![]() * (backend) use parking_lot crate for RwLock fairness
# Conflicts:
# backends/trtllm/src/backend.rs
* (launcher) default new server::run parameters to false for now
* (chore) fmt ... why?
* (ffi) use const for GetSamplingConfig
* (server) expose new SchedulingError
* (trt)
* (build) setup ccache if available
* (ffi) add max_new_tokens parameters
* (backend) cleanup a bit
* (backend) expose PullNewTokens
* (ffi) cleanup again
* (ffi) add missing headers imports
* (ffi) add template specialization to catch and convert to Rust Result<T, tensorrt_llm::common::TllmException>
* (looper) new looper initial implementation
* (ffi) remove narrowing type warning
* (ffi) encode the provided user prompt within each request thread
* (misc) change scope identifiers
* (backend) implement the post_processor background thread
* (misc) missing Result types for Rust
* use blocking_recv in looper to consume awaiting_requests at max before pulling in a single step
* (server) forward auth_token to server::run
* (build) fetchcontent use archives instead of git
* (ffi) fix usage of wrong vector constructor making a capacity fill call
* (ffi) missing namespace for tle::Response
* (ffi) do not use reference capture in lambda as we are not capturing anything
* (backend) refactor & cleanup
* (Dockerfile.trtllm) delete for now
* (misc) simplify [make_]move_iterator by using c++20 type inference
* (misc) no need to move for uint32_t items
* (scheduler) rework submit/pull logic
* (post) impl postprocessing
* (misc) delete backend.rs
* (misc) rerun-if-changed all the cmake modules
* (misc) move to latest trtllm
* (fix): HOPPER_SM_MAJOR is 9 not 8
* (misc: build for sm_{75,80,86,89,90} by default
* (misc): build with trtllm 0.13.0
* (misc): increase verbosity of spdlog
* (fix): do not recreate the stateful hashmap at every it
* (misc): update dependency in trtllm dockerfile
* (misc): update dependency in trtllm dockerfile
* (misc): disable logging in release mode
* (misc): improve trtllm download script robustness
* (fix): ore fixes for Dockerfile
* misc(cuda): require 12.6
* chore(cmake): use correct policy for download_timestamp
* feat(looper): check engine and executorWorker paths exist before creating the backend
* chore(cmake): download timestamp should be before URL
* feat(looper): minor optimizations to avoid growing too much the containers
* chore(trtllm): move dockerfile to right place
* chore(trtllm): disable tokenizer parallelism by default
* chore(trtllm): fmt
* chore(trtllm): post-rebase commit
* chore(trtllm): remove unused method
* feat(trtllm): cache maxNumTokens to avoid calling JSON everytime
* misc(router): remove SchedulingError
* feat(trtllm): do not tokenize twice
* Revert "chore(trtllm): remove unused method"
This reverts commit
|
||
---|---|---|
.devcontainer | ||
.github | ||
assets | ||
backends | ||
benchmark | ||
clients/python | ||
docs | ||
integration-tests | ||
launcher | ||
load_tests | ||
nix | ||
proto | ||
router | ||
server | ||
.dockerignore | ||
.gitignore | ||
.pre-commit-config.yaml | ||
.redocly.lint-ignore.yaml | ||
CODE_OF_CONDUCT.md | ||
CONTRIBUTING.md | ||
Cargo.lock | ||
Cargo.toml | ||
Dockerfile | ||
Dockerfile.nix | ||
Dockerfile_amd | ||
Dockerfile_intel | ||
Dockerfile_trtllm | ||
LICENSE | ||
Makefile | ||
README.md | ||
flake.lock | ||
flake.nix | ||
rust-toolchain.toml | ||
sagemaker-entrypoint.sh | ||
tgi-entrypoint.sh | ||
update_doc.py |
README.md
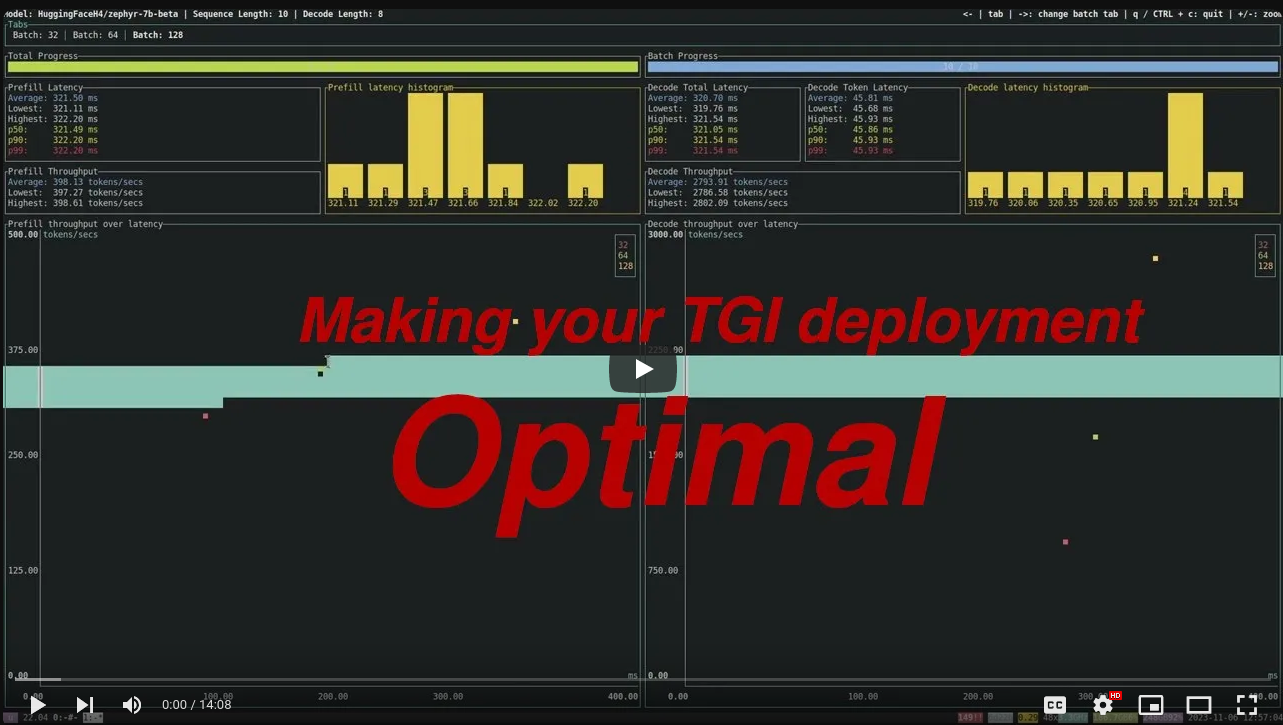
Text Generation Inference
A Rust, Python and gRPC server for text generation inference. Used in production at Hugging Face to power Hugging Chat, the Inference API and Inference Endpoint.
Table of contents
Text Generation Inference (TGI) is a toolkit for deploying and serving Large Language Models (LLMs). TGI enables high-performance text generation for the most popular open-source LLMs, including Llama, Falcon, StarCoder, BLOOM, GPT-NeoX, and more. TGI implements many features, such as:
- Simple launcher to serve most popular LLMs
- Production ready (distributed tracing with Open Telemetry, Prometheus metrics)
- Tensor Parallelism for faster inference on multiple GPUs
- Token streaming using Server-Sent Events (SSE)
- Continuous batching of incoming requests for increased total throughput
- Messages API compatible with Open AI Chat Completion API
- Optimized transformers code for inference using Flash Attention and Paged Attention on the most popular architectures
- Quantization with :
- Safetensors weight loading
- Watermarking with A Watermark for Large Language Models
- Logits warper (temperature scaling, top-p, top-k, repetition penalty, more details see transformers.LogitsProcessor)
- Stop sequences
- Log probabilities
- Speculation ~2x latency
- Guidance/JSON. Specify output format to speed up inference and make sure the output is valid according to some specs..
- Custom Prompt Generation: Easily generate text by providing custom prompts to guide the model's output
- Fine-tuning Support: Utilize fine-tuned models for specific tasks to achieve higher accuracy and performance
Hardware support
- Nvidia
- AMD (-rocm)
- Inferentia
- Intel GPU
- Gaudi
- Google TPU
Get Started
Docker
For a detailed starting guide, please see the Quick Tour. The easiest way of getting started is using the official Docker container:
model=HuggingFaceH4/zephyr-7b-beta
# share a volume with the Docker container to avoid downloading weights every run
volume=$PWD/data
docker run --gpus all --shm-size 1g -p 8080:80 -v $volume:/data \
ghcr.io/huggingface/text-generation-inference:2.3.1 --model-id $model
And then you can make requests like
curl 127.0.0.1:8080/generate_stream \
-X POST \
-d '{"inputs":"What is Deep Learning?","parameters":{"max_new_tokens":20}}' \
-H 'Content-Type: application/json'
You can also use TGI's Messages API to obtain Open AI Chat Completion API compatible responses.
curl localhost:8080/v1/chat/completions \
-X POST \
-d '{
"model": "tgi",
"messages": [
{
"role": "system",
"content": "You are a helpful assistant."
},
{
"role": "user",
"content": "What is deep learning?"
}
],
"stream": true,
"max_tokens": 20
}' \
-H 'Content-Type: application/json'
Note: To use NVIDIA GPUs, you need to install the NVIDIA Container Toolkit. We also recommend using NVIDIA drivers with CUDA version 12.2 or higher. For running the Docker container on a machine with no GPUs or CUDA support, it is enough to remove the --gpus all
flag and add --disable-custom-kernels
, please note CPU is not the intended platform for this project, so performance might be subpar.
Note: TGI supports AMD Instinct MI210 and MI250 GPUs. Details can be found in the Supported Hardware documentation. To use AMD GPUs, please use docker run --device /dev/kfd --device /dev/dri --shm-size 1g -p 8080:80 -v $volume:/data ghcr.io/huggingface/text-generation-inference:2.3.1-rocm --model-id $model
instead of the command above.
To see all options to serve your models (in the code or in the cli):
text-generation-launcher --help
API documentation
You can consult the OpenAPI documentation of the text-generation-inference
REST API using the /docs
route.
The Swagger UI is also available at: https://huggingface.github.io/text-generation-inference.
Using a private or gated model
You have the option to utilize the HF_TOKEN
environment variable for configuring the token employed by
text-generation-inference
. This allows you to gain access to protected resources.
For example, if you want to serve the gated Llama V2 model variants:
- Go to https://huggingface.co/settings/tokens
- Copy your cli READ token
- Export
HF_TOKEN=<your cli READ token>
or with Docker:
model=meta-llama/Meta-Llama-3.1-8B-Instruct
volume=$PWD/data # share a volume with the Docker container to avoid downloading weights every run
token=<your cli READ token>
docker run --gpus all --shm-size 1g -e HF_TOKEN=$token -p 8080:80 -v $volume:/data ghcr.io/huggingface/text-generation-inference:2.3.1 --model-id $model
A note on Shared Memory (shm)
NCCL
is a communication framework used by
PyTorch
to do distributed training/inference. text-generation-inference
make
use of NCCL
to enable Tensor Parallelism to dramatically speed up inference for large language models.
In order to share data between the different devices of a NCCL
group, NCCL
might fall back to using the host memory if
peer-to-peer using NVLink or PCI is not possible.
To allow the container to use 1G of Shared Memory and support SHM sharing, we add --shm-size 1g
on the above command.
If you are running text-generation-inference
inside Kubernetes
. You can also add Shared Memory to the container by
creating a volume with:
- name: shm
emptyDir:
medium: Memory
sizeLimit: 1Gi
and mounting it to /dev/shm
.
Finally, you can also disable SHM sharing by using the NCCL_SHM_DISABLE=1
environment variable. However, note that
this will impact performance.
Distributed Tracing
text-generation-inference
is instrumented with distributed tracing using OpenTelemetry. You can use this feature
by setting the address to an OTLP collector with the --otlp-endpoint
argument. The default service name can be
overridden with the --otlp-service-name
argument
Architecture
Detailed blogpost by Adyen on TGI inner workings: LLM inference at scale with TGI (Martin Iglesias Goyanes - Adyen, 2024)
Local install
You can also opt to install text-generation-inference
locally.
First install Rust and create a Python virtual environment with at least
Python 3.9, e.g. using conda
:
curl --proto '=https' --tlsv1.2 -sSf https://sh.rustup.rs | sh
conda create -n text-generation-inference python=3.11
conda activate text-generation-inference
You may also need to install Protoc.
On Linux:
PROTOC_ZIP=protoc-21.12-linux-x86_64.zip
curl -OL https://github.com/protocolbuffers/protobuf/releases/download/v21.12/$PROTOC_ZIP
sudo unzip -o $PROTOC_ZIP -d /usr/local bin/protoc
sudo unzip -o $PROTOC_ZIP -d /usr/local 'include/*'
rm -f $PROTOC_ZIP
On MacOS, using Homebrew:
brew install protobuf
Then run:
BUILD_EXTENSIONS=True make install # Install repository and HF/transformer fork with CUDA kernels
text-generation-launcher --model-id mistralai/Mistral-7B-Instruct-v0.2
Note: on some machines, you may also need the OpenSSL libraries and gcc. On Linux machines, run:
sudo apt-get install libssl-dev gcc -y
Optimized architectures
TGI works out of the box to serve optimized models for all modern models. They can be found in this list.
Other architectures are supported on a best-effort basis using:
AutoModelForCausalLM.from_pretrained(<model>, device_map="auto")
or
AutoModelForSeq2SeqLM.from_pretrained(<model>, device_map="auto")
Run locally
Run
text-generation-launcher --model-id mistralai/Mistral-7B-Instruct-v0.2
Quantization
You can also run pre-quantized weights (AWQ, GPTQ, Marlin) or on-the-fly quantize weights with bitsandbytes, EETQ, fp8, to reduce the VRAM requirement:
text-generation-launcher --model-id mistralai/Mistral-7B-Instruct-v0.2 --quantize
4bit quantization is available using the NF4 and FP4 data types from bitsandbytes. It can be enabled by providing --quantize bitsandbytes-nf4
or --quantize bitsandbytes-fp4
as a command line argument to text-generation-launcher
.
Read more about quantization in the Quantization documentation.
Develop
make server-dev
make router-dev
Testing
# python
make python-server-tests
make python-client-tests
# or both server and client tests
make python-tests
# rust cargo tests
make rust-tests
# integration tests
make integration-tests