||||
async fn generate_internal(
|
||||
pub(crate) async fn generate_internal(
|
||||
infer: Extension<Infer>,
|
||||
ComputeType(compute_type): ComputeType,
|
||||
Json(req): Json<GenerateRequest>,
|
||||
|
@ -1016,6 +1021,7 @@ async fn chat_completions(
|
|||
tool_choice,
|
||||
tool_prompt,
|
||||
temperature,
|
||||
response_format,
|
||||
..
|
||||
} = req;
|
||||
|
||||
|
@ -1030,6 +1036,18 @@ async fn chat_completions(
|
|||
other => (true, other),
|
||||
};
|
||||
|
||||
// response_format and tools are mutually exclusive
|
||||
if response_format.is_some() && tools.as_ref().is_some() {
|
||||
metrics::increment_counter!("tgi_request_failure", "err" => "validation");
|
||||
return Err((
|
||||
StatusCode::UNPROCESSABLE_ENTITY,
|
||||
Json(ErrorResponse {
|
||||
error: "Grammar and tools are mutually exclusive".to_string(),
|
||||
error_type: "grammar and tools".to_string(),
|
||||
}),
|
||||
));
|
||||
}
|
||||
|
||||
// extract tool grammar if present
|
||||
let tool_grammar = match ToolGrammar::apply(tools, tool_choice) {
|
||||
Ok(grammar) => grammar,
|
||||
|
@ -1046,16 +1064,21 @@ async fn chat_completions(
|
|||
}
|
||||
};
|
||||
|
||||
let grammar_with_prompt = tool_grammar
|
||||
// determine the appropriate arguments for apply_chat_template
|
||||
let tools_grammar_prompt = tool_grammar
|
||||
.as_ref()
|
||||
.map(|t| (GrammarType::Json(serde_json::json!(t)), tool_prompt));
|
||||
|
||||
let typed_grammar = grammar_with_prompt
|
||||
.as_ref()
|
||||
.map(|(grammar, _)| grammar.clone());
|
||||
let (tools_grammar_prompt, grammar) = match response_format {
|
||||
Some(response_format) => (None, Some(response_format)),
|
||||
None => (
|
||||
tools_grammar_prompt.clone(),
|
||||
tools_grammar_prompt.map(|(grammar, _)| grammar.clone()),
|
||||
),
|
||||
};
|
||||
|
||||
// apply chat template to flatten the request into a single input
|
||||
let inputs = match infer.apply_chat_template(messages, grammar_with_prompt) {
|
||||
let inputs = match infer.apply_chat_template(messages, tools_grammar_prompt) {
|
||||
Ok(inputs) => inputs,
|
||||
Err(err) => {
|
||||
metrics::increment_counter!("tgi_request_failure", "err" => "validation");
|
||||
|
@ -1091,7 +1114,7 @@ async fn chat_completions(
|
|||
decoder_input_details: !stream,
|
||||
seed,
|
||||
top_n_tokens: req.top_logprobs,
|
||||
grammar: typed_grammar,
|
||||
grammar,
|
||||
},
|
||||
};
|
||||
|
||||
|
@ -1709,28 +1732,58 @@ pub async fn run(
|
|||
docker_label: option_env!("DOCKER_LABEL"),
|
||||
};
|
||||
|
||||
// Define VertextApiDoc conditionally only if the "google" feature is enabled
|
||||
let doc = {
|
||||
// avoid `mut` if possible
|
||||
#[cfg(feature = "google")]
|
||||
{
|
||||
use crate::VertexInstance;
|
||||
#[allow(unused_mut)] // mut is needed for conditional compilation
|
||||
let mut doc = ApiDoc::openapi();
|
||||
|
||||
#[derive(OpenApi)]
|
||||
#[openapi(
|
||||
paths(vertex_compatibility),
|
||||
components(schemas(VertexInstance, VertexRequest, VertexResponse))
|
||||
)]
|
||||
struct VertextApiDoc;
|
||||
#[cfg(feature = "google")]
|
||||
{
|
||||
use crate::VertexInstance;
|
||||
|
||||
// limiting mutability to the smallest scope necessary
|
||||
let mut doc = ApiDoc::openapi();
|
||||
doc.merge(VertextApiDoc::openapi());
|
||||
doc
|
||||
}
|
||||
#[cfg(not(feature = "google"))]
|
||||
ApiDoc::openapi()
|
||||
};
|
||||
#[derive(OpenApi)]
|
||||
#[openapi(
|
||||
paths(vertex_compatibility),
|
||||
components(schemas(VertexInstance, VertexRequest, VertexResponse))
|
||||
)]
|
||||
struct VertexApiDoc;
|
||||
|
||||
doc.merge(VertexApiDoc::openapi());
|
||||
}
|
||||
|
||||
#[cfg(feature = "kserve")]
|
||||
{
|
||||
use crate::kserve::{
|
||||
InferenceOutput, InferenceRequest, LiveResponse, MetadataServerResponse, OutputChunk,
|
||||
ReadyResponse,
|
||||
};
|
||||
use crate::kserve::{
|
||||
__path_kerve_server_metadata, __path_kserve_health_live, __path_kserve_health_ready,
|
||||
__path_kserve_model_infer, __path_kserve_model_metadata,
|
||||
__path_kserve_model_metadata_ready,
|
||||
};
|
||||
|
||||
#[derive(OpenApi)]
|
||||
#[openapi(
|
||||
paths(
|
||||
kserve_model_infer,
|
||||
kserve_health_live,
|
||||
kserve_health_ready,
|
||||
kerve_server_metadata,
|
||||
kserve_model_metadata,
|
||||
kserve_model_metadata_ready,
|
||||
),
|
||||
components(schemas(
|
||||
InferenceOutput,
|
||||
InferenceRequest,
|
||||
LiveResponse,
|
||||
MetadataServerResponse,
|
||||
OutputChunk,
|
||||
ReadyResponse,
|
||||
))
|
||||
)]
|
||||
struct KServeApiDoc;
|
||||
|
||||
doc.merge(KServeApiDoc::openapi());
|
||||
}
|
||||
|
||||
// Configure Swagger UI
|
||||
let swagger_ui = SwaggerUi::new("/docs").url("/api-doc/openapi.json", doc);
|
||||
|
@ -1780,6 +1833,27 @@ pub async fn run(
|
|||
}
|
||||
}
|
||||
|
||||
#[cfg(feature = "kserve")]
|
||||
{
|
||||
tracing::info!("Built with `kserve` feature");
|
||||
app = app
|
||||
.route(
|
||||
"/v2/models/:model_name/versions/:model_version/infer",
|
||||
post(kserve_model_infer),
|
||||
)
|
||||
.route(
|
||||
"/v2/models/:model_name/versions/:model_version",
|
||||
get(kserve_model_metadata),
|
||||
)
|
||||
.route("/v2/health/ready", get(kserve_health_ready))
|
||||
.route("/v2/health/live", get(kserve_health_live))
|
||||
.route("/v2", get(kerve_server_metadata))
|
||||
.route(
|
||||
"/v2/models/:model_name/versions/:model_version/ready",
|
||||
get(kserve_model_metadata_ready),
|
||||
);
|
||||
}
|
||||
|
||||
// add layers after routes
|
||||
app = app
|
||||
.layer(Extension(info))
|
||||
|
|
|
@ -1,5 +1,5 @@
|
|||
[toolchain]
|
||||
# Released on: 13 June, 2024
|
||||
# Released on: June 13, 2024
|
||||
# https://releases.rs/docs/1.79.0/
|
||||
channel = "1.79.0"
|
||||
components = ["rustfmt", "clippy"]
|
||||
|
|
|
@ -3,7 +3,6 @@ include Makefile-flash-att-v2
|
|||
include Makefile-vllm
|
||||
include Makefile-awq
|
||||
include Makefile-eetq
|
||||
include Makefile-marlin
|
||||
include Makefile-selective-scan
|
||||
|
||||
unit-tests:
|
||||
|
|
|
@ -1,11 +0,0 @@
|
|||
marlin_commit := 2f6d7c10e124b3c5fa29ff8d77d568bd7af3274c
|
||||
|
||||
build-marlin:
|
||||
if [ ! -d 'marlin' ]; then \
|
||||
pip install -U ninja packaging --no-cache-dir && \
|
||||
git clone https://github.com/IST-DASLab/marlin.git marlin; \
|
||||
fi
|
||||
cd marlin && git fetch && git checkout $(marlin_commit) && python setup.py build
|
||||
|
||||
install-marlin: build-marlin
|
||||
cd marlin && git fetch && git checkout $(marlin_commit) && pip install -e .
|
|
@ -1,5 +1,5 @@
|
|||
commit_cuda := b5dfc61db88a81069e45b44f7cc99bd9e62a60fa
|
||||
commit_rocm := 559200c1a028de990c1ddea761b0ccd62109e3a0
|
||||
commit_rocm := c6ee53b1be97e3bbc791b95f22827501297f8921
|
||||
build-vllm-cuda:
|
||||
if [ ! -d 'vllm' ]; then \
|
||||
pip install -U ninja packaging --no-cache-dir && \
|
||||
|
|
|
@ -0,0 +1,20 @@
|
|||
These kernels were vendored from VLLM. The Marlin kernels were developed
|
||||
by Elias Frantar and extended by Neural Magic.
|
||||
|
||||
---
|
||||
|
||||
Copyright (C) Marlin.2024 Elias Frantar
|
||||
Modified by Neural Magic
|
||||
Copyright 2024 The vLLM team.
|
||||
|
||||
Licensed under the Apache License, Version 2.0 (the "License");
|
||||
you may not use this file except in compliance with the License.
|
||||
You may obtain a copy of the License at
|
||||
|
||||
http://www.apache.org/licenses/LICENSE-2.0
|
||||
|
||||
Unless required by applicable law or agreed to in writing, software
|
||||
distributed under the License is distributed on an "AS IS" BASIS,
|
||||
WITHOUT WARRANTIES OR CONDITIONS OF ANY KIND, either express or implied.
|
||||
See the License for the specific language governing permissions and
|
||||
limitations under the License.
|
|
@ -0,0 +1,44 @@
|
|||
import torch
|
||||
|
||||
def gptq_marlin_gemm(
|
||||
a: torch.Tensor,
|
||||
b_q_weight: torch.Tensor,
|
||||
b_scales: torch.Tensor,
|
||||
g_idx: torch.Tensor,
|
||||
perm: torch.Tensor,
|
||||
workspace: torch.Tensor,
|
||||
num_bits: int,
|
||||
size_m: int,
|
||||
size_n: int,
|
||||
size_k: int,
|
||||
is_k_full: bool,
|
||||
) -> torch.Tensor:
|
||||
"""
|
||||
Matrix multiplication using Marlin kernels. This is an extension of
|
||||
`marlin_gemm` that supports converted GPTQ kernels.
|
||||
"""
|
||||
...
|
||||
|
||||
def gptq_marlin_repack(
|
||||
b_q_weight: torch.Tensor,
|
||||
perm: torch.Tensor,
|
||||
size_k: int,
|
||||
size_n: int,
|
||||
num_bits: int,
|
||||
) -> torch.Tensor:
|
||||
"""Repack GPTQ parameters for Marlin kernels."""
|
||||
...
|
||||
|
||||
def marlin_gemm(
|
||||
a: torch.Tensor,
|
||||
b_q_weight: torch.Tensor,
|
||||
b_scales: torch.Tensor,
|
||||
workspace: torch.Tensor,
|
||||
size_m: int,
|
||||
size_n: int,
|
||||
size_k: int,
|
||||
) -> torch.Tensor:
|
||||
"""
|
||||
Matrix multiplication using Marlin kernels.
|
||||
"""
|
||||
...
|
|
@ -0,0 +1,11 @@
|
|||
#include <torch/extension.h>
|
||||
|
||||
#include "ext.hh"
|
||||
|
||||
PYBIND11_MODULE(TORCH_EXTENSION_NAME, m) {
|
||||
m.def("gptq_marlin_gemm", &gptq_marlin_gemm,
|
||||
"Marlin gemm with GPTQ compatibility");
|
||||
m.def("gptq_marlin_repack", &gptq_marlin_repack,
|
||||
"Repack GPTQ parameters for Marlin");
|
||||
m.def("marlin_gemm", &marlin_gemm, "Marlin gemm");
|
||||
}
|
|
@ -0,0 +1,23 @@
|
|||
#pragma once
|
||||
|
||||
#include <torch/library.h>
|
||||
|
||||
#if defined(__CUDA_ARCH__) && __CUDA_ARCH__ < 800
|
||||
// No support for async
|
||||
#else
|
||||
|
||||
torch::Tensor gptq_marlin_gemm(torch::Tensor &a, torch::Tensor &b_q_weight,
|
||||
torch::Tensor &b_scales, torch::Tensor &g_idx,
|
||||
torch::Tensor &perm, torch::Tensor &workspace,
|
||||
int64_t num_bits, int64_t size_m, int64_t size_n,
|
||||
int64_t size_k, bool is_k_full);
|
||||
|
||||
torch::Tensor gptq_marlin_repack(torch::Tensor &b_q_weight, torch::Tensor &perm,
|
||||
int64_t size_k, int64_t size_n,
|
||||
int64_t num_bits);
|
||||
|
||||
torch::Tensor marlin_gemm(torch::Tensor &a, torch::Tensor &b_q_weight,
|
||||
torch::Tensor &b_scales, torch::Tensor &workspace,
|
||||
int64_t size_m, int64_t size_n, int64_t size_k);
|
||||
|
||||
#endif
|
File diff suppressed because it is too large
Load Diff
|
@ -0,0 +1,76 @@
|
|||
#pragma once
|
||||
|
||||
#include <torch/all.h>
|
||||
|
||||
#include <ATen/cuda/CUDAContext.h>
|
||||
#include <c10/cuda/CUDAGuard.h>
|
||||
#include <cuda.h>
|
||||
#include <cuda_fp16.h>
|
||||
#include <cuda_runtime.h>
|
||||
#include <iostream>
|
||||
|
||||
namespace gptq_marlin {
|
||||
|
||||
// 8 warps are a good choice since every SM has 4 schedulers and having more
|
||||
// than 1 warp per schedule allows some more latency hiding. At the same time,
|
||||
// we want relatively few warps to have many registers per warp and small tiles.
|
||||
static constexpr int default_threads = 256;
|
||||
|
||||
static constexpr int pipe_stages =
|
||||
4; // 4 pipeline stages fit into shared memory
|
||||
|
||||
static constexpr int min_thread_n = 64;
|
||||
static constexpr int min_thread_k = 64;
|
||||
|
||||
static constexpr int tile_size = 16;
|
||||
static constexpr int max_par = 16;
|
||||
|
||||
template <typename T, int n>
|
||||
struct Vec {
|
||||
T elems[n];
|
||||
__device__ T& operator[](int i) { return elems[i]; }
|
||||
};
|
||||
|
||||
using I4 = Vec<int, 4>;
|
||||
|
||||
constexpr int div_ceil(int a, int b) { return (a + b - 1) / b; }
|
||||
|
||||
#if defined(__CUDA_ARCH__) && __CUDA_ARCH__ < 800
|
||||
// No support for async
|
||||
#else
|
||||
|
||||
__device__ inline void cp_async4_pred(void* smem_ptr, const void* glob_ptr,
|
||||
bool pred = true) {
|
||||
const int BYTES = 16;
|
||||
uint32_t smem = static_cast<uint32_t>(__cvta_generic_to_shared(smem_ptr));
|
||||
asm volatile(
|
||||
"{\n"
|
||||
" .reg .pred p;\n"
|
||||
" setp.ne.b32 p, %0, 0;\n"
|
||||
" @p cp.async.cg.shared.global [%1], [%2], %3;\n"
|
||||
"}\n" ::"r"((int)pred),
|
||||
"r"(smem), "l"(glob_ptr), "n"(BYTES));
|
||||
}
|
||||
|
||||
__device__ inline void cp_async4(void* smem_ptr, const void* glob_ptr) {
|
||||
const int BYTES = 16;
|
||||
uint32_t smem = static_cast<uint32_t>(__cvta_generic_to_shared(smem_ptr));
|
||||
asm volatile(
|
||||
"{\n"
|
||||
" cp.async.cg.shared.global [%0], [%1], %2;\n"
|
||||
"}\n" ::"r"(smem),
|
||||
"l"(glob_ptr), "n"(BYTES));
|
||||
}
|
||||
|
||||
__device__ inline void cp_async_fence() {
|
||||
asm volatile("cp.async.commit_group;\n" ::);
|
||||
}
|
||||
|
||||
template <int n>
|
||||
__device__ inline void cp_async_wait() {
|
||||
asm volatile("cp.async.wait_group %0;\n" ::"n"(n));
|
||||
}
|
||||
|
||||
#endif
|
||||
|
||||
} // namespace gptq_marlin
|
|
@ -0,0 +1,77 @@
|
|||
|
||||
#ifndef _data_types_cuh
|
||||
#define _data_types_cuh
|
||||
#include "gptq_marlin.cuh"
|
||||
#include <cuda_fp16.h>
|
||||
#include <cuda_bf16.h>
|
||||
|
||||
namespace gptq_marlin {
|
||||
|
||||
template <typename scalar_t>
|
||||
class ScalarType {};
|
||||
|
||||
template <>
|
||||
class ScalarType<half> {
|
||||
public:
|
||||
using scalar_t = half;
|
||||
using scalar_t2 = half2;
|
||||
|
||||
// Matrix fragments for tensor core instructions; their precise layout is
|
||||
// documented here:
|
||||
// https://docs.nvidia.com/cuda/parallel-thread-execution/index.html#matrix-fragments-for-mma-m16n8k16-with-floating-point-type
|
||||
using FragA = Vec<half2, 4>;
|
||||
using FragB = Vec<half2, 2>;
|
||||
using FragC = Vec<float, 4>;
|
||||
using FragS = Vec<half2, 1>;
|
||||
|
||||
static __device__ float inline num2float(const half x) {
|
||||
return __half2float(x);
|
||||
}
|
||||
|
||||
static __device__ half2 inline num2num2(const half x) {
|
||||
return __half2half2(x);
|
||||
}
|
||||
|
||||
static __device__ half2 inline nums2num2(const half x1, const half x2) {
|
||||
return __halves2half2(x1, x2);
|
||||
}
|
||||
|
||||
static __host__ __device__ half inline float2num(const float x) {
|
||||
return __float2half(x);
|
||||
}
|
||||
};
|
||||
|
||||
template <>
|
||||
class ScalarType<nv_bfloat16> {
|
||||
public:
|
||||
using scalar_t = nv_bfloat16;
|
||||
using scalar_t2 = nv_bfloat162;
|
||||
|
||||
using FragA = Vec<nv_bfloat162, 4>;
|
||||
using FragB = Vec<nv_bfloat162, 2>;
|
||||
using FragC = Vec<float, 4>;
|
||||
using FragS = Vec<nv_bfloat162, 1>;
|
||||
|
||||
#if defined(__CUDA_ARCH__) && __CUDA_ARCH__ >= 800
|
||||
static __device__ float inline num2float(const nv_bfloat16 x) {
|
||||
return __bfloat162float(x);
|
||||
}
|
||||
|
||||
static __device__ nv_bfloat162 inline num2num2(const nv_bfloat16 x) {
|
||||
return __bfloat162bfloat162(x);
|
||||
}
|
||||
|
||||
static __device__ nv_bfloat162 inline nums2num2(const nv_bfloat16 x1,
|
||||
const nv_bfloat16 x2) {
|
||||
return __halves2bfloat162(x1, x2);
|
||||
}
|
||||
|
||||
static __host__ __device__ nv_bfloat16 inline float2num(const float x) {
|
||||
return __float2bfloat16(x);
|
||||
}
|
||||
#endif
|
||||
};
|
||||
|
||||
} // namespace gptq_marlin
|
||||
|
||||
#endif
|
|
@ -0,0 +1,350 @@
|
|||
#include "gptq_marlin.cuh"
|
||||
|
||||
namespace gptq_marlin {
|
||||
|
||||
static constexpr int repack_stages = 8;
|
||||
|
||||
static constexpr int repack_threads = 256;
|
||||
|
||||
static constexpr int tile_k_size = tile_size;
|
||||
static constexpr int tile_n_size = tile_k_size * 4;
|
||||
|
||||
#if defined(__CUDA_ARCH__) && __CUDA_ARCH__ < 800
|
||||
|
||||
template <int const num_threads, int const num_bits, bool const has_perm>
|
||||
__global__ void marlin_repack_kernel(
|
||||
uint32_t const* __restrict__ b_q_weight_ptr,
|
||||
uint32_t const* __restrict__ perm_ptr, uint32_t* __restrict__ out_ptr,
|
||||
int size_k, int size_n) {}
|
||||
|
||||
} // namespace gptq_marlin
|
||||
|
||||
torch::Tensor gptq_marlin_repack(torch::Tensor& b_q_weight, torch::Tensor& perm,
|
||||
int64_t size_k, int64_t size_n,
|
||||
int64_t num_bits) {
|
||||
TORCH_CHECK_NOT_IMPLEMENTED(
|
||||
false, "marlin_repack_from_gptq(..) requires CUDA_ARCH >= 8.0");
|
||||
return torch::empty({1, 1});
|
||||
}
|
||||
|
||||
#else
|
||||
|
||||
template <int const num_threads, int const num_bits, bool const has_perm>
|
||||
__global__ void marlin_repack_kernel(
|
||||
uint32_t const* __restrict__ b_q_weight_ptr,
|
||||
uint32_t const* __restrict__ perm_ptr, uint32_t* __restrict__ out_ptr,
|
||||
int size_k, int size_n) {
|
||||
constexpr int pack_factor = 32 / num_bits;
|
||||
|
||||
int k_tiles = size_k / tile_k_size;
|
||||
int n_tiles = size_n / tile_n_size;
|
||||
int block_k_tiles = div_ceil(k_tiles, gridDim.x);
|
||||
|
||||
int start_k_tile = blockIdx.x * block_k_tiles;
|
||||
if (start_k_tile >= k_tiles) {
|
||||
return;
|
||||
}
|
||||
|
||||
int finish_k_tile = min(start_k_tile + block_k_tiles, k_tiles);
|
||||
|
||||
// Wait until the next thread tile has been loaded to shared memory.
|
||||
auto wait_for_stage = [&]() {
|
||||
// We only have `stages - 2` active fetches since we are double buffering
|
||||
// and can only issue the next fetch when it is guaranteed that the previous
|
||||
// shared memory load is fully complete (as it may otherwise be
|
||||
// overwritten).
|
||||
cp_async_wait<repack_stages - 2>();
|
||||
__syncthreads();
|
||||
};
|
||||
|
||||
extern __shared__ int4 sh[];
|
||||
|
||||
constexpr int perm_size = tile_k_size / 4;
|
||||
|
||||
int4* sh_perm_ptr = sh;
|
||||
int4* sh_pipe_ptr = sh_perm_ptr;
|
||||
if constexpr (has_perm) {
|
||||
sh_pipe_ptr += perm_size;
|
||||
}
|
||||
|
||||
constexpr int tile_ints = tile_k_size / pack_factor;
|
||||
|
||||
constexpr int stage_n_threads = tile_n_size / 4;
|
||||
constexpr int stage_k_threads = has_perm ? tile_k_size : tile_ints;
|
||||
constexpr int stage_size = stage_k_threads * stage_n_threads;
|
||||
|
||||
auto load_perm_to_shared = [&](int k_tile_id) {
|
||||
int first_k_int4 = (k_tile_id * tile_k_size) / 4;
|
||||
|
||||
int4 const* perm_int4_ptr = reinterpret_cast<int4 const*>(perm_ptr);
|
||||
|
||||
if (threadIdx.x < perm_size) {
|
||||
sh_perm_ptr[threadIdx.x] = perm_int4_ptr[first_k_int4 + threadIdx.x];
|
||||
}
|
||||
__syncthreads();
|
||||
};
|
||||
|
||||
auto fetch_to_shared = [&](int pipe, int k_tile_id, int n_tile_id) {
|
||||
if (n_tile_id >= n_tiles) {
|
||||
cp_async_fence();
|
||||
return;
|
||||
}
|
||||
|
||||
int first_n = n_tile_id * tile_n_size;
|
||||
|
||||
int4* sh_ptr = sh_pipe_ptr + stage_size * pipe;
|
||||
|
||||
if constexpr (has_perm) {
|
||||
if (threadIdx.x < stage_size) {
|
||||
int k_id = threadIdx.x / stage_n_threads;
|
||||
int n_id = threadIdx.x % stage_n_threads;
|
||||
|
||||
uint32_t const* sh_perm_int_ptr =
|
||||
reinterpret_cast<uint32_t const*>(sh_perm_ptr);
|
||||
|
||||
int src_k = sh_perm_int_ptr[k_id];
|
||||
int src_k_packed = src_k / pack_factor;
|
||||
|
||||
cp_async4(
|
||||
&sh_ptr[k_id * stage_n_threads + n_id],
|
||||
reinterpret_cast<int4 const*>(&(
|
||||
b_q_weight_ptr[src_k_packed * size_n + first_n + (n_id * 4)])));
|
||||
}
|
||||
|
||||
} else {
|
||||
if (threadIdx.x < stage_size) {
|
||||
int k_id = threadIdx.x / stage_n_threads;
|
||||
int n_id = threadIdx.x % stage_n_threads;
|
||||
|
||||
int first_k = k_tile_id * tile_k_size;
|
||||
int first_k_packed = first_k / pack_factor;
|
||||
|
||||
cp_async4(&sh_ptr[k_id * stage_n_threads + n_id],
|
||||
reinterpret_cast<int4 const*>(
|
||||
&(b_q_weight_ptr[(first_k_packed + k_id) * size_n +
|
||||
first_n + (n_id * 4)])));
|
||||
}
|
||||
}
|
||||
|
||||
cp_async_fence();
|
||||
};
|
||||
|
||||
auto repack_tile = [&](int pipe, int k_tile_id, int n_tile_id) {
|
||||
if (n_tile_id >= n_tiles) {
|
||||
return;
|
||||
}
|
||||
|
||||
int warp_id = threadIdx.x / 32;
|
||||
int th_id = threadIdx.x % 32;
|
||||
|
||||
if (warp_id >= 4) {
|
||||
return;
|
||||
}
|
||||
|
||||
int tc_col = th_id / 4;
|
||||
int tc_row = (th_id % 4) * 2;
|
||||
|
||||
constexpr int tc_offsets[4] = {0, 1, 8, 9};
|
||||
|
||||
int cur_n = warp_id * 16 + tc_col;
|
||||
|
||||
constexpr int sh_stride = 64;
|
||||
constexpr uint32_t mask = (1 << num_bits) - 1;
|
||||
|
||||
int4* sh_stage_ptr = sh_pipe_ptr + stage_size * pipe;
|
||||
uint32_t* sh_stage_int_ptr = reinterpret_cast<uint32_t*>(sh_stage_ptr);
|
||||
|
||||
uint32_t* sh_perm_int_ptr = reinterpret_cast<uint32_t*>(sh_perm_ptr);
|
||||
|
||||
uint32_t vals[8];
|
||||
|
||||
if constexpr (has_perm) {
|
||||
for (int i = 0; i < 4; i++) {
|
||||
int k_idx = tc_row + tc_offsets[i];
|
||||
|
||||
uint32_t src_k = sh_perm_int_ptr[k_idx];
|
||||
uint32_t src_k_pos = src_k % pack_factor;
|
||||
|
||||
uint32_t b1_val = sh_stage_int_ptr[k_idx * sh_stride + cur_n];
|
||||
uint32_t b1_cur_val = (b1_val >> (src_k_pos * num_bits)) & mask;
|
||||
|
||||
uint32_t b2_val = sh_stage_int_ptr[k_idx * sh_stride + cur_n + 8];
|
||||
uint32_t b2_cur_val = (b2_val >> (src_k_pos * num_bits)) & mask;
|
||||
|
||||
vals[i] = b1_cur_val;
|
||||
vals[4 + i] = b2_cur_val;
|
||||
}
|
||||
|
||||
} else {
|
||||
uint32_t b1_vals[tile_ints];
|
||||
uint32_t b2_vals[tile_ints];
|
||||
|
||||
#pragma unroll
|
||||
for (int i = 0; i < tile_ints; i++) {
|
||||
b1_vals[i] = sh_stage_int_ptr[cur_n + sh_stride * i];
|
||||
b2_vals[i] = sh_stage_int_ptr[cur_n + 8 + sh_stride * i];
|
||||
}
|
||||
|
||||
#pragma unroll
|
||||
for (int i = 0; i < 4; i++) {
|
||||
int cur_elem = tc_row + tc_offsets[i];
|
||||
int cur_int = cur_elem / pack_factor;
|
||||
int cur_pos = cur_elem % pack_factor;
|
||||
|
||||
vals[i] = (b1_vals[cur_int] >> (cur_pos * num_bits)) & mask;
|
||||
vals[4 + i] = (b2_vals[cur_int] >> (cur_pos * num_bits)) & mask;
|
||||
}
|
||||
}
|
||||
|
||||
constexpr int tile_size = tile_k_size * tile_n_size / pack_factor;
|
||||
int out_offset = (k_tile_id * n_tiles + n_tile_id) * tile_size;
|
||||
|
||||
// Result of:
|
||||
// https://github.com/NVIDIA/FasterTransformer/blob/main/src/fastertransformer/cutlass_extensions/include/cutlass_extensions/interleaved_numeric_conversion.h
|
||||
if constexpr (num_bits == 4) {
|
||||
constexpr int pack_idx[8] = {0, 2, 4, 6, 1, 3, 5, 7};
|
||||
|
||||
uint32_t res = 0;
|
||||
#pragma unroll
|
||||
for (int i = 0; i < 8; i++) {
|
||||
res |= vals[pack_idx[i]] << (i * 4);
|
||||
}
|
||||
|
||||
out_ptr[out_offset + th_id * 4 + warp_id] = res;
|
||||
|
||||
} else {
|
||||
constexpr int pack_idx[4] = {0, 2, 1, 3};
|
||||
|
||||
uint32_t res1 = 0;
|
||||
uint32_t res2 = 0;
|
||||
#pragma unroll
|
||||
for (int i = 0; i < 4; i++) {
|
||||
res1 |= vals[pack_idx[i]] << (i * 8);
|
||||
res2 |= vals[4 + pack_idx[i]] << (i * 8);
|
||||
}
|
||||
|
||||
out_ptr[out_offset + th_id * 8 + (warp_id * 2) + 0] = res1;
|
||||
out_ptr[out_offset + th_id * 8 + (warp_id * 2) + 1] = res2;
|
||||
}
|
||||
};
|
||||
|
||||
auto start_pipes = [&](int k_tile_id, int n_tile_id) {
|
||||
#pragma unroll
|
||||
for (int pipe = 0; pipe < repack_stages - 1; pipe++) {
|
||||
fetch_to_shared(pipe, k_tile_id, n_tile_id + pipe);
|
||||
}
|
||||
|
||||
wait_for_stage();
|
||||
};
|
||||
#pragma unroll
|
||||
for (int k_tile_id = start_k_tile; k_tile_id < finish_k_tile; k_tile_id++) {
|
||||
int n_tile_id = 0;
|
||||
|
||||
if constexpr (has_perm) {
|
||||
load_perm_to_shared(k_tile_id);
|
||||
}
|
||||
|
||||
start_pipes(k_tile_id, n_tile_id);
|
||||
|
||||
while (n_tile_id < n_tiles) {
|
||||
#pragma unroll
|
||||
for (int pipe = 0; pipe < repack_stages; pipe++) {
|
||||
fetch_to_shared((pipe + repack_stages - 1) % repack_stages, k_tile_id,
|
||||
n_tile_id + pipe + repack_stages - 1);
|
||||
repack_tile(pipe, k_tile_id, n_tile_id + pipe);
|
||||
wait_for_stage();
|
||||
}
|
||||
n_tile_id += repack_stages;
|
||||
}
|
||||
}
|
||||
}
|
||||
|
||||
} // namespace gptq_marlin
|
||||
|
||||
#define CALL_IF(NUM_BITS, HAS_PERM) \
|
||||
else if (num_bits == NUM_BITS && has_perm == HAS_PERM) { \
|
||||
cudaFuncSetAttribute( \
|
||||
gptq_marlin::marlin_repack_kernel<gptq_marlin::repack_threads, \
|
||||
NUM_BITS, HAS_PERM>, \
|
||||
cudaFuncAttributeMaxDynamicSharedMemorySize, max_shared_mem); \
|
||||
gptq_marlin::marlin_repack_kernel<gptq_marlin::repack_threads, NUM_BITS, \
|
||||
HAS_PERM> \
|
||||
<<<blocks, gptq_marlin::repack_threads, max_shared_mem, stream>>>( \
|
||||
b_q_weight_ptr, perm_ptr, out_ptr, size_k, size_n); \
|
||||
}
|
||||
|
||||
torch::Tensor gptq_marlin_repack(torch::Tensor& b_q_weight, torch::Tensor& perm,
|
||||
int64_t size_k, int64_t size_n,
|
||||
int64_t num_bits) {
|
||||
// Verify compatibility with marlin tile of 16x64
|
||||
TORCH_CHECK(size_k % gptq_marlin::tile_k_size == 0, "size_k = ", size_k,
|
||||
" is not divisible by tile_k_size = ", gptq_marlin::tile_k_size);
|
||||
TORCH_CHECK(size_n % gptq_marlin::tile_n_size == 0, "size_n = ", size_n,
|
||||
" is not divisible by tile_n_size = ", gptq_marlin::tile_n_size);
|
||||
|
||||
TORCH_CHECK(num_bits == 4 || num_bits == 8,
|
||||
"num_bits must be 4 or 8. Got = ", num_bits);
|
||||
int const pack_factor = 32 / num_bits;
|
||||
|
||||
// Verify B
|
||||
TORCH_CHECK((size_k / pack_factor) == b_q_weight.size(0),
|
||||
"Shape mismatch: b_q_weight.size(0) = ", b_q_weight.size(0),
|
||||
", size_k = ", size_k, ", pack_factor = ", pack_factor);
|
||||
TORCH_CHECK(b_q_weight.size(1) == size_n,
|
||||
"b_q_weight.size(1) = ", b_q_weight.size(1),
|
||||
" is not size_n = ", size_n);
|
||||
|
||||
// Verify device and strides
|
||||
TORCH_CHECK(b_q_weight.device().is_cuda(), "b_q_weight is not on GPU");
|
||||
TORCH_CHECK(b_q_weight.is_contiguous(), "b_q_weight is not contiguous");
|
||||
TORCH_CHECK(b_q_weight.dtype() == at::kInt, "b_q_weight type is not kInt");
|
||||
|
||||
TORCH_CHECK(perm.device().is_cuda(), "perm is not on GPU");
|
||||
TORCH_CHECK(perm.is_contiguous(), "perm is not contiguous");
|
||||
TORCH_CHECK(perm.dtype() == at::kInt, "perm type is not at::kInt");
|
||||
|
||||
// Alloc buffers
|
||||
const at::cuda::OptionalCUDAGuard device_guard(device_of(b_q_weight));
|
||||
auto options = torch::TensorOptions()
|
||||
.dtype(b_q_weight.dtype())
|
||||
.device(b_q_weight.device());
|
||||
torch::Tensor out =
|
||||
torch::empty({size_k / gptq_marlin::tile_size,
|
||||
size_n * gptq_marlin::tile_size / pack_factor},
|
||||
options);
|
||||
|
||||
// Detect if there is act_order
|
||||
bool has_perm = perm.size(0) != 0;
|
||||
|
||||
// Get ptrs
|
||||
uint32_t const* b_q_weight_ptr =
|
||||
reinterpret_cast<uint32_t const*>(b_q_weight.data_ptr());
|
||||
uint32_t const* perm_ptr = reinterpret_cast<uint32_t const*>(perm.data_ptr());
|
||||
uint32_t* out_ptr = reinterpret_cast<uint32_t*>(out.data_ptr());
|
||||
|
||||
// Get dev info
|
||||
int dev = b_q_weight.get_device();
|
||||
cudaStream_t stream = at::cuda::getCurrentCUDAStream(dev);
|
||||
int blocks;
|
||||
cudaDeviceGetAttribute(&blocks, cudaDevAttrMultiProcessorCount, dev);
|
||||
|
||||
int max_shared_mem = 0;
|
||||
cudaDeviceGetAttribute(&max_shared_mem,
|
||||
cudaDevAttrMaxSharedMemoryPerBlockOptin, dev);
|
||||
TORCH_CHECK(max_shared_mem > 0);
|
||||
|
||||
if (false) {
|
||||
}
|
||||
CALL_IF(4, false)
|
||||
CALL_IF(4, true)
|
||||
CALL_IF(8, false)
|
||||
CALL_IF(8, true)
|
||||
else {
|
||||
TORCH_CHECK(false, "Unsupported repack config: num_bits = ", num_bits,
|
||||
", has_perm = ", has_perm);
|
||||
}
|
||||
|
||||
return out;
|
||||
}
|
||||
|
||||
#endif
|
File diff suppressed because it is too large
Load Diff
|
@ -0,0 +1,21 @@
|
|||
from setuptools import setup
|
||||
from torch.utils.cpp_extension import BuildExtension, CUDAExtension
|
||||
|
||||
extra_compile_args = []
|
||||
|
||||
setup(
|
||||
name="marlin_kernels",
|
||||
ext_modules=[
|
||||
CUDAExtension(
|
||||
name="marlin_kernels",
|
||||
sources=[
|
||||
"marlin_kernels/gptq_marlin.cu",
|
||||
"marlin_kernels/gptq_marlin_repack.cu",
|
||||
"marlin_kernels/marlin_cuda_kernel.cu",
|
||||
"marlin_kernels/ext.cpp",
|
||||
],
|
||||
extra_compile_args=extra_compile_args,
|
||||
),
|
||||
],
|
||||
cmdclass={"build_ext": BuildExtension},
|
||||
)
|
|
@ -1,6 +1,7 @@
|
|||
from typing import Optional
|
||||
import torch
|
||||
from torch.nn import functional as F
|
||||
from text_generation_server.layers.marlin import GPTQMarlinLinear
|
||||
from text_generation_server.utils.import_utils import SYSTEM
|
||||
|
||||
if SYSTEM == "rocm":
|
||||
|
@ -223,13 +224,23 @@ def get_linear(weight, bias, quantize):
|
|||
"You do not seem to have awq installed, either install it (cd server && make install-awq), or try using GPTQ `---quantize gptq` a conversion AWQ->GPTQ will happen on the fly"
|
||||
)
|
||||
elif quantize == "marlin":
|
||||
from text_generation_server.layers.marlin import MarlinLinear, MarlinWeight
|
||||
from text_generation_server.layers.marlin import (
|
||||
GPTQMarlinWeight,
|
||||
MarlinLinear,
|
||||
MarlinWeight,
|
||||
)
|
||||
|
||||
if not isinstance(weight, MarlinWeight):
|
||||
if isinstance(weight, GPTQMarlinWeight):
|
||||
linear = GPTQMarlinLinear(
|
||||
weight=weight,
|
||||
bias=bias,
|
||||
)
|
||||
elif isinstance(weight, MarlinWeight):
|
||||
linear = MarlinLinear(weight=weight, bias=bias)
|
||||
else:
|
||||
raise NotImplementedError(
|
||||
f"The passed weight is not `marlin` compatible, loader needs to be updated."
|
||||
)
|
||||
linear = MarlinLinear(B=weight.B, s=weight.s, bias=bias)
|
||||
else:
|
||||
raise NotImplementedError(f"Quantization `{quantize}` is not implemented yet.")
|
||||
return linear
|
||||
|
|
|
@ -1,13 +1,15 @@
|
|||
from dataclasses import dataclass
|
||||
from typing import Optional
|
||||
from typing import Optional, Tuple, List
|
||||
|
||||
import torch
|
||||
import torch.nn as nn
|
||||
|
||||
from text_generation_server.utils.import_utils import SYSTEM
|
||||
|
||||
try:
|
||||
import marlin
|
||||
import marlin_kernels
|
||||
except ImportError:
|
||||
marlin = None
|
||||
marlin_kernels = None
|
||||
|
||||
try:
|
||||
major, _minor = torch.cuda.get_device_capability()
|
||||
|
@ -15,9 +17,204 @@ try:
|
|||
except Exception:
|
||||
has_sm_8_0 = False
|
||||
|
||||
|
||||
GPTQ_MARLIN_BITS = [4, 8]
|
||||
GPTQ_MARLIN_GROUP_SIZES = [-1, 32, 64, 128]
|
||||
MARLIN_TILE_SIZE = 16
|
||||
|
||||
|
||||
def _check_marlin_kernels():
|
||||
if not (SYSTEM == "cuda" and has_sm_8_0):
|
||||
raise NotImplementedError(
|
||||
"Using quantized Marlin models requires a GPU with CUDA capability 8.0 or later."
|
||||
)
|
||||
|
||||
if marlin_kernels is None:
|
||||
raise NotImplementedError(
|
||||
"marlin is not installed, install it with: pip install server/marlin"
|
||||
)
|
||||
|
||||
|
||||
def _check_valid_shape(in_features: int, out_features: int):
|
||||
if (in_features % 128 != 0 or out_features % 64 != 0) and (
|
||||
in_features % 64 != 0 or out_features % 128 != 0
|
||||
):
|
||||
raise ValueError(
|
||||
f"The GPTQ Marlin kernel does not have a valid thread configuration for weight matrix with shape ({out_features}, {in_features})."
|
||||
" The shape elements must be divisible by (128, 64) or (64, 128)."
|
||||
)
|
||||
|
||||
|
||||
# https://github.com/IST-DASLab/marlin/blob/2f6d7c10e124b3c5fa29ff8d77d568bd7af3274c/marlin/__init__.py#L40C1-L68C54
|
||||
def _get_perms() -> Tuple[List[int], List[int]]:
|
||||
scale_perm = []
|
||||
for i in range(8):
|
||||
scale_perm.extend([i + 8 * j for j in range(8)])
|
||||
scale_perm_single = []
|
||||
for i in range(4):
|
||||
scale_perm_single.extend([2 * i + j for j in [0, 1, 8, 9, 16, 17, 24, 25]])
|
||||
return scale_perm, scale_perm_single
|
||||
|
||||
|
||||
_scale_perm, _scale_perm_single = _get_perms()
|
||||
|
||||
|
||||
def permute_scales(scales: torch.Tensor):
|
||||
out_features = scales.shape[1]
|
||||
if scales.shape[0] == 1:
|
||||
scales = scales.reshape((-1, len(_scale_perm_single)))[:, _scale_perm_single]
|
||||
else:
|
||||
scales = scales.reshape((-1, len(_scale_perm)))[:, _scale_perm]
|
||||
return scales.reshape((-1, out_features)).contiguous()
|
||||
|
||||
|
||||
@dataclass
|
||||
class GPTQMarlinWeight:
|
||||
"""
|
||||
Repacked GPTQ Marlin weights.
|
||||
"""
|
||||
|
||||
qweight: torch.Tensor
|
||||
scales: torch.Tensor
|
||||
g_idx: torch.Tensor
|
||||
perm: torch.Tensor
|
||||
bits: int
|
||||
is_full_k: bool
|
||||
|
||||
def __post_init__(self):
|
||||
assert self.qweight.dtype == torch.int32
|
||||
assert self.scales.dtype == torch.float16
|
||||
assert self.g_idx.dtype == torch.int32
|
||||
assert self.perm.dtype == torch.int32
|
||||
|
||||
|
||||
def repack_gptq_for_marlin(
|
||||
*,
|
||||
qweight: torch.Tensor,
|
||||
scales: torch.Tensor,
|
||||
g_idx: torch.Tensor,
|
||||
bits: int,
|
||||
desc_act: bool,
|
||||
groupsize: int,
|
||||
sym: bool,
|
||||
sharded_infeatures: bool,
|
||||
) -> GPTQMarlinWeight:
|
||||
"""Convert GPTQ weights to a layout that's compatible with GPTQ-Marlin kernels."""
|
||||
_check_marlin_kernels()
|
||||
assert marlin_kernels is not None
|
||||
|
||||
if bits not in GPTQ_MARLIN_BITS:
|
||||
supported_bits = ", ".join(str(b) for b in GPTQ_MARLIN_BITS)
|
||||
raise RuntimeError(
|
||||
f"Repacking {bits}-bit GPTQ weights as Marlin is not supported, must be one of: {supported_bits}"
|
||||
)
|
||||
|
||||
if groupsize not in GPTQ_MARLIN_GROUP_SIZES:
|
||||
supported_sizes = ", ".join(str(b) for b in GPTQ_MARLIN_GROUP_SIZES)
|
||||
raise RuntimeError(
|
||||
f"Repacking GPTQ weights with group size {groupsize} as Marlin is not supported, must be one of: {supported_sizes}"
|
||||
)
|
||||
if not sym:
|
||||
raise RuntimeError(
|
||||
"Repacking GPTQ weights with asymmetric quantization as Marlin is not supported."
|
||||
)
|
||||
|
||||
weights_per_int = 32 // bits
|
||||
in_features = qweight.shape[0] * weights_per_int
|
||||
out_features = qweight.shape[1]
|
||||
|
||||
if in_features % groupsize != 0:
|
||||
raise ValueError(
|
||||
f"Number of input features ({in_features}) not divisible by group size ({groupsize})"
|
||||
)
|
||||
|
||||
if desc_act and groupsize != -1:
|
||||
perm = torch.argsort(g_idx).to(torch.int)
|
||||
g_idx = g_idx[perm]
|
||||
else:
|
||||
perm = torch.empty(0, dtype=torch.int, device=qweight.device)
|
||||
g_idx = torch.empty(0, dtype=torch.int, device=qweight.device)
|
||||
|
||||
repacked = marlin_kernels.gptq_marlin_repack(
|
||||
qweight, perm, in_features, out_features, bits
|
||||
)
|
||||
|
||||
scales = permute_scales(scales)
|
||||
|
||||
is_full_k = not (desc_act and sharded_infeatures)
|
||||
|
||||
return GPTQMarlinWeight(
|
||||
qweight=repacked,
|
||||
scales=scales,
|
||||
g_idx=g_idx,
|
||||
perm=perm,
|
||||
bits=bits,
|
||||
is_full_k=is_full_k,
|
||||
)
|
||||
|
||||
|
||||
class GPTQMarlinLinear(nn.Module):
|
||||
"""
|
||||
Linear layer for GPTQ weights that were converted for the GPTQ-Marlin
|
||||
kernels.
|
||||
"""
|
||||
|
||||
def __init__(
|
||||
self,
|
||||
*,
|
||||
weight: GPTQMarlinWeight,
|
||||
bias: Optional[torch.Tensor],
|
||||
):
|
||||
super().__init__()
|
||||
|
||||
_check_marlin_kernels()
|
||||
assert marlin_kernels is not None
|
||||
|
||||
in_features = weight.qweight.shape[0] * MARLIN_TILE_SIZE
|
||||
out_features = weight.scales.shape[1]
|
||||
_check_valid_shape(in_features=in_features, out_features=out_features)
|
||||
|
||||
self.bits = weight.bits
|
||||
self.is_full_k = weight.is_full_k
|
||||
|
||||
self.register_buffer("qweight", weight.qweight)
|
||||
self.register_buffer("scales", weight.scales)
|
||||
self.register_buffer("g_idx", weight.g_idx)
|
||||
self.register_buffer("perm", weight.perm)
|
||||
if bias is not None:
|
||||
self.register_buffer("bias", bias)
|
||||
else:
|
||||
self.bias = None
|
||||
|
||||
self.workspace = torch.zeros(
|
||||
out_features // 64 * 16, dtype=torch.int, device=weight.qweight.device
|
||||
)
|
||||
|
||||
def forward(self, A: torch.Tensor) -> torch.Tensor:
|
||||
assert marlin_kernels is not None
|
||||
|
||||
A_flat = A.view(-1, A.shape[-1])
|
||||
C = marlin_kernels.gptq_marlin_gemm(
|
||||
A_flat,
|
||||
self.qweight,
|
||||
self.scales,
|
||||
self.g_idx,
|
||||
self.perm,
|
||||
self.workspace,
|
||||
self.bits,
|
||||
A_flat.shape[0],
|
||||
self.scales.shape[1],
|
||||
A_flat.shape[1],
|
||||
self.is_full_k,
|
||||
)
|
||||
C = C.reshape(A.shape[:-1] + (self.scales.shape[1],))
|
||||
|
||||
if self.bias is not None:
|
||||
C += self.bias
|
||||
|
||||
return C
|
||||
|
||||
|
||||
@dataclass
|
||||
class MarlinWeight:
|
||||
"""
|
||||
|
@ -31,28 +228,20 @@ class MarlinWeight:
|
|||
B: torch.Tensor
|
||||
s: torch.Tensor
|
||||
|
||||
def __post_init__(self):
|
||||
assert self.B.dtype == torch.int32
|
||||
assert self.s.dtype == torch.float16
|
||||
|
||||
|
||||
class MarlinLinear(nn.Module):
|
||||
def __init__(
|
||||
self, *, B: torch.Tensor, s: torch.Tensor, bias: Optional[torch.Tensor]
|
||||
):
|
||||
def __init__(self, *, weight: MarlinWeight, bias: Optional[torch.Tensor]):
|
||||
super().__init__()
|
||||
|
||||
if not has_sm_8_0:
|
||||
raise NotImplementedError(
|
||||
"Using quantized marlin models requires CUDA capability 8.0 or later"
|
||||
)
|
||||
_check_marlin_kernels()
|
||||
assert marlin_kernels is not None
|
||||
|
||||
if marlin is None:
|
||||
raise NotImplementedError(
|
||||
"You do not seem to have marlin installed, either install it (cd server && make install-marlin)"
|
||||
)
|
||||
|
||||
assert B.dtype == torch.int32
|
||||
assert s.dtype == torch.float16
|
||||
|
||||
in_features = B.shape[0] * MARLIN_TILE_SIZE
|
||||
out_features = s.shape[1]
|
||||
in_features = weight.B.shape[0] * MARLIN_TILE_SIZE
|
||||
out_features = weight.s.shape[1]
|
||||
assert (
|
||||
in_features % 128 == 0
|
||||
), f"Number of input features ({in_features}) not divisable by 128"
|
||||
|
@ -60,35 +249,36 @@ class MarlinLinear(nn.Module):
|
|||
out_features % 256 == 0
|
||||
), f"Number of output features ({out_features}) not divisable by 256"
|
||||
|
||||
group_size = -1 if s.shape[0] == 1 else in_features // s.shape[0]
|
||||
assert group_size in {
|
||||
groupsize = -1 if weight.s.shape[0] == 1 else in_features // weight.s.shape[0]
|
||||
assert groupsize in {
|
||||
-1,
|
||||
128,
|
||||
}, f"Group size must be -1 or 128, was {group_size}"
|
||||
}, f"Group size must be -1 or 128, was {groupsize}"
|
||||
|
||||
self.register_buffer("B", B)
|
||||
self.register_buffer("s", s)
|
||||
self.register_buffer("B", weight.B)
|
||||
self.register_buffer("s", weight.s)
|
||||
if bias is not None:
|
||||
self.register_buffer("bias", bias)
|
||||
else:
|
||||
self.bias = None
|
||||
|
||||
self.workspace = torch.zeros(
|
||||
out_features // 128 * 16, dtype=torch.int, device=B.device
|
||||
out_features // 64 * 16, dtype=torch.int, device=weight.B.device
|
||||
)
|
||||
|
||||
def forward(self, A: torch.Tensor) -> torch.Tensor:
|
||||
assert marlin is not None
|
||||
C = torch.empty(
|
||||
A.shape[:-1] + (self.s.shape[1],), dtype=A.dtype, device=A.device
|
||||
)
|
||||
marlin.mul(
|
||||
A.view((-1, A.shape[-1])),
|
||||
assert marlin_kernels is not None
|
||||
|
||||
C = marlin_kernels.marlin_gemm(
|
||||
A.view(-1, A.shape[-1]),
|
||||
self.B,
|
||||
C.view((-1, C.shape[-1])),
|
||||
self.s,
|
||||
self.workspace,
|
||||
A.shape[0],
|
||||
self.s.shape[1],
|
||||
A.shape[1],
|
||||
)
|
||||
C = C.reshape(A.shape[:-1] + (self.s.shape[1],))
|
||||
|
||||
if self.bias is not None:
|
||||
C += self.bias
|
||||
|
|
|
@ -267,19 +267,21 @@ class SuRotaryEmbedding(PositionRotaryEmbedding):
|
|||
or self._cos_cached.dtype != dtype
|
||||
):
|
||||
self._seq_len_cached = seqlen
|
||||
if seqlen > self.original_max_position_embeddings:
|
||||
inv_freq = self.long_inv_freq
|
||||
else:
|
||||
inv_freq = self.short_inv_freq
|
||||
t = torch.arange(seqlen, device=device, dtype=inv_freq.dtype)
|
||||
if self.scaling_factor is not None:
|
||||
t /= self.scaling_factor
|
||||
# Don't do einsum, it converts fp32 to fp16
|
||||
# freqs = torch.einsum("i,j->ij", t, self.inv_freq)
|
||||
|
||||
freqs = torch.outer(t, inv_freq.to(device=t.device))
|
||||
self._cos_cached = torch.cos(freqs).to(dtype)
|
||||
self._sin_cached = torch.sin(freqs).to(dtype)
|
||||
t = torch.arange(seqlen, device=device, dtype=self.short_inv_freq.dtype)
|
||||
short_freqs = torch.outer(
|
||||
t[: self.original_max_position_embeddings],
|
||||
self.short_inv_freq.to(device=t.device),
|
||||
)
|
||||
long_freqs = torch.outer(
|
||||
t[self.original_max_position_embeddings :],
|
||||
self.long_inv_freq.to(device=t.device),
|
||||
)
|
||||
|
||||
freqs = torch.cat([short_freqs, long_freqs])
|
||||
|
||||
self._cos_cached = (torch.cos(freqs) * self.scaling_factor).to(dtype)
|
||||
self._sin_cached = (torch.sin(freqs) * self.scaling_factor).to(dtype)
|
||||
|
||||
|
||||
class DynamicPositionRotaryEmbedding(PositionRotaryEmbedding):
|
||||
|
|
|
@ -196,7 +196,7 @@ class ModelType(enum.Enum):
|
|||
QWEN2 = {
|
||||
"type": "qwen2",
|
||||
"name": "Qwen 2",
|
||||
"url": "https://huggingface.co/bigcode/starcoder2-15b-instruct-v0.1",
|
||||
"url": "https://huggingface.co/collections/Qwen/qwen2-6659360b33528ced941e557f",
|
||||
}
|
||||
OPT = {
|
||||
"type": "opt",
|
||||
|
|
|
@ -83,7 +83,7 @@ class BLOOMSharded(CausalLM):
|
|||
process_group=self.process_group,
|
||||
prefix="transformer",
|
||||
)
|
||||
if config.quantize == "gptq":
|
||||
if config.quantize in ["gptq", "marlin"]:
|
||||
weights._set_gptq_params(model_id, revision)
|
||||
|
||||
model = BloomForCausalLM(config, weights)
|
||||
|
|
|
@ -166,7 +166,7 @@ def _load_gqa(config, prefix: str, weights):
|
|||
dim=0,
|
||||
)
|
||||
|
||||
if config.quantize not in ["gptq", "awq"]:
|
||||
if config.quantize not in ["gptq", "awq", "marlin"]:
|
||||
weight = weight.to(dtype=weights.dtype).to(device=weights.device)
|
||||
|
||||
head_size = config.hidden_size // config.num_attention_heads
|
||||
|
|
|
@ -81,16 +81,11 @@ def _load_multi_mqa_gptq(
|
|||
qzeros = torch.cat([q_tensor, kv_tensor], dim=1)
|
||||
qzeros = qzeros.to(device=weights.device)
|
||||
|
||||
(
|
||||
bits,
|
||||
groupsize,
|
||||
_,
|
||||
quant_method,
|
||||
) = weights._get_gptq_params()
|
||||
if quant_method == "gptq":
|
||||
gptq_params = weights._get_gptq_params()
|
||||
if gptq_params.quant_method == "gptq":
|
||||
g_idx = weights.get_tensor(f"{prefix}.c_attn.g_idx")
|
||||
g_idx = g_idx.to(device=weights.device)
|
||||
elif quant_method == "awq":
|
||||
elif gptq_params.quant_method == "awq":
|
||||
g_idx = None
|
||||
from text_generation_server.layers.awq.conversion_utils import (
|
||||
fast_awq_to_gptq,
|
||||
|
@ -105,8 +100,8 @@ def _load_multi_mqa_gptq(
|
|||
qzeros=qzeros,
|
||||
scales=scales,
|
||||
g_idx=g_idx,
|
||||
bits=bits,
|
||||
groupsize=groupsize,
|
||||
bits=gptq_params.bits,
|
||||
groupsize=gptq_params.groupsize,
|
||||
use_exllama=HAS_EXLLAMA,
|
||||
)
|
||||
|
||||
|
|
|
@ -130,7 +130,7 @@ def _load_gqa(config, prefix: str, weights):
|
|||
dim=0,
|
||||
)
|
||||
|
||||
if config.quantize not in ["gptq", "awq"]:
|
||||
if config.quantize not in ["gptq", "awq", "marlin"]:
|
||||
weight = weight.to(dtype=weights.dtype).to(device=weights.device)
|
||||
|
||||
head_size = config.hidden_size // config.num_attention_heads
|
||||
|
|
|
@ -792,7 +792,7 @@ class OPTForCausalLM(OPTPreTrainedModel):
|
|||
return_dict=return_dict,
|
||||
)
|
||||
|
||||
logits, speculative_logits = self.lm_head(outputs)
|
||||
logits, speculative_logits = self.lm_head(outputs.last_hidden_state)
|
||||
|
||||
loss = None
|
||||
|
||||
|
|
|
@ -55,7 +55,7 @@ class FlashCohere(FlashCausalLM):
|
|||
|
||||
filenames = weight_files(model_id, revision=revision, extension=".safetensors")
|
||||
weights = Weights(filenames, device, dtype, process_group=self.process_group)
|
||||
if config.quantize in ["gptq", "awq"]:
|
||||
if config.quantize in ["gptq", "awq", "marlin"]:
|
||||
weights._set_gptq_params(model_id, revision)
|
||||
|
||||
model = FlashCohereForCausalLM(config, weights)
|
||||
|
|
|
@ -80,7 +80,7 @@ class FlashDbrx(FlashCausalLM):
|
|||
|
||||
filenames = weight_files(model_id, revision=revision, extension=".safetensors")
|
||||
weights = Weights(filenames, device, dtype, process_group=self.process_group)
|
||||
if config.quantize in ["gptq", "awq"]:
|
||||
if config.quantize in ["gptq", "awq", "marlin"]:
|
||||
weights._set_gptq_params(model_id, revision)
|
||||
|
||||
model = FlashDbrxForCausalLM(config, weights)
|
||||
|
|
|
@ -53,7 +53,7 @@ class FlashGemma(FlashCausalLM):
|
|||
|
||||
filenames = weight_files(model_id, revision=revision, extension=".safetensors")
|
||||
weights = Weights(filenames, device, dtype, process_group=self.process_group)
|
||||
if config.quantize in ["gptq", "awq"]:
|
||||
if config.quantize in ["gptq", "awq", "marlin"]:
|
||||
weights._set_gptq_params(model_id, revision)
|
||||
|
||||
# TODO hardcoded
|
||||
|
|
|
@ -67,7 +67,7 @@ class FlashLlama(FlashCausalLM):
|
|||
|
||||
filenames = weight_files(model_id, revision=revision, extension=".safetensors")
|
||||
weights = Weights(filenames, device, dtype, process_group=self.process_group)
|
||||
if config.quantize in ["gptq", "awq", "exl2"]:
|
||||
if config.quantize in ["awq", "exl2", "gptq", "marlin"]:
|
||||
weights._set_gptq_params(model_id, revision)
|
||||
|
||||
prefix = ""
|
||||
|
|
|
@ -68,7 +68,7 @@ class BaseFlashMistral(FlashCausalLM):
|
|||
|
||||
filenames = weight_files(model_id, revision=revision, extension=".safetensors")
|
||||
weights = Weights(filenames, device, dtype, process_group=self.process_group)
|
||||
if config.quantize in ["gptq", "awq"]:
|
||||
if config.quantize in ["gptq", "awq", "marlin"]:
|
||||
weights._set_gptq_params(model_id, revision)
|
||||
|
||||
prefix = ""
|
||||
|
|
|
@ -58,7 +58,7 @@ class FlashNeoXSharded(FlashCausalLM):
|
|||
weights = Weights(
|
||||
filenames, device=device, dtype=dtype, process_group=self.process_group
|
||||
)
|
||||
if config.quantize == "gptq":
|
||||
if config.quantize in ["gptq", "marlin"]:
|
||||
weights._set_gptq_params(model_id, revision)
|
||||
|
||||
model = FlashGPTNeoXForCausalLM(config, weights)
|
||||
|
|
|
@ -8,7 +8,6 @@ from typing import Optional
|
|||
from text_generation_server.models import FlashCausalLM
|
||||
from text_generation_server.models.custom_modeling.flash_phi_modeling import (
|
||||
FlashPhiForCausalLM,
|
||||
PhiConfig,
|
||||
)
|
||||
from text_generation_server.utils import (
|
||||
initialize_torch_distributed,
|
||||
|
@ -44,7 +43,7 @@ class FlashPhi(FlashCausalLM):
|
|||
trust_remote_code=trust_remote_code,
|
||||
)
|
||||
|
||||
config = PhiConfig.from_pretrained(
|
||||
config = AutoConfig.from_pretrained(
|
||||
model_id, revision=revision, trust_remote_code=trust_remote_code
|
||||
)
|
||||
config.quantize = quantize
|
||||
|
@ -54,7 +53,7 @@ class FlashPhi(FlashCausalLM):
|
|||
|
||||
filenames = weight_files(model_id, revision=revision, extension=".safetensors")
|
||||
weights = Weights(filenames, device, dtype, process_group=self.process_group)
|
||||
if config.quantize in ["gptq", "awq"]:
|
||||
if config.quantize in ["gptq", "awq", "marlin"]:
|
||||
weights._set_gptq_params(model_id, revision)
|
||||
|
||||
model = FlashPhiForCausalLM(config, weights)
|
||||
|
|
|
@ -62,7 +62,7 @@ class FlashQwen2(BaseFlashMistral):
|
|||
|
||||
filenames = weight_files(model_id, revision=revision, extension=".safetensors")
|
||||
weights = Weights(filenames, device, dtype, process_group=self.process_group)
|
||||
if config.quantize in ["gptq", "awq"]:
|
||||
if config.quantize in ["gptq", "awq", "marlin"]:
|
||||
weights._set_gptq_params(model_id, revision)
|
||||
|
||||
model = Qwen2ForCausalLM(config, weights)
|
||||
|
|
|
@ -67,7 +67,7 @@ class FlashRWSharded(FlashCausalLM):
|
|||
|
||||
config.quantize = quantize
|
||||
config.speculator = speculator
|
||||
if config.quantize == "gptq":
|
||||
if config.quantize in ["gptq", "marlin"]:
|
||||
weights._set_gptq_params(model_id, revision)
|
||||
|
||||
model = FlashRWForCausalLM(config, weights)
|
||||
|
|
|
@ -69,7 +69,7 @@ class FlashSantacoderSharded(FlashCausalLM):
|
|||
process_group=self.process_group,
|
||||
aliases={"transformer.wte.weight": ["lm_head.weight"]},
|
||||
)
|
||||
if config.quantize == "gptq":
|
||||
if config.quantize in ["gptq", "marlin"]:
|
||||
weights._set_gptq_params(model_id, revision)
|
||||
|
||||
model = FlashSantacoderForCausalLM(config, weights)
|
||||
|
|
|
@ -61,7 +61,7 @@ class FlashStarcoder2(BaseFlashMistral):
|
|||
|
||||
filenames = weight_files(model_id, revision=revision, extension=".safetensors")
|
||||
weights = Weights(filenames, device, dtype, process_group=self.process_group)
|
||||
if config.quantize in ["gptq", "awq"]:
|
||||
if config.quantize in ["gptq", "awq", "marlin"]:
|
||||
weights._set_gptq_params(model_id, revision)
|
||||
|
||||
model = FlashStarcoder2ForCausalLM(config, weights)
|
||||
|
|
|
@ -205,7 +205,7 @@ class GalacticaSharded(CausalLM):
|
|||
weights = Weights(
|
||||
filenames, device=device, dtype=dtype, process_group=self.process_group
|
||||
)
|
||||
if config.quantize == "gptq":
|
||||
if config.quantize in ["gptq", "marlin"]:
|
||||
weights._set_gptq_params(model_id, revision)
|
||||
|
||||
model = OPTForCausalLM(config, weights)
|
||||
|
|
|
@ -58,7 +58,7 @@ class GPTNeoxSharded(CausalLM):
|
|||
weights = Weights(
|
||||
filenames, device=device, dtype=dtype, process_group=self.process_group
|
||||
)
|
||||
if config.quantize == "gptq":
|
||||
if config.quantize in ["gptq", "marlin"]:
|
||||
weights._set_gptq_params(model_id, revision)
|
||||
|
||||
model = GPTNeoxForCausalLM(config, weights)
|
||||
|
@ -85,5 +85,4 @@ class GPTNeoxSharded(CausalLM):
|
|||
use_cache=True,
|
||||
)
|
||||
|
||||
logits = outputs.logits
|
||||
return logits, speculative_logits, outputs.past_key_values
|
||||
return outputs.logits, speculative_logits, outputs.past_key_values
|
||||
|
|
|
@ -82,7 +82,7 @@ class MPTSharded(CausalLM):
|
|||
|
||||
filenames = weight_files(model_id, revision=revision, extension=".safetensors")
|
||||
weights = Weights(filenames, device, dtype, process_group=self.process_group)
|
||||
if config.quantize == "gptq":
|
||||
if config.quantize in ["gptq", "marlin"]:
|
||||
weights._set_gptq_params(model_id, revision)
|
||||
|
||||
config.quantize = quantize
|
||||
|
|
|
@ -56,7 +56,7 @@ class OPTSharded(CausalLM):
|
|||
weights = Weights(
|
||||
filenames, device=device, dtype=dtype, process_group=self.process_group
|
||||
)
|
||||
if config.quantize == "gptq":
|
||||
if config.quantize in ["gptq", "marlin"]:
|
||||
weights._set_gptq_params(model_id, revision)
|
||||
|
||||
model = OPTForCausalLM(config, weights)
|
||||
|
@ -75,11 +75,11 @@ class OPTSharded(CausalLM):
|
|||
def forward(
|
||||
self, input_ids, attention_mask, position_ids, past_key_values: Optional = None
|
||||
):
|
||||
outputs = self.model.forward(
|
||||
outputs, speculative_logits = self.model.forward(
|
||||
input_ids=input_ids,
|
||||
attention_mask=attention_mask,
|
||||
past_key_values=past_key_values,
|
||||
use_cache=True,
|
||||
)
|
||||
|
||||
return outputs.logits, outputs.past_key_values
|
||||
return outputs.logits, speculative_logits, outputs.past_key_values
|
||||
|
|
|
@ -71,11 +71,13 @@ class RW(CausalLM):
|
|||
|
||||
def forward(
|
||||
self, input_ids, attention_mask, position_ids, past_key_values: Optional = None
|
||||
) -> Tuple[torch.Tensor, List[Tuple[torch.Tensor, torch.Tensor]]]:
|
||||
):
|
||||
# Model Forward
|
||||
outputs = self.model.forward(
|
||||
outputs, speculative_logits = self.model.forward(
|
||||
input_ids=input_ids,
|
||||
attention_mask=attention_mask,
|
||||
past_key_values=past_key_values,
|
||||
use_cache=True,
|
||||
)
|
||||
return outputs.logits, outputs.past_key_values
|
||||
|
||||
return outputs.logits, speculative_logits, outputs.past_key_values
|
||||
|
|
|
@ -53,7 +53,9 @@ def image_text_replacement(image_input, config, image_id) -> str:
|
|||
num_features = get_number_of_features(height, width, config)
|
||||
from loguru import logger
|
||||
|
||||
logger.info(f"Found {num_features} in image of resolution {height}x{width}")
|
||||
logger.info(
|
||||
f"Found {num_features} features in image of resolution {height}x{width}"
|
||||
)
|
||||
return "<image>" * num_features
|
||||
|
||||
elif config.model_type == "paligemma":
|
||||
|
@ -141,23 +143,41 @@ class VlmCausalLMBatch(FlashCausalLMBatch):
|
|||
def batch_tokenized_inputs(
|
||||
cls, requests: Iterable[generate_pb2.Request], tokenizer, processor, config
|
||||
):
|
||||
# Process images first. We need all of them so that the processor
|
||||
# can make the image splits the same size. And we need the final
|
||||
# sizes to insert correct number of image tokens.
|
||||
images = []
|
||||
for r in requests:
|
||||
for chunk in r.input_chunks.chunks:
|
||||
chunk_type = chunk.WhichOneof("chunk")
|
||||
if chunk_type == "text":
|
||||
pass
|
||||
elif chunk_type == "image":
|
||||
image = Image.open(BytesIO(chunk.image.data))
|
||||
if config.model_type == "llava_next":
|
||||
images.append(image)
|
||||
else:
|
||||
images.append([image])
|
||||
else:
|
||||
raise RuntimeError(f"Invalid chunk type {chunk_type}")
|
||||
|
||||
if images:
|
||||
image_inputs = processor.image_processor(images, return_tensors="pt")
|
||||
else:
|
||||
image_inputs = None
|
||||
|
||||
batch_inputs = []
|
||||
image_inputs = []
|
||||
max_truncation = 0
|
||||
image_id = 0
|
||||
for r in requests:
|
||||
full_text = ""
|
||||
image_id = 0
|
||||
for chunk in r.input_chunks.chunks:
|
||||
chunk_type = chunk.WhichOneof("chunk")
|
||||
if chunk_type == "text":
|
||||
full_text += chunk.text
|
||||
elif chunk_type == "image":
|
||||
image = Image.open(BytesIO(chunk.image.data))
|
||||
image_input = processor.image_processor(image, return_tensors="pt")
|
||||
full_text += image_text_replacement(image_input, config, image_id)
|
||||
image_inputs.append(image_input)
|
||||
else:
|
||||
raise RuntimeError(f"Invalid chunk type {chunk_type}")
|
||||
full_text += image_text_replacement(image_inputs, config, image_id)
|
||||
image_id += 1
|
||||
|
||||
batch_inputs.append(full_text)
|
||||
max_truncation = max(max_truncation, r.truncate)
|
||||
|
@ -168,24 +188,7 @@ class VlmCausalLMBatch(FlashCausalLMBatch):
|
|||
max_length=max_truncation,
|
||||
add_special_tokens=not config.model_type == "paligemma",
|
||||
)["input_ids"]
|
||||
if image_inputs:
|
||||
image_input = image_inputs[0]
|
||||
new_image_inputs = {
|
||||
"pixel_values": torch.cat(
|
||||
[img["pixel_values"] for img in image_inputs], dim=0
|
||||
),
|
||||
}
|
||||
if "pixel_attention_mask" in image_input:
|
||||
new_image_inputs["pixel_attention_mask"] = torch.cat(
|
||||
[img["pixel_attention_mask"] for img in image_inputs], dim=0
|
||||
)
|
||||
if "image_sizes" in image_input:
|
||||
new_image_inputs["image_sizes"] = torch.cat(
|
||||
[img["image_sizes"] for img in image_inputs], dim=0
|
||||
)
|
||||
image_inputs = new_image_inputs
|
||||
else:
|
||||
image_inputs = None
|
||||
|
||||
return batch_tokenized_inputs, image_inputs
|
||||
|
||||
@classmethod
|
||||
|
|
|
@ -246,7 +246,11 @@ def serve(
|
|||
interceptors=[
|
||||
ExceptionInterceptor(),
|
||||
UDSOpenTelemetryAioServerInterceptor(),
|
||||
]
|
||||
],
|
||||
options=[
|
||||
# Set the maximum possible message length: i32::MAX
|
||||
("grpc.max_receive_message_length", (1 << 31) - 1)
|
||||
],
|
||||
)
|
||||
generate_pb2_grpc.add_TextGenerationServiceServicer_to_server(
|
||||
TextGenerationService(model, Cache(), quantize, server_urls), server
|
||||
|
|
|
@ -1,4 +1,5 @@
|
|||
import os
|
||||
from dataclasses import dataclass
|
||||
from pathlib import Path
|
||||
from typing import Dict, List, Optional, Tuple, Union
|
||||
from safetensors import safe_open, SafetensorError
|
||||
|
@ -9,6 +10,15 @@ import json
|
|||
from text_generation_server.utils.log import log_once
|
||||
|
||||
|
||||
@dataclass
|
||||
class _GPTQParams:
|
||||
bits: int
|
||||
groupsize: int
|
||||
desc_act: bool
|
||||
quant_method: str
|
||||
sym: bool
|
||||
|
||||
|
||||
class Weights:
|
||||
def __init__(
|
||||
self,
|
||||
|
@ -181,15 +191,15 @@ class Weights:
|
|||
f"Cannot load `{quantize}` weight, make sure the model is already quantized."
|
||||
)
|
||||
|
||||
bits, groupsize, _, quant_method = self._get_gptq_params()
|
||||
gptq_params = self._get_gptq_params()
|
||||
|
||||
qzeros = self._get_qweight(f"{prefix}.qzeros", block_sizes)
|
||||
scales = self._get_qweight(f"{prefix}.scales", block_sizes)
|
||||
scales = scales.to(dtype=self.dtype)
|
||||
|
||||
if quantize == "gptq" and quant_method == "gptq":
|
||||
if quantize == "gptq" and gptq_params.quant_method == "gptq":
|
||||
g_idx = self.get_tensor(f"{prefix}.g_idx")
|
||||
elif quantize == "gptq" and quant_method == "awq":
|
||||
elif quantize == "gptq" and gptq_params.quant_method == "awq":
|
||||
log_once(
|
||||
logger.info, "Converting AWQ model to Exllama/GPTQ packing format."
|
||||
)
|
||||
|
@ -199,8 +209,11 @@ class Weights:
|
|||
|
||||
qweight, qzeros = fast_awq_to_gptq(qweight, qzeros)
|
||||
g_idx = (
|
||||
torch.arange(qweight.shape[0] * (32 // bits), device=qweight.device)
|
||||
// groupsize
|
||||
torch.arange(
|
||||
qweight.shape[0] * (32 // gptq_params.bits),
|
||||
device=qweight.device,
|
||||
)
|
||||
// gptq_params.groupsize
|
||||
).to(dtype=torch.int32)
|
||||
else:
|
||||
g_idx = None
|
||||
|
@ -210,16 +223,43 @@ class Weights:
|
|||
qzeros=qzeros,
|
||||
scales=scales,
|
||||
g_idx=g_idx,
|
||||
bits=bits,
|
||||
groupsize=groupsize,
|
||||
bits=gptq_params.bits,
|
||||
groupsize=gptq_params.groupsize,
|
||||
use_exllama=False,
|
||||
)
|
||||
elif quantize == "marlin":
|
||||
from text_generation_server.layers.marlin import MarlinWeight
|
||||
from text_generation_server.layers.marlin import (
|
||||
MarlinWeight,
|
||||
repack_gptq_for_marlin,
|
||||
)
|
||||
|
||||
B = self._get_qweight(f"{prefix}.B", block_sizes)
|
||||
s = self._get_qweight(f"{prefix}.s", block_sizes)
|
||||
weight = MarlinWeight(B=B, s=s)
|
||||
quant_method = getattr(self, "quant_method", "marlin")
|
||||
if quant_method == "gptq":
|
||||
gptq_params = self._get_gptq_params()
|
||||
try:
|
||||
qweight = self._get_qweight(f"{prefix}.qweight", block_sizes)
|
||||
except RuntimeError:
|
||||
raise RuntimeError(
|
||||
f"Cannot load `{quantize}` weight for GPTQ -> Marlin repacking, make sure the model is already quantized"
|
||||
)
|
||||
|
||||
scales = self._get_qweight(f"{prefix}.scales", block_sizes)
|
||||
g_idx = self.get_tensor(f"{prefix}.g_idx")
|
||||
weight = repack_gptq_for_marlin(
|
||||
qweight=qweight,
|
||||
scales=scales,
|
||||
g_idx=g_idx,
|
||||
bits=gptq_params.bits,
|
||||
desc_act=gptq_params.desc_act,
|
||||
groupsize=gptq_params.groupsize,
|
||||
sym=gptq_params.sym,
|
||||
sharded_infeatures=False,
|
||||
)
|
||||
|
||||
else:
|
||||
B = self._get_qweight(f"{prefix}.B", block_sizes)
|
||||
s = self._get_qweight(f"{prefix}.s", block_sizes)
|
||||
weight = MarlinWeight(B=B, s=s)
|
||||
else:
|
||||
slice_ = self._get_slice(f"{prefix}.weight")
|
||||
total_size = slice_.get_shape()[0]
|
||||
|
@ -295,20 +335,23 @@ class Weights:
|
|||
[self.get_sharded(f"{p}.scales", dim=1) for p in prefixes], dim=1
|
||||
)
|
||||
|
||||
bits, groupsize, desc_act, quant_method = self._get_gptq_params()
|
||||
gptq_params = self._get_gptq_params()
|
||||
|
||||
from text_generation_server.layers.gptq import HAS_EXLLAMA
|
||||
|
||||
use_exllama = (
|
||||
bits == 4 and HAS_EXLLAMA and quantize == "gptq" and not desc_act
|
||||
gptq_params.bits == 4
|
||||
and HAS_EXLLAMA
|
||||
and quantize == "gptq"
|
||||
and not gptq_params.desc_act
|
||||
)
|
||||
|
||||
if quantize == "gptq" and quant_method == "gptq":
|
||||
if quantize == "gptq" and gptq_params.quant_method == "gptq":
|
||||
w = [self.get_tensor(f"{p}.g_idx") for p in prefixes]
|
||||
for w2 in w[1:]:
|
||||
torch.testing.assert_close(w2, w[0])
|
||||
g_idx = w[0]
|
||||
elif quantize == "gptq" and quant_method == "awq":
|
||||
elif quantize == "gptq" and gptq_params.quant_method == "awq":
|
||||
log_once(
|
||||
logger.info, "Converting AWQ model to Exllama/GPTQ packing format."
|
||||
)
|
||||
|
@ -322,9 +365,10 @@ class Weights:
|
|||
else:
|
||||
g_idx = (
|
||||
torch.arange(
|
||||
qweight.shape[0] * (32 // bits), device=qweight.device
|
||||
qweight.shape[0] * (32 // gptq_params.bits),
|
||||
device=qweight.device,
|
||||
)
|
||||
// groupsize
|
||||
// gptq_params.groupsize
|
||||
).to(dtype=torch.int32)
|
||||
else:
|
||||
g_idx = None
|
||||
|
@ -334,24 +378,62 @@ class Weights:
|
|||
qzeros=qzeros,
|
||||
scales=scales,
|
||||
g_idx=g_idx,
|
||||
bits=bits,
|
||||
groupsize=groupsize,
|
||||
bits=gptq_params.bits,
|
||||
groupsize=gptq_params.groupsize,
|
||||
use_exllama=use_exllama,
|
||||
)
|
||||
elif quantize == "marlin":
|
||||
from text_generation_server.layers.marlin import MarlinWeight
|
||||
from text_generation_server.layers.gptq import GPTQWeight
|
||||
from text_generation_server.layers.marlin import (
|
||||
MarlinWeight,
|
||||
repack_gptq_for_marlin,
|
||||
)
|
||||
|
||||
try:
|
||||
B = torch.cat(
|
||||
[self.get_sharded(f"{p}.B", dim=1) for p in prefixes], dim=1
|
||||
)
|
||||
except RuntimeError:
|
||||
raise RuntimeError(
|
||||
f"Cannot load `{quantize}` weight, make sure the model is already quantized"
|
||||
)
|
||||
s = torch.cat([self.get_sharded(f"{p}.s", dim=1) for p in prefixes], dim=1)
|
||||
quant_method = getattr(self, "quant_method", "marlin")
|
||||
if quant_method == "gptq":
|
||||
gptq_params = self._get_gptq_params()
|
||||
try:
|
||||
qweight = torch.cat(
|
||||
[self.get_sharded(f"{p}.qweight", dim=1) for p in prefixes],
|
||||
dim=1,
|
||||
)
|
||||
except RuntimeError:
|
||||
raise RuntimeError(
|
||||
f"Cannot load `{quantize}` weight for GPTQ -> Marlin repacking, make sure the model is already quantized"
|
||||
)
|
||||
|
||||
weight = MarlinWeight(B=B, s=s)
|
||||
scales = torch.cat(
|
||||
[self.get_sharded(f"{p}.scales", dim=1) for p in prefixes], dim=1
|
||||
)
|
||||
w = [self.get_tensor(f"{p}.g_idx") for p in prefixes]
|
||||
for w2 in w[1:]:
|
||||
torch.testing.assert_close(w2, w[0])
|
||||
g_idx = w[0]
|
||||
|
||||
weight = repack_gptq_for_marlin(
|
||||
qweight=qweight,
|
||||
scales=scales,
|
||||
g_idx=g_idx,
|
||||
bits=gptq_params.bits,
|
||||
desc_act=gptq_params.desc_act,
|
||||
groupsize=gptq_params.groupsize,
|
||||
sym=gptq_params.sym,
|
||||
sharded_infeatures=False,
|
||||
)
|
||||
else:
|
||||
try:
|
||||
B = torch.cat(
|
||||
[self.get_sharded(f"{p}.B", dim=1) for p in prefixes], dim=1
|
||||
)
|
||||
except RuntimeError:
|
||||
raise RuntimeError(
|
||||
f"Cannot load `{quantize}` weight, make sure the model is already quantized"
|
||||
)
|
||||
s = torch.cat(
|
||||
[self.get_sharded(f"{p}.s", dim=1) for p in prefixes], dim=1
|
||||
)
|
||||
|
||||
weight = MarlinWeight(B=B, s=s)
|
||||
|
||||
else:
|
||||
w = [self.get_sharded(f"{p}.weight", dim=0) for p in prefixes]
|
||||
|
@ -401,12 +483,12 @@ class Weights:
|
|||
|
||||
elif quantize == "gptq":
|
||||
use_exllama = True
|
||||
bits, groupsize, desc_act, quant_method = self._get_gptq_params()
|
||||
gptq_params = self._get_gptq_params()
|
||||
|
||||
if bits != 4:
|
||||
if gptq_params.bits != 4:
|
||||
use_exllama = False
|
||||
|
||||
if desc_act:
|
||||
if gptq_params.desc_act:
|
||||
log_once(logger.warning, "Disabling exllama because desc_act=True")
|
||||
use_exllama = False
|
||||
|
||||
|
@ -417,9 +499,9 @@ class Weights:
|
|||
"Cannot load `gptq` weight, make sure the model is already quantized, or quantize it with `text-generation-server quantize ORIGINAL_MODEL_ID NEW_MODEL_ID`"
|
||||
)
|
||||
|
||||
if quant_method == "gptq":
|
||||
if gptq_params.quant_method == "gptq":
|
||||
g_idx = self.get_sharded(f"{prefix}.g_idx", dim=0)
|
||||
elif quant_method == "awq":
|
||||
elif gptq_params.quant_method == "awq":
|
||||
g_idx = None
|
||||
|
||||
if self.process_group.size() > 1:
|
||||
|
@ -428,7 +510,10 @@ class Weights:
|
|||
not torch.equal(
|
||||
g_idx.cpu(),
|
||||
torch.tensor(
|
||||
[i // groupsize for i in range(g_idx.shape[0])],
|
||||
[
|
||||
i // gptq_params.groupsize
|
||||
for i in range(g_idx.shape[0])
|
||||
],
|
||||
dtype=torch.int32,
|
||||
),
|
||||
)
|
||||
|
@ -455,7 +540,7 @@ class Weights:
|
|||
else:
|
||||
log_once(logger.info, f"Using exllama kernels v{HAS_EXLLAMA}")
|
||||
|
||||
if use_exllama and groupsize != -1:
|
||||
if use_exllama and gptq_params.groupsize != -1:
|
||||
qzeros = self.get_sharded(f"{prefix}.qzeros", dim=0)
|
||||
scales = self.get_sharded(f"{prefix}.scales", dim=0)
|
||||
else:
|
||||
|
@ -465,7 +550,7 @@ class Weights:
|
|||
if use_exllama and g_idx is not None:
|
||||
g_idx = g_idx - g_idx[0]
|
||||
|
||||
if quant_method == "awq":
|
||||
if gptq_params.quant_method == "awq":
|
||||
log_once(
|
||||
logger.info, "Converting AWQ model to Exllama/GPTQ packing format."
|
||||
)
|
||||
|
@ -479,9 +564,10 @@ class Weights:
|
|||
else:
|
||||
g_idx = (
|
||||
torch.arange(
|
||||
qweight.shape[0] * (32 // bits), device=qweight.device
|
||||
qweight.shape[0] * (32 // gptq_params.bits),
|
||||
device=qweight.device,
|
||||
)
|
||||
// groupsize
|
||||
// gptq_params.groupsize
|
||||
).to(dtype=torch.int32)
|
||||
|
||||
weight = GPTQWeight(
|
||||
|
@ -489,14 +575,14 @@ class Weights:
|
|||
qzeros=qzeros,
|
||||
scales=scales,
|
||||
g_idx=g_idx,
|
||||
bits=bits,
|
||||
groupsize=groupsize,
|
||||
bits=gptq_params.bits,
|
||||
groupsize=gptq_params.groupsize,
|
||||
use_exllama=use_exllama,
|
||||
)
|
||||
elif quantize == "awq":
|
||||
from text_generation_server.layers.gptq import GPTQWeight
|
||||
|
||||
bits, groupsize, _, _ = self._get_gptq_params()
|
||||
gptq_params = self._get_gptq_params()
|
||||
|
||||
try:
|
||||
qweight = self.get_sharded(f"{prefix}.qweight", dim=0)
|
||||
|
@ -515,38 +601,74 @@ class Weights:
|
|||
qzeros=qzeros,
|
||||
scales=scales,
|
||||
g_idx=g_idx,
|
||||
bits=bits,
|
||||
groupsize=groupsize,
|
||||
bits=gptq_params.bits,
|
||||
groupsize=gptq_params.groupsize,
|
||||
use_exllama=use_exllama,
|
||||
)
|
||||
elif quantize == "marlin":
|
||||
from text_generation_server.layers.marlin import MarlinWeight
|
||||
from text_generation_server.layers.gptq import GPTQWeight
|
||||
from text_generation_server.layers.marlin import (
|
||||
MarlinWeight,
|
||||
repack_gptq_for_marlin,
|
||||
)
|
||||
|
||||
try:
|
||||
B = self.get_sharded(f"{prefix}.B", dim=0)
|
||||
except RuntimeError:
|
||||
raise RuntimeError(
|
||||
"Cannot load `marlin` weight, make sure the model is already quantized, or quantize it with `text-generation-server quantize ORIGINAL_MODEL_ID NEW_MODEL_ID`"
|
||||
quant_method = getattr(self, "quant_method", "marlin")
|
||||
if quant_method == "gptq":
|
||||
log_once(logger.info, "Converting GPTQ model to Marlin packing format.")
|
||||
gptq_params = self._get_gptq_params()
|
||||
|
||||
try:
|
||||
qweight = self.get_sharded(f"{prefix}.qweight", dim=0)
|
||||
except RuntimeError:
|
||||
raise RuntimeError(
|
||||
f"Cannot load `{quantize}` weight for GPTQ -> Marlin repacking, make sure the model is already quantized"
|
||||
)
|
||||
|
||||
g_idx = self.get_sharded(f"{prefix}.g_idx", dim=0)
|
||||
if gptq_params.desc_act or gptq_params.groupsize == -1:
|
||||
scales = self.get_tensor(f"{prefix}.scales")
|
||||
else:
|
||||
scales = self.get_sharded(f"{prefix}.scales", dim=0)
|
||||
|
||||
sharded_in_features = self.process_group.size() > 1
|
||||
|
||||
weight = repack_gptq_for_marlin(
|
||||
qweight=qweight,
|
||||
scales=scales,
|
||||
g_idx=g_idx,
|
||||
bits=gptq_params.bits,
|
||||
desc_act=gptq_params.desc_act,
|
||||
groupsize=gptq_params.groupsize,
|
||||
sym=gptq_params.sym,
|
||||
sharded_infeatures=sharded_in_features,
|
||||
)
|
||||
|
||||
num_groups = self._get_slice(f"{prefix}.s").get_shape()[0]
|
||||
if num_groups == 1:
|
||||
# The number of groups is 1 when group_size == -1. share
|
||||
# scales between all shards in this case.
|
||||
s = self.get_tensor(f"{prefix}.s")
|
||||
else:
|
||||
s = self.get_sharded(f"{prefix}.s", dim=0)
|
||||
weight = MarlinWeight(B=B, s=s)
|
||||
try:
|
||||
B = self.get_sharded(f"{prefix}.B", dim=0)
|
||||
except RuntimeError:
|
||||
raise RuntimeError(
|
||||
"Cannot load `marlin` weight, make sure the model is already quantized, or quantize it with `text-generation-server quantize ORIGINAL_MODEL_ID NEW_MODEL_ID`"
|
||||
)
|
||||
|
||||
num_groups = self._get_slice(f"{prefix}.s").get_shape()[0]
|
||||
if num_groups == 1:
|
||||
# The number of groups is 1 when groupsize == -1. share
|
||||
# scales between all shards in this case.
|
||||
s = self.get_tensor(f"{prefix}.s")
|
||||
else:
|
||||
s = self.get_sharded(f"{prefix}.s", dim=0)
|
||||
weight = MarlinWeight(B=B, s=s)
|
||||
|
||||
else:
|
||||
weight = self.get_sharded(f"{prefix}.weight", dim=1)
|
||||
return weight
|
||||
|
||||
def _get_gptq_params(self) -> Tuple[int, int, int, str]:
|
||||
def _get_gptq_params(self) -> _GPTQParams:
|
||||
try:
|
||||
bits = self.get_tensor("gptq_bits").item()
|
||||
groupsize = self.get_tensor("gptq_groupsize").item()
|
||||
desc_act = False
|
||||
sym = True
|
||||
quant_method = "gptq"
|
||||
except (SafetensorError, RuntimeError) as e:
|
||||
try:
|
||||
|
@ -554,10 +676,17 @@ class Weights:
|
|||
groupsize = self.gptq_groupsize
|
||||
desc_act = getattr(self, "gptq_desc_act", False)
|
||||
quant_method = getattr(self, "quant_method", "gptq")
|
||||
sym = getattr(self, "sym", True)
|
||||
except Exception:
|
||||
raise e
|
||||
|
||||
return bits, groupsize, desc_act, quant_method
|
||||
return _GPTQParams(
|
||||
bits=bits,
|
||||
desc_act=desc_act,
|
||||
groupsize=groupsize,
|
||||
quant_method=quant_method,
|
||||
sym=sym,
|
||||
)
|
||||
|
||||
def _set_gptq_params(self, model_id, revision):
|
||||
filename = "config.json"
|
||||
|
@ -574,6 +703,7 @@ class Weights:
|
|||
self.gptq_groupsize = data["quantization_config"]["group_size"]
|
||||
# Order is important here, desc_act is missing on some real models
|
||||
self.quant_method = data["quantization_config"]["quant_method"]
|
||||
self.gptq_sym = data["quantization_config"]["sym"]
|
||||
self.gptq_desc_act = data["quantization_config"]["desc_act"]
|
||||
except Exception:
|
||||
filename = "quantize_config.json"
|
||||
|
@ -588,6 +718,7 @@ class Weights:
|
|||
data = json.load(f)
|
||||
self.gptq_bits = data["bits"]
|
||||
self.gptq_groupsize = data["group_size"]
|
||||
self.gptq_sym = data["sym"]
|
||||
self.gptq_desc_act = data["desc_act"]
|
||||
if "version" in data and data["version"] == "GEMM":
|
||||
self.quant_method = "awq"
|
||||
|
|
Loading…
Reference in New Issue